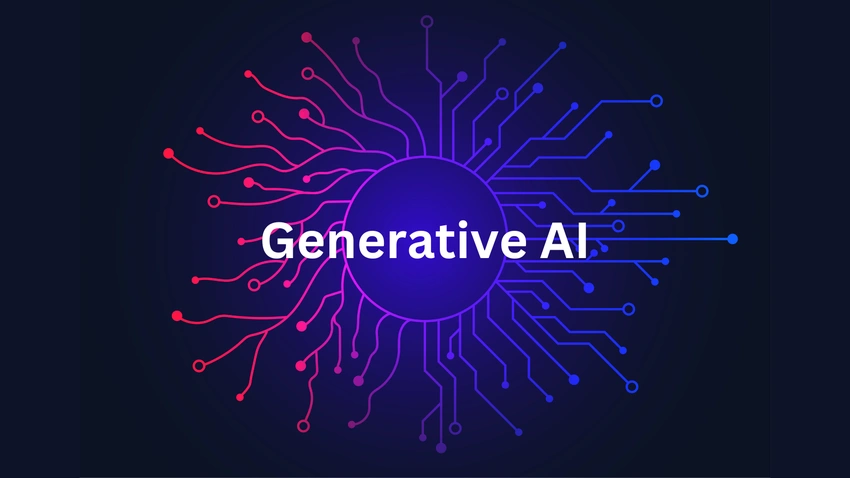
Generative AI for drug discovery
December 27, 2023Table of Contents
I. Introduction:
A. Brief Overview of Traditional Drug Discovery Process:
In the traditional drug discovery process, the development of new drugs typically involves several sequential steps, each with its own challenges and time-consuming aspects. The process generally includes the following key stages:
- Target Identification and Validation: Scientists identify specific biological targets, such as proteins or nucleic acids, that are associated with a particular disease or condition. These targets are then validated to ensure their relevance.
- High-Throughput Screening: Compounds, often from large chemical libraries, are tested to identify those that interact with the chosen target. This step involves screening thousands to millions of compounds.
- Hit to Lead Optimization: Promising compounds, or “hits,” are optimized to enhance their properties, such as efficacy, safety, and bioavailability. This iterative process involves chemical modifications to improve the compound’s characteristics.
- Preclinical Testing: Optimized compounds undergo rigorous testing in laboratory settings and animal models to assess safety, efficacy, and potential toxicities before advancing to human trials.
- Clinical Trials: Compounds that pass preclinical testing enter human clinical trials, which consist of three phases. Phase I focuses on safety, Phase II on efficacy and side effects, and Phase III involves large-scale testing to confirm results.
- FDA Approval: Successful completion of clinical trials leads to regulatory submission, review, and potential approval by regulatory agencies like the U.S. Food and Drug Administration (FDA).
B. Current Challenges and Limitations in Drug Discovery:
While the traditional drug discovery process has led to many successful drugs, it is associated with several challenges and limitations:
- Time-Consuming: The process can take 10-15 years or more, making drug development a lengthy and resource-intensive endeavor.
- High Costs: The costs of developing a new drug are exorbitant, with a significant portion allocated to failed candidates during the clinical trial phases.
- Low Success Rates: Many potential drug candidates fail during clinical trials due to safety concerns, lack of efficacy, or unforeseen side effects.
- Limited Target Space: Identifying novel and viable drug targets is becoming increasingly challenging as known targets are extensively explored.
C. Potential of Generative AI to Revolutionize Drug Discovery:
Generative artificial intelligence (AI) holds the promise to transform drug discovery by addressing some of the existing challenges:
- Accelerated Target Identification: Generative AI models can analyze large datasets to identify potential drug targets more efficiently, allowing researchers to explore a broader range of possibilities.
- Enhanced Compound Screening: AI algorithms can predict how well a compound may interact with a target, reducing the number of compounds that need to be experimentally tested in high-throughput screening.
- De Novo Drug Design: Generative AI can propose novel molecular structures that may exhibit desirable properties for drug development. This capability is particularly valuable in hit-to-lead optimization.
- Personalized Medicine: AI can analyze diverse patient data to identify biomarkers and develop tailored therapies for specific patient populations, advancing the concept of personalized medicine.
- Data Integration: Generative AI can leverage vast amounts of omics data (genomics, transcriptomics, proteomics, etc.) to provide a more comprehensive understanding of diseases and potential drug targets.
In summary, the introduction outlines the traditional drug discovery process, highlights current challenges and limitations, and introduces the potential of generative AI to revolutionize drug discovery by addressing these challenges and expediting the development of novel and effective therapeutics.
II. How Generative AI Works:
A. Explanation of Machine Learning Algorithms Used in Generative AI:
Generative AI relies on sophisticated machine learning algorithms, particularly within the domain of deep learning, to create new and valuable outputs, such as novel drug molecules. Key machine learning algorithms used in generative AI for drug discovery include:
- Generative Adversarial Networks (GANs): GANs consist of two neural networks, a generator, and a discriminator, which are trained simultaneously. The generator creates new data (in this case, potential drug molecules), and the discriminator evaluates them. This iterative process refines the generator’s ability to create increasingly realistic and valuable outputs.
