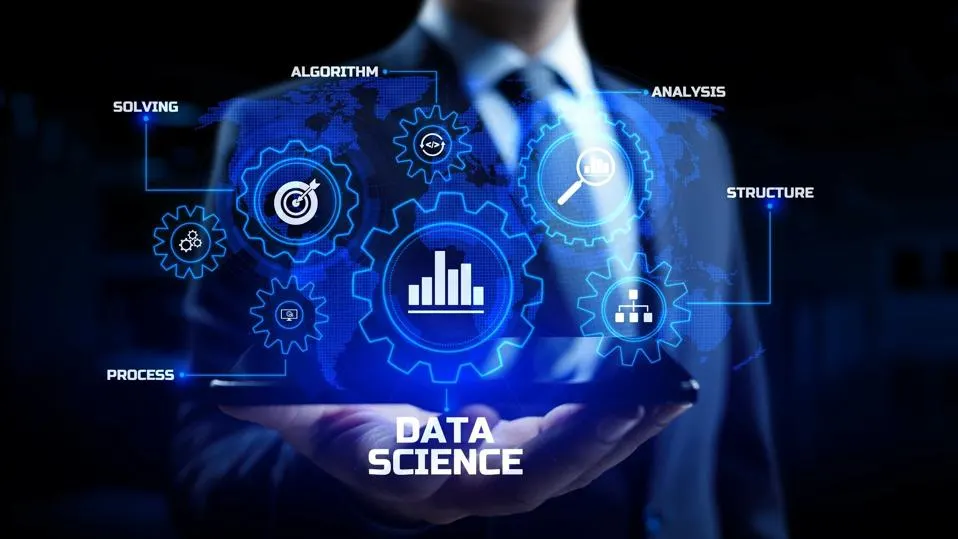
Navigating the World of Big Data: Mastering the Art of Data Science: Top Courses for Aspiring Data Scientists
December 6, 2023I. Introduction
In the era of Big Data, the growing importance of data science has become a defining factor for businesses, industries, and decision-makers. As vast amounts of data continue to be generated, collected, and stored, the role of data science in extracting valuable insights and making informed decisions has never been more crucial. This introduction aims to provide a brief overview of the escalating significance of data science in the context of Big Data and emphasize the importance of acquiring skills through specialized courses.
1. Big Data Landscape:
- The digital landscape has undergone a paradigm shift with the advent of Big Data. Organizations are grappling with unprecedented volumes, velocities, and varieties of data. Traditional methods of data analysis are insufficient to extract meaningful patterns and insights from this deluge of information.
2. The Role of Data Science:
- Data science has emerged as the key discipline to navigate the complexities of Big Data. It combines expertise in statistics, mathematics, programming, and domain knowledge to uncover hidden patterns, trends, and correlations within massive datasets. Data scientists play a pivotal role in transforming raw data into actionable insights.
3. Business and Decision-Making Impact:
- The impact of data science extends across various sectors, including finance, healthcare, marketing, and technology. Businesses leverage data science to enhance decision-making processes, optimize operations, identify market trends, and gain a competitive edge. Data-driven insights are increasingly becoming indispensable for strategic planning.
4. Specialized Courses in Data Science:
- The demand for skilled data scientists has surged in response to the expanding role of data in business and research. Specialized courses in data science offer a structured and comprehensive approach to acquiring the necessary skills. These courses cover topics such as statistical analysis, machine learning, data visualization, and programming languages like Python and R.
5. Importance of Skill Acquisition:
- Acquiring skills through specialized courses is essential for individuals seeking to enter or advance in the field of data science. These courses provide a foundation in theoretical concepts and hands-on experience with practical applications. They enable professionals to stay abreast of evolving technologies and methodologies in data science.
6. Career Opportunities:
- Data science skills are in high demand, and individuals with expertise in this field are well-positioned for rewarding career opportunities. From data analysts and machine learning engineers to data scientists and AI specialists, the job market offers diverse roles for those with a strong foundation in data science.
