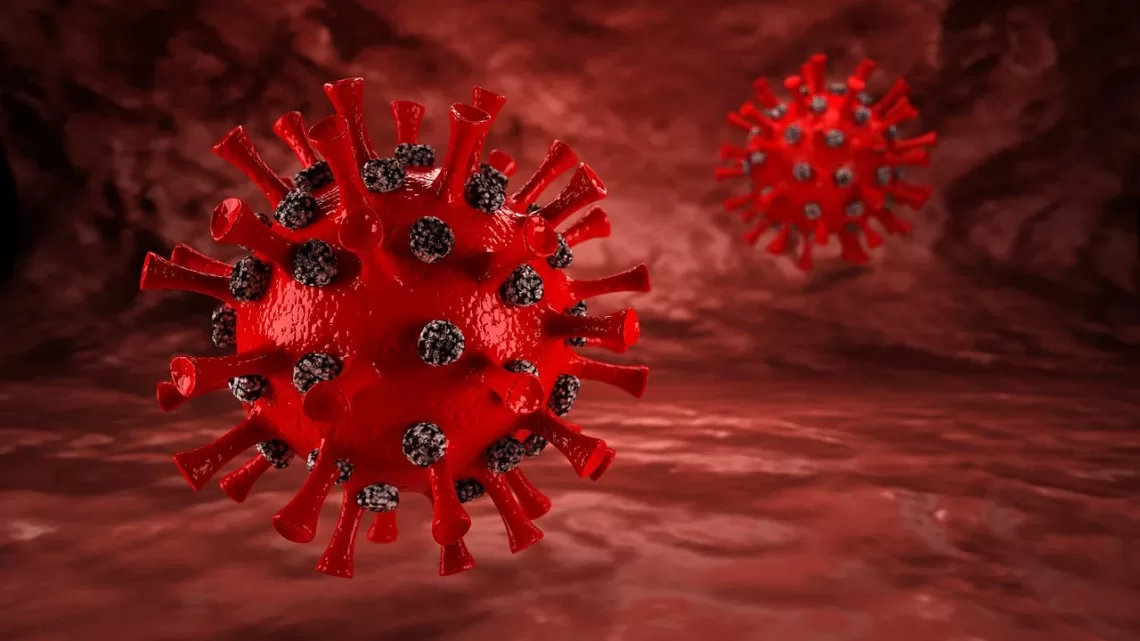
Early COVID-19 symptoms vary by age group, according to new research.
July 31, 2021New research has discovered that the symptoms of early COVID-19 infection vary by age group and by men and women. These distinctions are especially noticeable between younger age groups (16-59 years) and older age groups (60->80 years), and men exhibit distinct symptoms from women during the early stages of COVID-19 infection.
The study, headed by researchers from King’s College London and published today in the Lancet Digital Health, analyses data from the ZOE COVID Symptom Study app from April 20th to October 15th, 2020. Through a cooperative initiative with the Department of Health and Social Care, app contributors are encouraged to get checked as soon as they report any new symptoms. When three days of self-reported symptoms were used to mimic the early indicators of COVID-19 infection, the researchers correctly recognised 80% of cases.
Researchers examined the ability of current National Health Service UK diagnostic criteria with a Hierarchical Gaussian Process model, a type of machine learning, to predict early indications of COVID-19 infection.
This machine learning model was able to incorporate some of the individual’s characteristics, such as age, sex, and health status, and shown that early COVID-19 infection symptoms vary among different groups.
They used prospective, observational, longitudinal self-reported data from participants in the United Kingdom on 19 symptoms over the course of three days following symptom onset, as well as COVID-19 PCR test results extracted from the COVID-19 Symptom Study mobile phone app, in this large-scale, prospective, epidemiological surveillance study. They divided the study population into a training set (those who reported symptoms between April 29, 2020, and October 15, 2020) and a test set (those who reported symptoms between Oct 16, 2020, and Nov 30, 2020), and analysed self-reported symptoms using three models: the National Health Service (NHS) algorithm in the United Kingdom, logistic regression, and the hierarchical Gaussian process model researchers developed (eg, specific COVID-19 symptoms, comorbidities, and clinical information). Sensitivity, specificity, and area under the receiver operating characteristic curve (AUC) of the model used to predict COVID-19 positive in the test set were all compared. They also assessed the importance of symptoms in the early detection of COVID-19 in subgroups of the population stratified by occupation, sex, age, and body mass index using the hierarchical Gaussian process model.
18 symptoms were evaluated, each of which was associated with a varied level of importance for early detection in different groups. The most common symptoms associated with early identification of COVID-19 were loss of smell, chest pain, persistent cough, abdominal pain, blisters on the feet, eye soreness, and peculiar muscular pain. However, the loss of scent was no longer significant in adults over the age of 60 and was irrelevant in subjects over the age of 80. In older age groups (60-79 and >80), other early symptoms such as diarrhoea were critical. While fever is a well-known indication of sickness, it was not an early component of any age group.
Men were more likely to express shortness of breath, exhaustion, chills, and shivering, while women reported a loss of scent, chest pain, and a persistent cough.
While these models were developed within the COVID Symptom study app, they were reproduced across time, indicating that they would apply to non-app contributors as well. While the models were developed for the virus’s initial strain and Alpha variants, the major findings indicate that the symptoms of the Delta variant and subsequent variants will also vary amongst demographic groups.
Claire Steves, Reader at King’s College London and lead author, stated: “It is critical for individuals to understand that the initial symptoms are diverse and may look different for each member of a family or household.” Testing guidelines might be modified to enable cases to be identified sooner, particularly in the face of novel highly transmissible variations. This may entail administering readily available lateral flow tests to individuals who exhibit any of these non-core symptoms.”
Dr Liane dos Santos Canas of King’s College London, the study’s primary author, explained: “Currently, in the United Kingdom, just a few indicators are used to encourage self-isolation and additional testing. Using a wider number of symptoms and only after a few days of illness, we can improve the detection of COVID-19 positive cases using AI. We hope that such an approach is used to encourage more individuals to be tested as soon as feasible in order to reduce the chance of infection spreading.”
Dr Marc Modat, Senior Lecturer at King’s College London, explained: “As part of our investigation, we discovered that the profile of symptoms caused by COVID-19 varies by group. This suggests that the criterion for encouraging people to get tested should be tailored to their specific circumstances, such as age. Alternatively, a broader collection of symptoms could be evaluated, taking into consideration the disease’s diverse manifestations among different groups.”
Reference
Canas, L. S., Sudre, C. H., Capdevila Pujol, J., Polidori, L., Murray, B., Molteni, E., Graham, M. S., Klaser, K., Antonelli, M., Berry, S., Davies, R., Nguyen, L. H., Drew, D. A., Wolf, J., Chan, A. T., Spector, T., Steves, C. J., Ourselin, S., & Modat, M. (2021). Early detection of COVID-19 in the UK using self-reported symptoms: a large-scale, prospective, epidemiological surveillance study. The Lancet Digital Health. Published. https://doi.org/10.1016/s2589-7500(21)00131-x