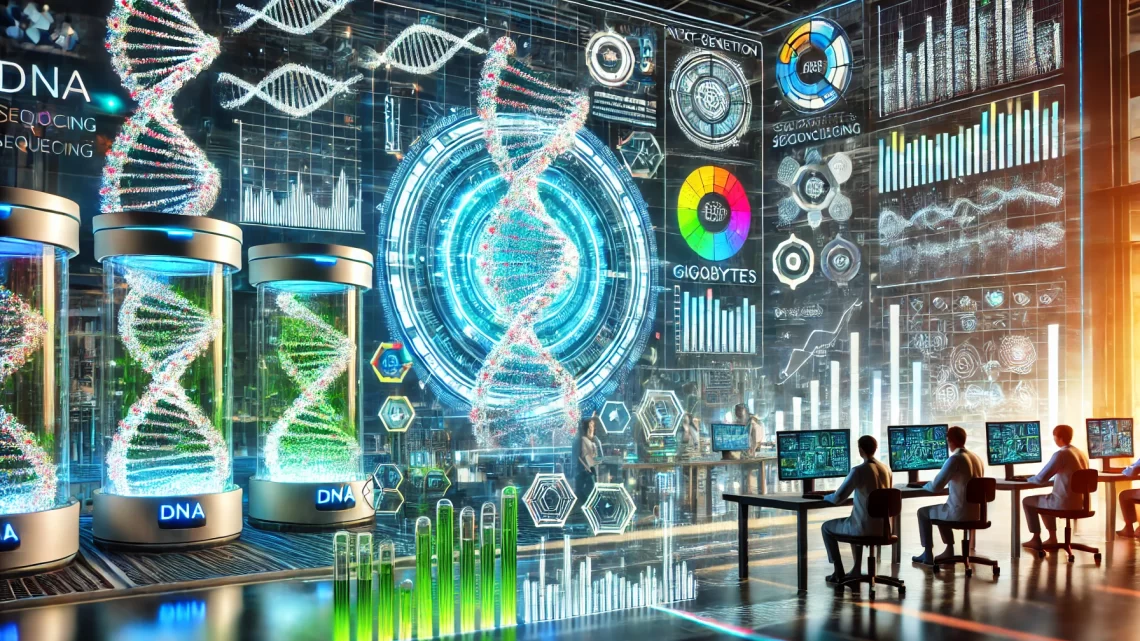
Generative AI in De Novo Drug Design
December 18, 2024The Rise of Generative AI in Drug Design: Shaping the Future of Molecule and Protein Generation
The rapid development of generative artificial intelligence (AI) has revolutionized various industries, and one of the most promising areas of transformation is drug discovery. Traditional drug design has often been a costly and time-consuming process, constrained by the limitations of chemical libraries and available biological data. However, the advent of generative AI in drug design, particularly in de novo molecule and protein generation, is ushering in an exciting new era of innovation, efficiency, and potential breakthroughs.
This blog post delves into the application of generative AI models in drug design, examining the core methods, advancements, challenges, and future directions in this rapidly evolving field.
What is De Novo Drug Design?
De novo drug design is a novel approach in drug development that allows scientists to create entirely new compounds from scratch, bypassing the limitations of traditional drug discovery that relies on optimizing known molecules or screening chemical libraries. Unlike conventional drug design methods, which often focus on modifying existing compounds, de novo drug design involves exploring uncharted chemical spaces to generate new biological entities with unique properties. This approach is particularly exciting because it holds the potential to uncover drug candidates that are more effective, safer, and cost-efficient, offering a promising solution to the long-standing challenges in drug development.
The core idea behind de novo drug design is the use of AI models to generate molecules and proteins that have never been seen before. By leveraging statistical methods and machine learning (ML) techniques, these models can explore the vast space of possible compounds, finding novel molecular structures that can be fine-tuned to fulfill specific therapeutic needs.