7. Addressing the Skills Gap:
- The rapid evolution of data science technologies has led to a skills gap in the workforce. Specialized courses bridge this gap by offering structured learning paths, hands-on projects, and industry-relevant curriculum. They empower individuals to develop the expertise needed to tackle real-world data challenges.
In conclusion, the era of Big Data underscores the vital role of data science in transforming raw information into actionable insights. Acquiring skills through specialized courses becomes not only a strategic advantage for individuals but also a necessity to meet the demands of a data-driven world. As organizations continue to harness the power of data, the relevance of data science and the importance of honing its skills will only continue to grow.
II. Why Pursue Data Science Courses
A. Career Opportunities
1. High Demand for Data Scientists:
- In today’s data-driven world, there is an exponential demand for professionals who can extract meaningful insights from large datasets. Data scientists are at the forefront of this demand, with organizations across industries seeking skilled individuals to help them navigate the complexities of Big Data.
- The proliferation of data sources, coupled with advancements in technology, has created an environment where businesses recognize the strategic importance of data science in gaining a competitive edge. As a result, the demand for data scientists continues to outpace the supply of qualified professionals.
2. Lucrative Job Prospects:
- Pursuing data science courses opens doors to lucrative job prospects. The scarcity of skilled data scientists has led to competitive salary offerings, making data science one of the most financially rewarding career paths. Organizations are willing to invest in top talent capable of deriving actionable insights from data.
- Data scientists often enjoy competitive compensation packages that reflect the specialized nature of their skills. The value they bring to organizations in terms of informed decision-making and strategic planning positions them as key contributors to an organization’s success.
3. Diverse Industry Applications:
- The versatility of data science skills allows professionals to work across diverse industries. Whether in finance, healthcare, e-commerce, marketing, or technology, data scientists play a crucial role in uncovering patterns, identifying trends, and driving innovation.
- The broad applicability of data science skills ensures that individuals have the flexibility to explore industries aligned with their interests and expertise. This diversity contributes to a dynamic and ever-evolving career landscape for data scientists.
4. Career Growth Opportunities:
- Data science is not just a job; it is a career path with abundant growth opportunities. As organizations increasingly rely on data-driven insights to inform their strategic decisions, the role of data scientists becomes integral to the core functions of businesses.
- Data scientists often find themselves in positions where they can progress into leadership roles, taking on responsibilities such as leading data teams, shaping data strategies, and driving innovation within organizations. Continuous advancements in the field provide opportunities for professionals to upskill and stay at the forefront of industry trends.
5. Innovation and Impact:
- Data scientists are at the forefront of driving innovation and making a substantial impact on business outcomes. By leveraging advanced analytics, machine learning, and artificial intelligence, data scientists contribute to the development of new products, services, and solutions that can revolutionize industries.
- The ability to bring about positive change and contribute to groundbreaking discoveries is a compelling aspect of a career in data science. Professionals in this field have the opportunity to be at the intersection of technology, business, and scientific inquiry.
6. Global Relevance:
- The skills acquired through data science courses have global relevance. Data-driven decision-making is a universal trend, and organizations worldwide are seeking individuals who can harness the power of data to drive innovation and solve complex challenges.
- Data scientists often find opportunities to work with global teams, collaborate on international projects, and contribute to solutions that have a widespread impact. The global nature of data science ensures that skilled professionals can explore career opportunities on a global scale.
In summary, pursuing data science courses is a strategic investment in a career path that offers high demand, lucrative job prospects, diverse industry applications, continuous career growth, and the opportunity to drive innovation with a global impact. As organizations increasingly recognize the value of data-driven decision-making, the role of data scientists will continue to be central to shaping the future of various industries.
B. Skill Development
1. Importance of Acquiring Technical Skills:
- Data Analysis:
- Acquiring proficiency in data analysis is fundamental to data science. Data analysts use tools and techniques to clean, process, and analyze large datasets, extracting valuable insights. Skills in data manipulation, exploratory data analysis, and statistical analysis are essential for interpreting complex datasets.
