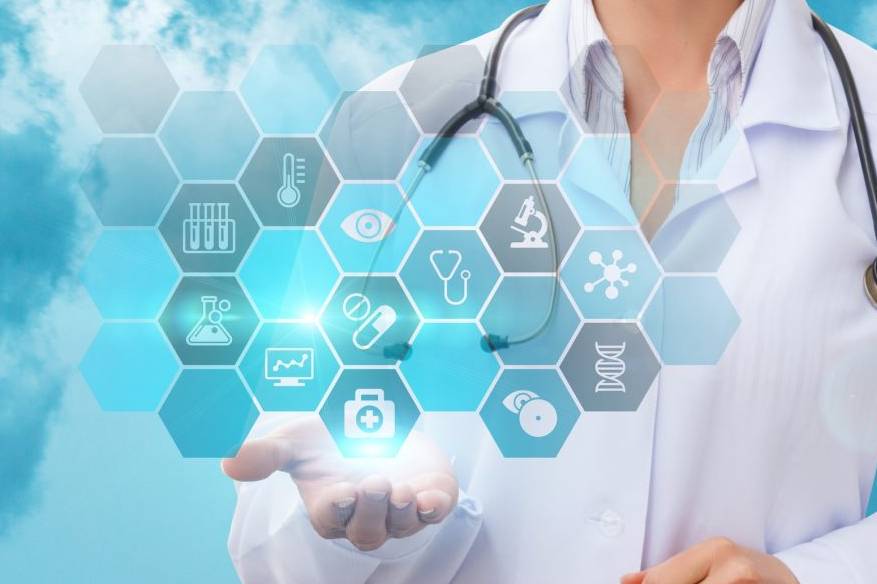
AI-Driven Revolution in Clinical Document Parsing: Improving Heart Failure Diagnosis
December 26, 2023The healthcare landscape is on the verge of a major transformation, thanks to the impact of generative AI, particularly in the realm of clinical document parsing.
A recent breakthrough in using AI for analyzing echocardiogram reports has showcased the immense potential of AI technologies in reshaping how medical data is interpreted and, consequently, how patient care is delivered.
Modern healthcare faces a significant challenge when it comes to parsing clinical documents, particularly complex reports like echocardiograms, crucial for diagnosing heart conditions. These reports contain vital information, such as ejection fraction (EF) values, essential for diagnosing heart failure. Efficiently and accurately parsing these reports is crucial, but the presence of medical jargon, abbreviations, patient-specific data, and unstructured free-text narratives, along with charts and tables, makes consistent interpretation a difficult task. This not only adds pressure on time-constrained clinicians but also increases the risk of errors in patient care and record-keeping.
Enter Generative AI – a game-changer in the field of clinical document parsing. It has the potential to automate the extraction and structuring of intricate medical data from unstructured documents, significantly improving accuracy and efficiency. For instance, recent research introduces an AI-powered system that uses a pre-trained transformer model fine-tuned with a custom dataset of annotated echocardiogram reports. This system demonstrates remarkable efficiency in extracting EF values, a critical marker in heart failure diagnosis.
What makes this technology even more promising is its adaptability. It learns specific medical terminologies over time, ensuring continual improvement and customization. This not only saves clinicians valuable time but also allows them to shift their focus from administrative tasks to providing better patient care.
The recent advancements in Generative AI owe much to the revolutionary ‘transformers’ model architecture. Unlike previous models that processed text sequentially, transformers can analyze entire text blocks simultaneously, leading to a deeper and more nuanced understanding of language.
Pre-trained transformers, equipped with a broad understanding of general language patterns and structures, serve as a solid foundation for systems utilizing this technology. However, fine-tuning is crucial to adapting them for specialized tasks and industry-specific requirements. This process involves further training on specific datasets, enabling the model to understand the unique linguistic characteristics, terminologies, and text structures of a particular domain.
For instance, a pre-trained transformer may lack the nuances of language specific to echocardiogram reports. Fine-tuning it with a targeted dataset allows the model to accurately extract and interpret information crucial in cardiology. The result is a model that aids healthcare professionals in making more informed decisions, improving patient care, and potentially saving lives.
The impact of fine-tuning on a custom dataset is evident in the research results, showcasing a 90% reduction in sensitivity to different prompts. This reduction, measured by standard deviation in evaluation metrics, highlights the efficiency gained through fine-tuning, making the model more adept at handling specialized tasks.
AI-driven clinical document parsing goes beyond efficiency gains – it significantly streamlines clinical workflows. By automating the extraction and analysis of vital data from medical documents, it reduces the need for manual data entry, improving accuracy and allowing clinicians to focus more on patient care. The technology’s ability to understand complex medical terms and extract relevant information leads to better patient outcomes by enabling faster and more comprehensive analyses of patient histories and conditions. In practice, this transformative AI technology has saved over 1,500 hours annually, enhancing healthcare delivery efficiency.
While AI streamlines information management, the human touch remains indispensable in delivering excellent patient care. The ‘clinician-in-the-loop’ concept ensures a balance between AI’s technological efficiency and the expertise of healthcare professionals. The clinician’s involvement guarantees the accuracy and ethical application of the technology, reinforcing patient safety and clinician trust in the system.
As we embrace AI in healthcare, its integration into clinical settings is poised to become more widespread. This study not only underscores the transformative potential of AI but also provides a glimpse into a future where technology and medicine converge for the greater benefit of society. For those interested in delving deeper, the complete research can be accessed on arXiv.
Reference:
Fine-tuning pre-trained extractive QA models for clinical document parsing – arXiv:2312.02314