Table summarizing the timeline of main events in generative AI for drug design, following the order of development and improvements in methods:
Stage | Event | Details |
---|---|---|
Early Days | Traditional Drug Discovery Methods | Computationally expensive methods; establishment of drug-likeness concepts using QSAR and virtual screening (VS) for optimizing drug discovery. |
Pre-Generative AI | Machine Learning (ML) in Drug Design | ML-driven QSAR and ML-assisted directed evolution for protein engineering; optimization of existing compounds and biological entities using chemical libraries. |
Emergence of De Novo Drug Design | De Novo Drug Design Concept | Introduction of generating new molecules and proteins from scratch, instead of optimizing existing compounds. |
Early 1D Molecular Generation | Initial Generative Models Using SMILES Strings | Use of Variational Autoencoders (VAEs) like CVAE, GVAE, SD-VAE for 1D representations; limited molecular similarity representation in SMILES strings. |
Shift to 2D Graph Generation | 2D Graph Representation of Molecular Structures | Introduction of Junction Tree VAE (JTVAE) for generating 2D molecular graph structures, improving performance in molecular generation. |
Introduction of Diffusion Models | Diffusion Models for Molecular Generation | Introduction of Equivariant Diffusion Models (EDM) for direct generation in atomic feature space, bypassing the need for atomic ordering in molecular generation. |
Improved Diffusion-Based Models | Advanced Diffusion Models | Improvements in scalability and diversity; GCDM, MDM, GeoLDM, and MiDi address challenges like graph edge distinctions, low-dimensional latent space mapping, and improved performance on complex datasets like GEOM-Drugs. |
Target-Aware Molecular Design | Target-Aware Design Methods | Emergence of target-aware designs focusing on generating molecules based on specific biological targets, contrasting with target-agnostic approaches. LBDD and SBDD approaches gain traction. |
SBDD Gains Prominence | Structure-Based Drug Design (SBDD) | Increased use of protein 3D structures in molecule generation; diffusion models used in methods like LiGAN, Pocket2Mol, TargetDiff, and DiffSBDD for binding affinity optimization. |
Protein Structure Prediction Advances | Advancements in Protein Structure Prediction | Use of deep learning methods like AlphaFold for accurate protein structure prediction; introduction of new metrics (GDT-TS, TM-score, LDDT). |
Protein Sequence Generation and Design | Models for Amino Acid Sequence Generation | Development of models like ProteinSolver, PiFold, and ABACUS-R for generating protein sequences from structures; advancements in GNN and Transformer-based sequence design (e.g., GVP-GNN, ESM-IF1, ProteinMPNN). |
De Novo Protein Backbone Design | Models for De Novo Protein Backbone Generation | Introduction of ProtDiff, FoldingDiff, LatentDiff, FrameDiff, Genie, and RFDiffusion models for generating novel protein structures from scratch or based on motifs, with higher consistency and designability. |
Peptide-Specific AI Models | Models for Peptide Design | Development of peptide-specific AI models like MMCD for therapeutic peptide generation and co-designing sequence and structure; introduction of AdaNovo for de novo peptide sequencing from mass spectrometry data. |
This timeline outlines key advancements and shifts in generative AI techniques for drug design, showcasing the progression from traditional methods to modern AI-driven approaches.
Key Areas of De Novo Drug Design: Molecule and Protein Generation
De novo drug design can be broadly categorized into two key areas: small molecule generation and protein generation. Both of these areas require unique approaches and face distinct challenges, but both are critical in the pursuit of next-generation therapeutics.
Small Molecule Generation
The first area of focus is small molecule generation, which aims to create novel molecular compounds that can serve as drug candidates. These molecules are designed to be stable, valid, and “drug-like,” meaning they have the necessary properties to be developed into pharmaceutical products. Traditional methods for generating small molecules involve screening vast chemical libraries, which is a costly and time-consuming process. AI-driven generative models, however, offer a way to circumvent these limitations by generating molecules that are not constrained by previously known compounds.
Generative models used for small molecule design can be divided into two main approaches:
- Target-Agnostic Molecule Design: This involves generating molecules without considering any specific biological target. The goal is to produce novel, stable, and unique compounds that could have potential therapeutic value.