- Machine Learning:
- Machine learning is a core component of data science, enabling the development of models that can predict outcomes, classify data, and identify patterns. Acquiring skills in machine learning involves understanding algorithms, model training, and evaluating model performance—a critical aspect of building predictive models.
- Statistics:
- Statistical knowledge is foundational for drawing valid conclusions from data. Data scientists need to understand probability, hypothesis testing, and statistical inference to make informed decisions based on data patterns. Statistical skills are crucial for designing experiments, conducting analyses, and validating results.
2. Relevance of Practical, Hands-on Experience:
- Real-World Application:
- The application of theoretical concepts in real-world scenarios is a hallmark of effective data science. Hands-on experience allows individuals to bridge the gap between theory and practice, gaining a deeper understanding of how to approach and solve complex data-related challenges encountered in professional settings.
- Project-Based Learning:
- Practical, project-based learning is invaluable for reinforcing technical skills. Engaging in hands-on projects, such as building machine learning models, analyzing datasets, and solving data-driven problems, provides a tangible showcase of one’s abilities. It also allows for the development of a portfolio that demonstrates practical applications of acquired skills.
- Problem-Solving and Critical Thinking:
- Hands-on experience cultivates problem-solving and critical-thinking skills. Working on real-world projects requires individuals to navigate uncertainties, make decisions based on incomplete information, and iterate on solutions. This iterative problem-solving process is central to the day-to-day challenges faced by data scientists.
- Collaboration and Communication:
- Practical experience enhances collaboration and communication skills. Data scientists often work in interdisciplinary teams, collaborating with domain experts, business stakeholders, and other professionals. Effectively communicating findings, insights, and recommendations is crucial for the successful application of data science in diverse industries.
- Tools and Technologies:
- Familiarity with industry-relevant tools and technologies is essential. Hands-on experience with data science tools, programming languages (e.g., Python, R), and frameworks (e.g., TensorFlow, scikit-learn) equips individuals with the technical proficiency needed to navigate the data science ecosystem.
3. Continuous Learning and Adaptation:
- Dynamic Nature of Data Science:
- Data science is a dynamic field that continually evolves with technological advancements and changing industry needs. Acquiring practical skills fosters a mindset of continuous learning and adaptation, enabling professionals to stay current with emerging trends and technologies.
- Professional Growth:
- Continuous learning is a key driver of professional growth. As data science evolves, individuals who actively seek opportunities to expand their skill set, embrace new methodologies, and stay abreast of industry developments are better positioned for long-term success and career advancement.
- Adaptability to Industry Demands:
- Hands-on experience enhances adaptability to industry demands. Professionals who can apply their skills to a variety of use cases, industries, and data types are better equipped to address the diverse challenges posed by the evolving landscape of data science.
In conclusion, the pursuit of data science courses is not just about theoretical knowledge but also about acquiring the technical skills necessary for effective data analysis, machine learning, and statistical interpretation. Practical, hands-on experience is a critical component that bridges theory and application, fostering problem-solving abilities, collaboration skills, and adaptability—key attributes of successful data scientists.
III. Key Concepts in Data Science
A. Overview of Data Science
1. Definition and Scope:
- Data Science Defined:
- Data science is an interdisciplinary field that involves the extraction of meaningful insights and knowledge from data. It encompasses a range of techniques and methods, including statistical analysis, machine learning, data mining, and data visualization, to uncover patterns, trends, and correlations within large and complex datasets.
- Extracting Value from Data:
- At its core, data science aims to turn raw data into actionable insights that drive informed decision-making. It involves the entire data lifecycle—from data collection and cleaning to analysis and interpretation. The goal is to derive valuable knowledge that can inform strategic planning, optimize processes, and solve complex problems.
- Multifaceted Nature:
- Data science is multifaceted, drawing on expertise from various domains, including statistics, mathematics, computer science, and domain-specific knowledge. It integrates quantitative and qualitative approaches to extract insights, providing a holistic view of data and its implications.
