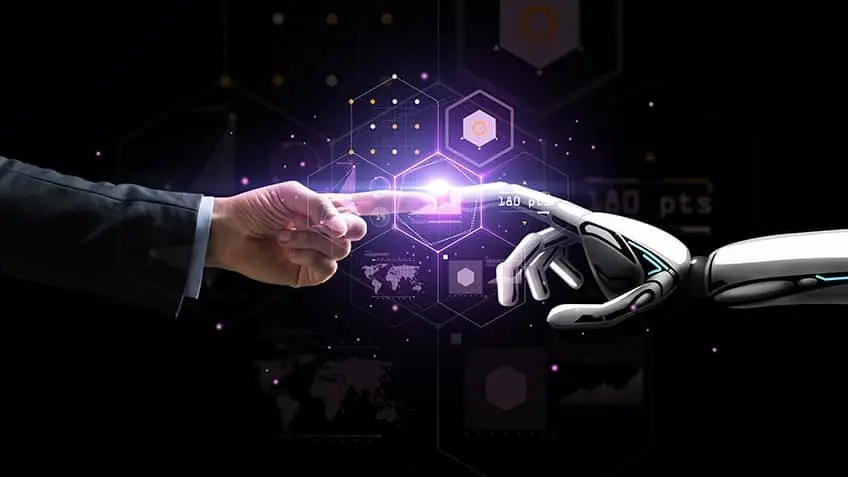
AI System Predicts Consequences of Gene Modifications
January 31, 2024Researchers Harness Artificial Intelligence to Decode the Symphony of Human Genes
In a groundbreaking collaboration, scientists from Gladstone Institutes, the Broad Institute of MIT and Harvard, and Dana-Farber Cancer Institute have tapped into the realm of artificial intelligence (AI) to unravel the complexities of gene networks governing cellular functions. The researchers, led by Gladstone Assistant Investigator Dr. Christina Theodoris, introduced a cutting-edge AI model named Geneformer, showcased in a recent publication in the journal Nature.
Geneformer, a large language model, delves into the intricate web of gene interactions, seeking to understand how disruptions in these networks contribute to diseases. The AI system, akin to the widely recognized ChatGPT, employs transfer learning, a method where fundamental knowledge is gleaned from massive datasets and applied to new tasks, propelling the model into the forefront of AI advancements.
Dr. Theodoris emphasizes the broad applications of Geneformer across various biological domains, envisioning its role in identifying potential drug targets for diseases. The model’s unique approach holds promise for designing network-correcting therapies, particularly in diseases where limited data has hindered progress.
Decoding the Complexity of Gene Networks
The challenge lies in comprehending the intricate dance of thousands of interconnected genes, a task likened to untangling a spiderweb. Gene networks, when disturbed, can lead to cascades of molecular activity influencing cellular functions and contributing to diseases. Traditional mapping methods often rely on extensive datasets specific to particular diseases, limiting their applicability.
Geneformer revolutionizes this approach through transfer learning, where it is first pretrained on a vast dataset encompassing gene interactions across diverse human tissues. Fine-tuning Geneformer allows it to make predictions about gene connections and potential disease outcomes with remarkable accuracy.
Transfer Learning: A Leap Beyond Conventional Models
What sets Geneformer apart is its ability to make accurate predictions even with minimal examples of relevant data. Unlike traditional machine learning models, which require retraining from scratch for each new task, Geneformer’s core knowledge can be seamlessly transferred to address diverse biological questions. This feature becomes crucial in diseases where limited patient data impedes progress, such as rare diseases or those affecting challenging-to-sample tissues.
Heart Disease Insights: A Testimonial to Geneformer’s Capabilities
Theodoris and her team put Geneformer to the test in unraveling the mysteries of heart disease. Predicting genes with detrimental effects on cardiomyocytes, the muscle cells in the heart, Geneformer identified both known and previously undiscovered genes associated with heart disease.
The discovery of TEAD4, a gene not previously linked to heart disease, showcased Geneformer’s ability to make novel predictions. Experimental validation confirmed TEAD4’s crucial role in cardiomyocyte function, underscoring the model’s capacity to uncover new insights.
Targeted Therapies Unveiled: A Glimpse into the Future
Taking a step further, Geneformer predicted genes that, when targeted, could transform diseased cardiomyocytes into a healthy state at a gene network level. In the laboratory, removing these predicted genes using CRISPR gene editing technology successfully restored the beating ability of diseased cardiomyocytes.
Dr. Theodoris emphasizes the transfer learning approach as a breakthrough in overcoming data limitations. The model’s ability to identify potential proteins for drug targeting in diseased cells holds promise for more efficient drug development.
Open-Source Innovation and Future Endeavors
The researchers plan to expand Geneformer’s capabilities by analyzing a broader range of cell types. The open-source nature of the model invites collaboration and exploration by other scientists, heralding a new era in AI-driven genomics.
Dr. Theodoris concludes, “The exciting thing about our approach is that Geneformer’s fundamental knowledge about gene networks can now be transferred to answer many biological questions, and we’re looking forward to seeing what other people do with it.” The unveiling of Geneformer marks a significant leap toward deciphering the intricate language of gene networks and opens doors to innovative solutions in the realm of precision medicine.
Reference
Theodoris, C. V., Xiao, L., Chopra, A., Chaffin, M. D., Al Sayed, Z. R., Hill, M. C., Mantineo, H., Brydon, E. M., Zeng, Z., Liu, X. S., & Ellinor, P. T. (2023). Transfer learning enables predictions in network biology. Nature, 618(6), 616–624.