- Target-Aware Molecule Design: In contrast, target-aware generation focuses on designing molecules that specifically interact with a defined biological target, such as a particular protein receptor. This method can be further divided into ligand-based drug design (LBDD), which uses protein sequences, and structure-based drug design (SBDD), which uses 3D structural information of the target protein.
AI has shown significant promise in both of these approaches. Notable AI models such as variational autoencoders (VAEs) and generative adversarial networks (GANs) are being employed to generate novel molecules that are not only chemically valid but also possess the potential for pharmaceutical application.
Protein Generation
The second major area of de novo drug design is protein generation, which focuses on the creation or modification of proteins for therapeutic applications. Protein engineering is central to advancements in fields such as synthetic biology, immunotherapy, and targeted drug delivery. Unlike small molecule drugs, which are often designed to interact with specific targets, proteins must be designed to fold into functional 3D structures that adhere to biological constraints.
Protein design faces unique challenges, including the complexity of protein folding and the need to create functional structures that can perform specific biological tasks. De novo protein design is an exciting area of research because it enables the creation of proteins that are not limited by natural evolutionary processes. AI models such as AlphaFold2 and RoseTTAFold have made great strides in predicting protein structures, while generative models are now being used to create entirely new protein sequences.
Key AI Models in Generative Drug Design
Generative AI models have become essential tools in both small molecule and protein generation. Some of the most influential model architectures include:
- Variational Autoencoders (VAEs): VAEs work by learning a compressed representation of input data, which is then used to generate new data points. This architecture has been successfully applied to molecule generation, where the model learns to represent chemical compounds in a latent space and can then sample new molecules from this space.
- Generative Adversarial Networks (GANs): GANs consist of two neural networks—the generator and the discriminator—that compete against each other to produce realistic data. GANs are particularly effective in generating realistic molecular structures by iterating between generating new data and refining it based on feedback from the discriminator.
- Diffusion Models: These models gradually add noise to data and learn to reverse the noise to generate new data. Diffusion models have proven highly effective in generating complex structures, particularly in the case of molecular graphs.
- Graph Neural Networks (GNNs): GNNs are used to capture the graph-like structure of molecules, which is essential for understanding molecular interactions and properties. When paired with generative methods, GNNs can help create more accurate and biologically relevant molecular structures.
Applications in Drug Discovery: Real-World Impact
Generative AI models are not just theoretical constructs—they are actively being applied to real-world drug discovery efforts. Several biotechnology companies have already begun leveraging AI to generate novel small molecules, with over 150 AI-generated small-molecule drugs currently in the discovery phase and 15 in clinical trials. Additionally, protein engineering has opened up new possibilities for targeted therapies, especially in the fields of immunology and cancer treatment.
The ability to generate molecules and proteins with tailored properties has vast implications for personalized medicine. By designing drugs that specifically target an individual’s genetic makeup or disease profile, AI can enable more effective treatments with fewer side effects.
Challenges and Future Directions
While the progress in generative AI for drug design is undeniable, several challenges remain. Key hurdles include:
- Complexity and Scalability: Current models still struggle with generating large and highly complex molecules or proteins.
- Benchmarking and Evaluation: Standardized benchmarks for evaluating AI models are lacking, making it difficult to compare different approaches and assess their true effectiveness.
- Interpretability: Most generative models remain “black boxes,” making it challenging for researchers to understand why a particular model generates a successful outcome. There is a growing need for more transparent and interpretable AI methods in drug design.
Looking ahead, the future of generative AI in drug discovery is incredibly promising. Researchers are actively working on overcoming these challenges, and with advancements in AI techniques such as improved diffusion models and the increasing use of graph-based neural networks, we can expect even greater breakthroughs in the years to come.
Conclusion
Generative AI is transforming the landscape of drug discovery, particularly in the creation of novel molecules and proteins from scratch. By bypassing traditional methods that rely on existing chemical libraries, AI models open up new possibilities for discovering drugs that were previously unimaginable. While there are challenges to address, the progress made so far is remarkable, and the continued evolution of generative AI promises to revolutionize the pharmaceutical industry, accelerating the development of more effective, personalized, and accessible treatments. The future of drug design lies in the innovative intersection of AI, biology, and chemistry—shaping the next generation of therapeutics.