- Business and Research Applications:
- The scope of data science extends across industries, including business, healthcare, finance, marketing, and research. It is applied to diverse domains, addressing challenges such as predictive modeling, pattern recognition, natural language processing, and optimization.
- Integration of Disciplines:
- Data science is inherently interdisciplinary, blending techniques and methodologies from multiple disciplines to create a cohesive approach to data analysis. It combines elements of statistics, computer science, and domain-specific knowledge to address complex problems.
- Statistical Foundations:
- Statistical principles form the foundation of data science, providing methods for sampling, hypothesis testing, and drawing inferences from data. Statistical techniques help validate findings and assess the reliability of patterns identified during analysis.
- Computer Science and Programming:
- Programming and computational skills are integral to data science. Professionals in this field use programming languages such as Python or R to manipulate data, implement algorithms, and create data visualizations. Proficiency in programming facilitates the automation of data analysis processes.
- Domain Knowledge:
- Data science often involves collaboration with domain experts who possess specialized knowledge in a particular field. Whether it’s healthcare, finance, or marketing, understanding the context and nuances of the domain is crucial for formulating relevant questions, selecting appropriate data features, and interpreting results.
- Machine Learning and Predictive Modeling:
- Machine learning techniques play a pivotal role in data science, enabling the development of predictive models. These models learn from data patterns and make predictions or classifications. The interdisciplinary nature of data science allows for the integration of machine learning algorithms tailored to specific problems.
- Data Visualization and Communication:
- Effective communication of findings is a key aspect of data science. Data scientists use visualization tools to convey insights in a comprehensible manner. The ability to communicate complex technical concepts to non-technical stakeholders is essential for driving informed decision-making.
- Responsible Data Use:
- Data science practitioners must adhere to ethical guidelines and principles. The responsible use of data involves considerations such as privacy, consent, and fairness. Ethical data practices ensure that data scientists handle information with integrity and respect for individuals’ rights.
- Bias and Fairness:
- Data scientists need to be mindful of potential biases in data and algorithms. Addressing bias ensures that data-driven decisions are fair and equitable. Ongoing efforts to mitigate biases contribute to the responsible and ethical practice of data science.
- Transparency and Accountability:
- Transparency in data science involves documenting methodologies, disclosing limitations, and providing clear explanations of findings. Accountability ensures that data scientists take responsibility for the impact of their analyses and actively work to address any unintended consequences.
In summary, data science is defined by its goal of extracting insights from data to inform decision-making. Its interdisciplinary nature draws on statistical principles, computer science, domain-specific knowledge, and ethical considerations to create a holistic approach to data analysis. The integration of diverse disciplines allows data science to address complex challenges and drive innovation across various industries.
B. Core Data Science Skills
1. Programming Languages:
- Python:
- Python is one of the most widely used programming languages in data science. Its simplicity, readability, and extensive libraries make it a preferred choice for tasks ranging from data manipulation to machine learning. Python’s ecosystem includes popular libraries like NumPy, Pandas, Matplotlib, and Scikit-learn, providing tools for data analysis, visualization, and machine learning.
- Significance:
- Versatility: Python is versatile and supports a wide range of data science tasks, from data cleaning and preprocessing to complex machine learning tasks.
- Community Support: The Python data science community is robust, contributing to the development of libraries and frameworks that enhance productivity.
- Integration: Python integrates well with other languages and tools, making it a suitable language for end-to-end data science workflows.
- R:
- R is a programming language and environment specifically designed for statistical computing and data analysis. It excels in statistical modeling, data visualization, and exploratory data analysis. R’s comprehensive set of statistical packages makes it a powerful tool for statisticians and data scientists.
- Significance:
- Statistical Analysis: R is well-suited for statistical tasks, making it a preferred language for researchers and statisticians.
- Data Visualization: R provides a range of packages for creating sophisticated data visualizations, facilitating effective communication of insights.
- Community for Research: R has a strong presence in academic and research communities, making it a valuable tool for data scientists in those domains.