- Recurrent Neural Networks (RNNs) and Long Short-Term Memory Networks (LSTMs): RNNs and LSTMs are types of neural networks suitable for processing sequential data. In drug discovery, they can be used to generate molecular structures by predicting the next element in a sequence based on the preceding elements.
- Variational Autoencoders (VAEs): VAEs are generative models that learn the underlying structure of the input data and can generate new samples from this learned distribution. In drug discovery, VAEs can be used to generate novel molecular structures with desired properties.
- Deep Reinforcement Learning: Reinforcement learning involves training models to make sequences of decisions by rewarding or punishing them based on the outcomes of their actions. In drug discovery, this can be applied to optimize molecular structures for desired properties.
B. Overview of How Generative AI Is Used to Design New Drug Molecules:
- Data Input:
- Generative AI starts by training on vast datasets containing information about existing drug molecules, their structures, and associated properties. This data often includes information from various omics sources, such as genomics, transcriptomics, and proteomics.
- Training the Model:
- The chosen machine learning algorithm is trained on the dataset, learning the patterns, relationships, and features of known drug molecules. The model becomes capable of generating new molecules that share characteristics with those in the training data.
- Generating Molecules:
- Once trained, the generative AI model can create entirely new molecular structures or suggest modifications to existing molecules. These generated structures aim to possess specific properties desirable for drug development, such as improved efficacy, reduced toxicity, or enhanced bioavailability.
- Optimization and Iteration:
- The generated molecules undergo optimization processes where their properties are refined. This may involve filtering for drug-likeness, predicting biological activity, and ensuring adherence to safety and regulatory standards. The model is iteratively improved based on the feedback from these optimization steps.
- Experimental Validation:
- The most promising candidates generated by the AI model are subjected to experimental validation in laboratory settings. This involves synthesizing and testing the physical properties and biological activities of the proposed molecules to verify their viability as potential drugs.
In summary, generative AI employs advanced machine learning algorithms to learn from existing data and generate novel drug molecules with desired properties. The process involves training the model, generating molecules, optimizing their properties, and validating their potential through experimental methods in the drug discovery pipeline.
III. Benefits of Generative AI in Drug Discovery:
A. Improved Efficiency and Speed of Drug Discovery Process:
- Accelerated Target Identification:
- Generative AI can quickly analyze vast datasets to identify potential drug targets, expediting the initial stages of drug discovery.
- High-Throughput Screening Enhancement:
- By predicting which compounds are likely to interact with a target, generative AI reduces the number of compounds that need to be experimentally screened, saving time and resources.
- Hit-to-Lead Optimization Streamlining:
- AI models can propose optimized compounds, accelerating the hit-to-lead optimization phase by suggesting modifications that enhance desired properties.
- Predictive Analysis for Clinical Trials:
- Generative AI can predict the potential success or failure of a drug candidate in clinical trials, allowing researchers to prioritize the most promising candidates and reduce the likelihood of failures.
B. Ability to Identify Novel Drug Candidates:
- Exploration of Chemical Space:
- Generative AI can explore vast chemical spaces and propose novel molecular structures that may not have been considered using traditional methods.
- Addressing Undruggable Targets:
- AI enables the identification of drug candidates for targets that were previously considered challenging or “undruggable” using conventional approaches.
- Diversity in Compound Libraries:
- AI-generated compounds contribute to the diversity of compound libraries, offering researchers a broader range of potential drug candidates to explore.
C. Potential to Design Drugs with Better Potency and Fewer Side Effects:
- Optimized Molecular Structures:
- Generative AI can suggest molecular structures that are optimized for desired properties, such as enhanced potency and reduced toxicity.
- Tailored Drug Design:
- AI models can take into account specific patient characteristics, leading to the development of personalized drugs with improved efficacy and fewer side effects.
- Predictive Toxicology:
- AI can predict potential toxicities early in the drug development process, allowing for the exclusion of high-risk candidates and reducing the likelihood of adverse effects.
- Fine-Tuning Pharmacokinetics:
- Generative AI can assist in designing drugs with optimal pharmacokinetic profiles, ensuring efficient absorption, distribution, metabolism, and excretion.
In summary, the integration of generative AI in drug discovery brings several benefits, including increased efficiency and speed, the identification of novel drug candidates, and the potential to design drugs with enhanced potency and fewer side effects. These advantages contribute to a more streamlined and effective drug discovery process.
IV. Examples of Generative AI in Drug Discovery:
A. Examples of Successful Applications of Generative AI:
- Atomwise:
- Atomwise utilizes AI for virtual drug screening. It has successfully identified potential drug candidates for diseases such as Ebola and multiple sclerosis. The technology analyzes the interaction between small molecules and target proteins, predicting the binding affinity to identify promising compounds.
- Insilico Medicine:
- Insilico Medicine employs generative AI for drug discovery, particularly in the identification of novel biomarkers and potential drug candidates for aging-related diseases. The company’s AI-driven platforms analyze molecular and patient data to accelerate drug development.