Frequently Asked Questions about Generative AI for Drug Design
1. What is de novo drug design and how does it differ from traditional drug discovery methods?
De novo drug design focuses on generating entirely novel biological compounds from scratch, unlike traditional methods that primarily screen existing chemical libraries or optimize known molecules. This approach allows researchers to explore a much larger chemical space, potentially uncovering unique and effective drugs that might not be found through conventional means. Traditional methods, like virtual screening and directed evolution, optimize and expedite tasks within existing frameworks, whereas de novo design attempts to generate entirely new molecules.
2. What are the main areas of focus within generative AI for de novo drug design?
This field primarily focuses on two key areas: small molecule generation and protein generation. Small molecule generation is concerned with creating novel, drug-like molecules, often with the aim of targeting specific proteins. Protein generation includes tasks like predicting protein structure from its sequence, designing new protein sequences for a given structure (or for no structure), and designing protein backbones from scratch. This also includes antibody and peptide generation given their high relevance. Both areas utilize similar concepts of generative modeling, but have different chemical nuances and challenges.
3. What are some common generative AI models used for molecule and protein generation?
Several generative models are widely used, including Variational Autoencoders (VAEs), Generative Adversarial Networks (GANs), Flow-based models, and Diffusion models. Each model has its own way of learning the distributions of the training data to sample new points from scratch. Additionally, graph neural networks (GNNs), and specifically equivariant graph neural networks (EGNNs), are often paired with these generative methods to effectively capture the structural aspects of molecules and proteins. Diffusion models, combined with EGNNs, are currently showing exceptional promise in this field.
4. How are generative models evaluated for molecule generation tasks?
For target-agnostic molecule generation, models are evaluated on the stability, validity, uniqueness, and novelty of the generated molecules. Quantitative Estimate of Drug-Likeness (QED) and properties of specific molecules are often tested as well. For target-aware molecule generation, evaluation metrics include binding affinity (measured by scores like Vina Score and Vina Energy), diversity of the generated molecules, as well as QED and synthetic accessibility (SA) scores. Models are often evaluated by properties of generated structures or by how well they can match a property given as a condition for generation.
5. What are the main challenges in applying generative AI for molecule generation?
Key challenges include generating molecules that are both valid (satisfying chemical constraints) and stable, and achieving scalability to generate larger, more complex molecules. Another key challenge is designing molecules that have a high binding affinity to specific protein targets while maintaining “drug-likeness,” a critical aspect for pharmaceutical applicability. Current models do not perform well at both of these goals simultaneously.
6. How is protein structure prediction different from protein sequence design, and how are they evaluated?
Protein structure prediction focuses on generating the 3D structure of a protein from its amino acid sequence, a very complex problem given the vast space of structural possibilities. Evaluation metrics for this task include RMSD (Root-Mean-Square Deviation), GDT-TS (Global Distance Test-Total Score), TM-score (Template Modeling score), and LDDT (Local-Distance Difference Test), which all assess the structural similarity between the predicted and true protein structures. Protein sequence design focuses on the inverse task of creating an amino acid sequence that folds into a desired structure (which may or may not be a structure from existing proteins). Evaluation metrics include Amino Acid Recovery (AAR), RMSD, perplexity (PPL), and a measure of rationality of polar/nonpolar presence.
7. What is backbone design in protein generation and how is it evaluated?
Backbone design focuses on creating protein structures from scratch, rather than merely predicting the structures of known proteins. Models in this field generate coordinates for the backbone atoms for each amino acid (nitrogen, alpha-carbon, carbonyl, and oxygen atom), with side-chain packing generally performed by external tools. Evaluation metrics include self-consistency TM (scTM) score and self-consistency RMSD (scRMSD), which measure how well a designed backbone can be folded into a protein. The scTM score simulates the folding process by using generated structures to generate sequences which are then converted back to sample structures by a model like AlphaFold to test similarity.