2. Statistical Analysis:
- Importance of Statistical Knowledge:
- Statistical analysis is fundamental to data science, providing the tools and techniques to make inferences from data. Statistical methods help data scientists identify patterns, test hypotheses, and validate findings. Key aspects include:
- Descriptive Statistics:
- Descriptive statistics summarize and describe the main features of a dataset. Measures such as mean, median, and standard deviation provide insights into the central tendency and spread of data.
- Inferential Statistics:
- Inferential statistics involve making predictions or inferences about a population based on a sample of data. This includes hypothesis testing, confidence intervals, and regression analysis.
- Probability Distributions:
- Understanding probability distributions is crucial for modeling uncertainty in data. Common distributions, such as normal and binomial distributions, are foundational to statistical analysis.
- Application in Data Science:
- Statistical analysis guides decision-making in data science. It helps identify whether observed patterns are statistically significant, assesses the reliability of predictions, and provides a framework for drawing conclusions from data.
3. Machine Learning:
- Basic Concepts:
- Machine learning involves the development of algorithms that enable computers to learn patterns from data and make predictions or decisions without being explicitly programmed. Key concepts include:
- Supervised Learning:
- In supervised learning, models are trained on labeled data, where the input features are paired with corresponding target labels. The model learns to map inputs to outputs and can make predictions on new, unseen data.
- Unsupervised Learning:
- Unsupervised learning deals with unlabeled data, where the algorithm identifies patterns or relationships without predefined target labels. Clustering and dimensionality reduction are common unsupervised learning tasks.
- Feature Engineering:
- Feature engineering involves selecting, transforming, or creating input features to improve the performance of machine learning models. It plays a crucial role in extracting relevant information from raw data.
- Applications:
- Machine learning has diverse applications in data science, including:
- Predictive Modeling: Building models to predict future outcomes, such as sales forecasts, stock prices, or customer behavior.
- Classification: Categorizing data into different classes or groups based on patterns identified in the training data.
- Clustering: Identifying natural groupings or clusters within a dataset.
- Recommendation Systems: Creating systems that recommend products, services, or content based on user preferences.
- Natural Language Processing (NLP): Analyzing and understanding human language, enabling tasks such as sentiment analysis and language translation.
- Role in Data Science Workflow:
- Machine learning is a key component of the data science workflow, allowing data scientists to build predictive models that can automate decision-making processes and uncover insights from complex datasets.
In conclusion, core data science skills encompass proficiency in programming languages such as Python and R, a solid foundation in statistical analysis for drawing meaningful conclusions from data, and knowledge of machine learning concepts for building predictive models and uncovering patterns in diverse datasets. These skills collectively empower data scientists to navigate the intricacies of data science workflows and derive actionable insights from complex data.
IV. Top Data Science Courses
A. Online Learning Platforms
1. Coursera:
- Popular Data Science Courses:
- “Data Science and Machine Learning Bootcamp with R”
- Offered by Udemy on Coursera, this bootcamp covers R programming, statistical concepts, and machine learning techniques. It is suitable for beginners and provides hands-on experience with real-world datasets.
- “Applied Data Science with Python Specialization”
- Provided by the University of Michigan, this specialization on Coursera covers Python programming, data visualization, machine learning, and text mining. It includes practical projects and a capstone to apply learned skills.
- “Machine Learning” by Stanford University
- This course, taught by Andrew Ng, is one of the most renowned in the field. It covers machine learning concepts, algorithms, and applications. The course includes both theoretical understanding and practical implementation.
- “Data Science and Machine Learning Bootcamp with Python”
- Similar to the R bootcamp, this course on Coursera, offered by Udemy, focuses on Python programming, data analysis, and machine learning. It is designed for beginners and includes hands-on projects.
- “Data Science and Machine Learning Bootcamp with R”
2. edX:
- Relevant Programs and Certifications:
- “MicroMasters Program in Data Science” by University of California, San Diego (UCSD)
- This program on edX covers a comprehensive curriculum, including statistical concepts, machine learning, and big data technologies. Completion of the MicroMasters can be credited towards a full master’s degree.