- Recursion Pharmaceuticals:
- Recursion Pharmaceuticals uses generative AI for image-based drug discovery. The company combines AI with high-throughput screening and cellular imaging to identify novel drug candidates for a range of diseases, including genetic disorders and cancers.
- BenevolentAI:
- BenevolentAI uses AI to analyze biomedical data and propose novel drug candidates. The platform integrates information from various sources to generate hypotheses and identify potential targets. It has been applied in drug discovery for conditions such as Parkinson’s disease.
- DeepMind’s AlphaFold:
- While originally developed for protein folding predictions, DeepMind’s AlphaFold has implications for drug discovery. By accurately predicting protein structures, it can aid in understanding biological mechanisms and identifying potential drug targets.
B. Emerging Trends and Future Prospects of Generative AI in Drug Discovery:
- Personalized Medicine Advancements:
- Generative AI is expected to play a crucial role in tailoring drug treatments to individual patients based on their unique genetic and molecular profiles. This personalized approach could enhance treatment efficacy and minimize side effects.
- Integration with Experimental Biology:
- Future trends include closer integration of generative AI with experimental biology, combining computational predictions with real-world laboratory data to validate and refine drug candidates more efficiently.
- AI-Driven Polypharmacology:
- Polypharmacology, the study of how drugs interact with multiple targets, is an emerging trend. Generative AI can aid in designing drugs that simultaneously target multiple pathways, potentially leading to more effective treatments.
- Increased Collaboration and Data Sharing:
- Collaborative efforts and data sharing among pharmaceutical companies, research institutions, and AI developers are expected to increase. This collaborative approach can leverage diverse datasets for more comprehensive generative AI models.
- Focus on Rare Diseases:
- Generative AI may be applied more extensively to rare diseases, where traditional drug discovery efforts face significant challenges. AI-driven platforms can efficiently explore potential treatment options for these less common conditions.
In summary, generative AI has shown success in various drug discovery applications, and ongoing trends suggest a growing focus on personalized medicine, increased collaboration, and the exploration of rare diseases. The continued evolution of generative AI holds great promise for revolutionizing drug discovery processes in the future.
V. Conclusion:
A. Summary of Key Points:
- Traditional Drug Discovery Challenges:
- The traditional drug discovery process is time-consuming, costly, and often faces challenges such as low success rates and limited target options.
- Generative AI Overview:
- Generative AI employs advanced machine learning algorithms, including GANs, RNNs, and VAEs, to accelerate the drug discovery process by predicting, designing, and optimizing novel drug candidates.
- Benefits of Generative AI:
- The use of generative AI in drug discovery offers improved efficiency, accelerated target identification, the ability to identify novel drug candidates, and the potential to design drugs with enhanced potency and fewer side effects.
- Successful Applications:
- Examples of successful applications of generative AI in drug discovery include companies like Atomwise, Insilico Medicine, Recursion Pharmaceuticals, and BenevolentAI, each contributing to the identification of potential therapeutics for various diseases.
- Emerging Trends:
- Emerging trends in generative AI for drug discovery include personalized medicine advancements, integration with experimental biology, AI-driven polypharmacology, increased collaboration and data sharing, and a focus on rare diseases.
B. Potential Impact of Generative AI on the Future of Drug Discovery:
- Revolutionizing Drug Discovery:
- Generative AI has the potential to revolutionize drug discovery by significantly reducing the time and resources required, accelerating the identification of novel drug candidates, and improving the overall success rates of drug development.
- Enhanced Target Exploration:
- The technology enables the exploration of a broader chemical and biological space, leading to the identification of targets and compounds that may have been overlooked by traditional methods.
- Personalized Medicine and Targeted Therapies:
- Generative AI facilitates the development of personalized medicines and targeted therapies by considering individual patient characteristics, contributing to more effective and tailored treatment options.
- Addressing Unmet Medical Needs:
- The application of generative AI to drug discovery can address unmet medical needs, particularly in the realm of rare diseases where traditional approaches face significant challenges.
C. Importance of Continued Research and Investment in this Area:
- Innovation and Breakthroughs:
- Continued research and investment in generative AI for drug discovery are crucial for fostering innovation and breakthroughs in the field. Advancements in algorithms, data integration, and experimental validation methods can further enhance the capabilities of generative AI.
- Optimizing Drug Development:
- Ongoing efforts in research and investment can optimize the drug development process, leading to the identification of safer, more efficacious drugs and improving the overall success rates of clinical trials.
- Addressing Complex Diseases:
- Continued investment is essential for tackling complex diseases and expanding the applications of generative AI to areas where traditional methods may fall short.
- Collaboration Across Sectors:
- Collaboration between academia, industry, and technology developers is crucial for advancing generative AI in drug discovery. Shared knowledge, data, and resources can accelerate progress and ensure a collective effort toward transformative outcomes.
In conclusion, the application of generative AI in drug discovery holds immense promise for revolutionizing the field. Continued research, innovation, and investment are vital to realizing the full potential of generative AI in addressing current challenges and shaping the future of drug discovery. This transformative technology has the capacity to usher in a new era of more efficient, targeted, and personalized therapeutic interventions.