8. How is AI being applied to peptide design, and what are some unique considerations for peptides?
AI is used in peptide design for tasks such as generating novel peptides, predicting peptide sequences from mass spectrometry data, and optimizing peptide-protein interactions. Peptides, being smaller and more flexible than proteins, require distinct computational models. Peptide design considers properties unique to peptides like their greater structural flexibility, and different applications, such as drug delivery, antimicrobial peptides, and anticancer peptides. Generative modeling for peptides seeks to generate peptides with desired properties using a diverse array of generative models.
Glossary of Key Terms
- De Novo Drug Design: The process of designing new drug molecules from scratch, rather than modifying existing ones.
- Generative Model: A type of model that learns the underlying distribution of training data and can be used to generate new data points with similar properties.
- Virtual Screening: A computational technique used to screen large libraries of molecules to identify those that might bind to a specific target.
- Directed Evolution: A method to optimize and modify biological molecules through iterative mutation and selection processes.
- Small Molecules: Organic compounds with low molecular weight, typically used as drugs.
- Protein: Large biomolecules made of amino acids, playing various structural and functional roles.
- Ligand: A molecule that binds to a protein or other biological target.
- Antibody: A protein produced by the immune system to neutralize foreign objects.
- Peptide: A short chain of amino acids; smaller than a protein.
- SMILES (Simplified Molecular-Input Line-Entry System): A textual notation for representing molecular structures.
- Graph Neural Networks (GNNs): A neural network architecture designed to operate on graph-structured data.
- Equivariant Graph Neural Networks (EGNNs): A GNN that is equivariant to various symmetries and transformations, useful for preserving the physics and geometry of molecules and proteins.
- VAE (Variational Autoencoder): A generative model that learns a compressed representation of the input data using an encoder and generates new data using a decoder.
- Reconstruction Loss: A measure of how well a generative model can reconstruct its input after encoding and decoding.
- KL Divergence: A measure of the difference between two probability distributions.
- GAN (Generative Adversarial Network): A generative model that uses two competing networks, a generator and a discriminator, to generate new data.
- Flow-based Model: A generative model that uses a series of invertible transformations to map a simple distribution to a complex one.
- Diffusion Model: A generative model that adds noise to data and learns to reverse this process to generate new samples.
- Target-Agnostic Molecule Design: Designing molecules without regard to a specific biological target.
- Target-Aware Molecule Design: Designing molecules to bind to a specific biological target, often through structure-based or ligand-based techniques.
- LBDD (Ligand-Based Drug Design): Drug design based on the properties of known ligands.
- SBDD (Structure-Based Drug Design): Drug design based on the 3D structure of the target protein.
- RMSD (Root-Mean-Square Deviation): A metric for comparing the similarity between two structures, such as protein structures, measuring the average distance between atom positions.
- GDT-TS (Global Distance Test-Total Score): A metric that evaluates protein structural similarity by finding the best fit between two structures based on the number of matching residues.
- TM-Score (Template Modeling Score): A protein structure similarity metric that normalizes the GDT-TS by the protein length.
- AAR (Amino Acid Recovery): The proportion of correctly predicted amino acids in a protein sequence.
- PPL (Perplexity): A measure of how well a model predicts a sequence of data, often used in language models and protein design.
- scTM (Self-Consistency TM Score): A measure of the designability of protein structures via comparison of structure outputs with their sequence inputs in a generative process.
- scRMSD (Self-Consistency RMSD): Similar to scTM, but using RMSD to compare generated and sampled structures to test the designability of generated proteins.
- Peptide Sequencing: Determining the amino acid sequence of a peptide from mass spectrometry data.
- Mass Spectrometry: An analytical technique that measures the mass-to-charge ratio of ions to identify and quantify molecules.
Reference
Tang, X., Dai, H., Knight, E., Wu, F., Li, Y., Li, T., & Gerstein, M. (2024). A survey of generative AI for de novo drug design: new frontiers in molecule and protein generation. Briefings in Bioinformatics, 25(4), bbae338.