- “Professional Certificate in Data Science” by Harvard University
- This program provides a hands-on introduction to data science using R and covers key topics such as data cleaning, exploration, and visualization. It is suitable for learners looking to build practical data science skills.
- “Microsoft Professional Program in Data Science”
- Offered on edX, this program is developed by Microsoft and covers the entire data science pipeline, including data exploration, statistical concepts, machine learning, and data visualization. It also includes a capstone project.
- “MicroMasters Program in Data Science” by University of California, San Diego (UCSD)
3. Udacity:
- Notable Data Science Nanodegree Programs:
- “Data Scientist Nanodegree”
- Udacity’s Data Scientist Nanodegree program covers foundational data science skills, including data wrangling, statistical analysis, and machine learning. It includes hands-on projects and real-world applications.
- “AI for Healthcare Nanodegree”
- Focused on the intersection of data science and healthcare, this nanodegree program on Udacity covers topics such as healthcare data, predictive modeling, and deploying machine learning models for healthcare applications.
- “Data Engineering Nanodegree”
- For learners interested in the data engineering aspect of data science, this program covers topics such as data modeling, data warehouses, and building data pipelines. It provides hands-on experience with industry-relevant tools.
- “Data Scientist Nanodegree”
These online learning platforms offer a variety of data science courses and programs catering to learners with different levels of expertise. Whether you’re a beginner looking to start your data science journey or an experienced professional seeking advanced skills, these platforms provide flexible and accessible learning opportunities with hands-on projects and industry-relevant content.
B. University Programs
1. Master’s in Data Science:
- Overview of Reputable Universities Offering Advanced Degrees:
- Massachusetts Institute of Technology (MIT) – Master of Business Analytics:
- MIT offers a Master of Business Analytics program that integrates business and data science. It covers topics such as machine learning, optimization, and data visualization. The program is designed for individuals seeking to apply data science in a business context.
- Stanford University – Master of Science in Statistics: Data Science:
- Stanford’s Master of Science in Statistics with a concentration in Data Science is an interdisciplinary program. It covers statistical methods, machine learning, and big data technologies. The program emphasizes the application of statistical methods to real-world problems.
- University of California, Berkeley – Master of Information and Data Science (MIDS):
- Berkeley’s MIDS program is offered through the School of Information. It covers a wide range of topics, including data visualization, machine learning, and ethical considerations in data science. The program is designed for working professionals.
- Columbia University – Master of Science in Data Science:
- Columbia’s MS in Data Science program covers both the technical and practical aspects of data science. It includes coursework in machine learning, data visualization, and big data technologies. Students also work on real-world projects.
- Harvard University – Master of Science in Data Science:
- Harvard’s Master of Science in Data Science program is offered by the Harvard John A. Paulson School of Engineering and Applied Sciences. It covers topics such as data wrangling, statistical modeling, and machine learning. The program includes a capstone project.
- Massachusetts Institute of Technology (MIT) – Master of Business Analytics:
2. Specialized Certifications:
- Recognized Certifications in Data Science:
- Certified Analytics Professional (CAP):
- Offered by the Institute for Operations Research and the Management Sciences (INFORMS), CAP is a vendor-neutral certification that validates expertise in analytics. It covers a broad range of analytics topics, including statistical analysis and predictive modeling.
- Microsoft Certified: Azure Data Scientist Associate:
- This certification, offered by Microsoft, is for data scientists using Azure Machine Learning to implement and run machine learning workloads on Azure. It validates skills in machine learning, data engineering, and model deployment.
- IBM Data Science Professional Certificate:
- This certificate program, offered on platforms like Coursera, is created by IBM. It covers key data science concepts and tools, including Python, Jupyter Notebooks, and machine learning with scikit-learn. Completion of the program provides a recognized certification.
- SAS Certified Data Scientist:
- SAS offers a certification for data scientists, which validates skills in data manipulation, feature engineering, and machine learning model development using SAS tools. The certification is recognized in the analytics industry.
- Cloudera Certified Data Scientist:
- Cloudera’s certification for data scientists focuses on skills related to big data and machine learning using the Cloudera platform. It covers areas such as data exploration, feature engineering, and model evaluation.
- Certified Analytics Professional (CAP):
These university programs and certifications are recognized for providing in-depth and specialized training in data science. University programs offer advanced degrees with a comprehensive curriculum, while certifications provide targeted validation of specific skills and expertise in the field. The choice between a degree program and a certification often depends on individual career goals and the depth of knowledge desired.
C. Specialized Topics
1. Natural Language Processing (NLP):
- “Natural Language Processing Specialization” on Coursera (offered by National Research University Higher School of Economics):
- This specialization covers key concepts in NLP, including text processing, sequence models, and language understanding. Learners work on projects that involve sentiment analysis, named entity recognition, and machine translation.
- “Applied Natural Language Processing with Python” on Udemy:
- This course on Udemy provides hands-on experience in building NLP applications using Python. Topics include text classification, sentiment analysis, and building chatbots. The course focuses on practical applications of NLP techniques.
2. Computer Vision:
- “Convolutional Neural Networks” on Coursera (offered by DeepLearning.AI):
- Part of the Deep Learning Specialization, this course delves into Convolutional Neural Networks (CNNs) and their application in computer vision. It covers image recognition, object detection, and neural style transfer.
- “Computer Vision Nanodegree” on Udacity:
- This nanodegree program provides a comprehensive exploration of computer vision concepts. Topics include image classification, object detection, and image segmentation. Learners work on projects involving real-world computer vision applications.
- “Time Series Analysis and Forecasting with Python” on Udemy:
- This course on Udemy focuses on time series analysis using Python. It covers topics such as seasonality, trend analysis, and forecasting techniques. Practical applications include financial forecasting and demand prediction.
- “Practical Time Series Analysis” on Coursera (offered by State University of New York):
- This course provides a practical approach to time series analysis, covering topics like autocorrelation, stationarity, and ARIMA models. Learners apply concepts to analyze and forecast time series data.
- “Reinforcement Learning Specialization” on Coursera (offered by University of Alberta):
- This specialization covers foundational concepts in reinforcement learning, including Markov decision processes, dynamic programming, and deep reinforcement learning. Learners implement reinforcement learning algorithms in practical scenarios.
- “Deep Reinforcement Learning Nanodegree” on Udacity:
- This nanodegree program focuses on deep reinforcement learning techniques. Topics include Q-learning, policy gradients, and actor-critic methods. Learners work on projects involving game playing and robotic control.
5. Big Data Technologies:
- “Big Data Specialization” on Coursera (offered by University of California, San Diego):
- This specialization covers various aspects of big data, including Hadoop, MapReduce, and Spark. It provides hands-on experience with processing large-scale datasets.
- “Big Data Engineer Nanodegree” on Udacity:
- This nanodegree program focuses on the skills needed to work as a big data engineer. It covers technologies such as Apache Spark and Hadoop, along with data modeling and processing.
- “Bayesian Methods for Machine Learning” on Coursera (offered by Higher School of Economics):
- This course introduces learners to Bayesian methods in the context of machine learning. Topics include Bayesian networks, probabilistic graphical models, and Bayesian parameter estimation.
- “Bayesian Statistics in Python” on Udemy:
- This course on Udemy provides a practical introduction to Bayesian statistics using Python. Topics include Bayesian reasoning, Bayesian modeling, and probabilistic programming.
Specialized courses in these niche areas offer in-depth exploration of advanced topics within the broader field of data science. Whether focusing on natural language processing, computer vision, time series analysis, reinforcement learning, big data technologies, or Bayesian statistics, these courses provide learners with specialized knowledge and skills applicable to specific domains and applications.
VII. Considerations for Choosing the Right Course
A. Accreditation and Recognition
1. Importance of Accredited Programs:
- Accreditation ensures that a program meets specific educational standards and quality benchmarks. When choosing a data science course, especially for advanced degrees, opting for accredited programs is crucial. Accreditation provides assurance that the curriculum, faculty, and overall educational experience meet recognized standards.
- Considerations:
- Look for programs accredited by relevant accreditation bodies or recognized institutions in the field of education.
- Accreditation can enhance the credibility of your qualifications and may be important for certain career paths.
B. Course Content
2. Reviewing Syllabi and Course Outlines:
- Thoroughly examining the syllabi and course outlines gives insight into the topics covered, depth of content, and alignment with your learning goals. Pay attention to the inclusion of practical applications, real-world projects, and the use of industry-relevant tools and technologies.
- Considerations:
- Ensure the curriculum covers a broad spectrum of data science concepts, from foundational to advanced topics.
- Look for hands-on projects and practical exercises that allow you to apply theoretical knowledge.
- Assess whether the course content aligns with your specific areas of interest within data science.
C. Industry Connections
3. Programs with Industry Partnerships and Real-World Projects:
- Industry connections and collaborations are valuable indicators of a program’s relevance to the professional landscape. Courses that partner with industry leaders, offer internships, or involve real-world projects provide a practical understanding of how data science is applied in the field.
- Considerations:
- Look for programs with partnerships or collaborations with industry organizations, companies, or professionals.
- Assess the inclusion of real-world projects, case studies, or internships that offer hands-on experience.
- Consider programs with faculty members who have industry experience or networks.
Additional Considerations:
4. Flexibility and Mode of Delivery:
- Consider the flexibility of the course, including the availability of part-time or online options. Assess whether the mode of delivery aligns with your learning preferences and accommodates your schedule.
5. Alumni Success and Placement Rates:
- Investigate the success stories of alumni from the program. High placement rates and notable career paths of graduates can indicate the program’s effectiveness in preparing individuals for the workforce.
6. Reviews and Testimonials:
- Seek reviews and testimonials from current or former students. Online platforms, forums, and social media can be valuable sources of feedback about the course, faculty, and overall learning experience.
7. Cost and Financial Considerations:
- Evaluate the overall cost of the course, including tuition, materials, and potential additional expenses. Consider any financial aid, scholarships, or payment plans that may be available.
8. Learning Resources and Support:
- Assess the availability of learning resources such as libraries, online platforms, and support services. Consider the level of support provided, including access to faculty, tutoring services, and technical support.
Choosing the right data science course involves a thorough evaluation of accreditation, course content, industry connections, flexibility, alumni success, reviews, cost, and available resources. By carefully considering these factors, you can select a program that aligns with your career goals and provides a high-quality learning experience.
IX. Conclusion
In conclusion, the significance of mastering data science through well-structured courses cannot be overstated in today’s dynamic and data-driven landscape. The field of data science plays a pivotal role in unlocking valuable insights from vast and complex datasets, driving innovation, informed decision-making, and solving real-world challenges. As technology continues to evolve, acquiring data science skills becomes increasingly crucial for professionals seeking to stay competitive and contribute meaningfully to their respective industries.
Throughout this exploration, we’ve touched upon key concepts in data science, specialized topics, and considerations for choosing the right course. We’ve showcased success stories of individuals who, through dedicated learning, not only mastered the intricacies of data science but also achieved remarkable career advancements and accomplishments in diverse fields.
For those considering a journey into data science or looking to enhance their existing skills, the suggested courses and learning platforms provide a roadmap to comprehensive education. Whether you are interested in foundational concepts, specialized topics like natural language processing or computer vision, or seeking advanced degrees from reputable universities, there is a diverse array of options tailored to your specific needs and career aspirations.
As you embark on this exciting journey, remember that mastering data science is not just about theoretical knowledge but also about gaining practical, hands-on experience. Embrace the opportunities to work on real-world projects, collaborate with industry professionals, and stay engaged with the vibrant data science community. Continuous learning and adaptation to emerging trends will be key to your long-term success in this ever-evolving field.
In the fast-paced world of data science, your commitment to ongoing education and skill development will set the stage for a rewarding and impactful career. Explore the suggested courses, seize the opportunities for growth, and position yourself at the forefront of innovation in the era of big data. Your journey into data science starts now—best of luck!