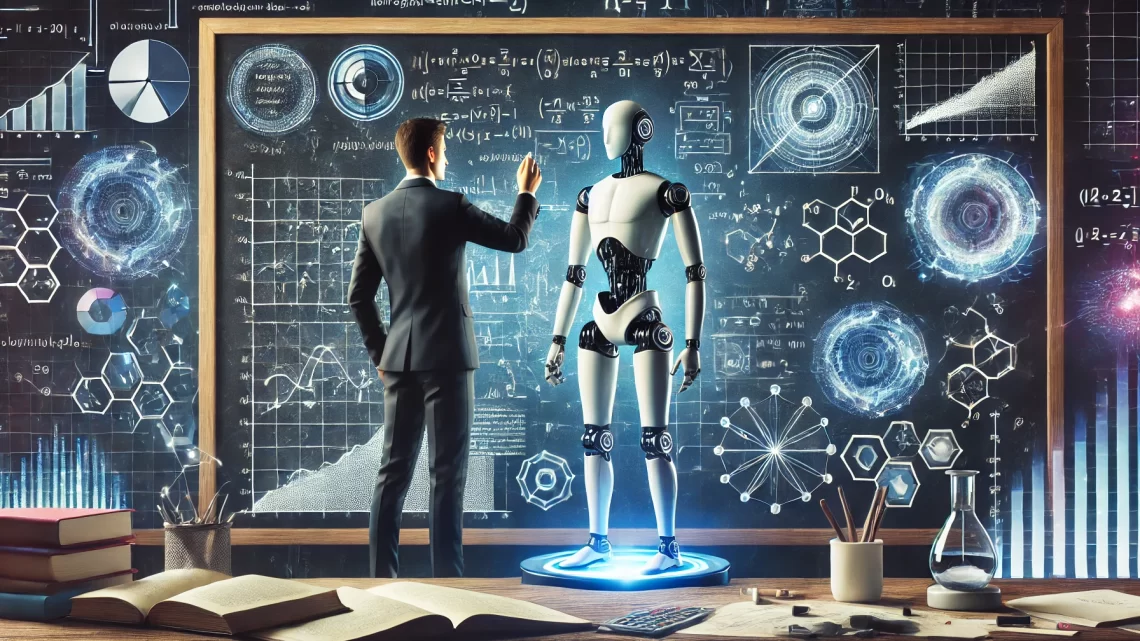
The Mathematical Capabilities of ChatGPT and GPT-4: Insights from GHOSTS Dataset
December 22, 2024Since its introduction in November 2022, ChatGPT has emerged as a widely recognized AI-driven question-and-answer system. With its evolution, GPT-4 raised hopes for enhanced capabilities, particularly in specialized domains like mathematics. In a comprehensive study led by researchers across institutions like Oxford and Princeton, the mathematical prowess of ChatGPT (two versions from January 2023) and GPT-4 was scrutinized using an innovative dataset called GHOSTS.
The GHOSTS Dataset: Pioneering Mathematical Evaluation
GHOSTS (Graduate-level Holistic Overview of Skills in Mathematical Reasoning) represents the first of its kind—a curated dataset testing language models on graduate-level mathematics. This dataset is divided into six subcategories:
- Grad-Text: Exercises from graduate-level textbooks.
- Holes-in-Proofs: Proofs with missing steps, challenging the model to fill the gaps.
- Olympiad-Problem-Solving: Complex problems akin to mathematical competitions.
- Symbolic-Integration: Testing models on integration problems beyond elementary levels.
- MATH: A mix of algebra and probability problems.
- Search-Engine-Aspects: Queries resembling tasks like theorem retrieval.
Accompanied by two subsets—miniGHOSTS and microGHOSTS—these datasets facilitate cost-effective evaluation of models by capturing the essence of their performance on mathematically intensive tasks.
How Did ChatGPT and GPT-4 Perform?
The study evaluated responses to over 1,600 prompts using metrics like accuracy, error types, and stability. Key findings include:
- Undergraduate-Level Proficiency: GPT-4 demonstrated sufficient capability for undergraduate mathematics but struggled with graduate-level complexity.
- Task-Specific Strengths: Both ChatGPT and GPT-4 excelled in factual queries and simple computations. However, performance dropped significantly for advanced proofs and symbolic integration.
- GPT-4’s Leap: Compared to ChatGPT, GPT-4 showed marked improvement, achieving higher accuracy and fewer errors in miniGHOSTS evaluations.
While GPT-4 achieved an average rating of 4.15 on a 5-point scale in miniGHOSTS, it faltered on graduate-level problems and mathematical olympiad challenges, reflecting the steep climb required to match human expertise.
Challenges and Insights
The models exhibited recurring challenges:
- Mistakes in Simple Calculations: Despite their sophistication, errors in basic arithmetic persisted.
- Overconfidence: Models rarely expressed uncertainty, even when outputs were incorrect.
- Ineffective Prompt Engineering: Modifying question phrasing improved performance only marginally.
Interestingly, the study highlighted GPT-4’s ability to deliver detailed, albeit sometimes rambling, explanations—beneficial for clarity but occasionally reducing readability.
Implications for Professional Use
The findings underscore that ChatGPT and GPT-4 are far from replacing human mathematicians but serve as powerful assistants. Their ability to function as mathematical search engines and provide contextually accurate notations can accelerate workflows for professionals already adept at validating results.
The Road Ahead
GHOSTS sets the stage for iterative improvement in mathematical reasoning by large language models. While current models lag behind domain-specific tools like symbolic solvers, they offer unmatched versatility, emphasizing their role as collaborative rather than standalone tools in mathematical research.
The research team encourages further exploration of GHOSTS and its subsets, aiming to build a robust benchmark for evaluating the mathematical potential of future AI models.
Conclusion
As we push the boundaries of AI capabilities, datasets like GHOSTS offer critical insights into both strengths and limitations. GPT-4’s advancements signal promising directions, but there remains substantial ground to cover before these models can handle the complexities of professional mathematics independently.
Frequently Asked Questions on the Mathematical Capabilities of ChatGPT and GPT-4
- What is the GHOSTS dataset, and what makes it unique compared to existing mathematical datasets for language models?
- The GHOSTS dataset is a newly created benchmark designed to evaluate the advanced mathematical abilities of Large Language Models (LLMs) like ChatGPT and GPT-4. It’s unique because it covers a broad range of mathematical skills, including answering computational questions, completing proofs, solving olympiad-level problems, and surveying mathematical literature. Unlike existing datasets that focus on basic mathematics or are very small, GHOSTS includes graduate-level material and aims to assess a holistic view of a model’s mathematical capabilities. It consists of carefully designed prompts and subdatasets that address different aspects of mathematical reasoning. Additionally, GHOSTS is the first natural-language dataset curated by working researchers in mathematics for the specific purpose of evaluating LLMs on professional mathematical tasks.
- What specific mathematical tasks does the GHOSTS dataset test, and what are the different subdatasets?
- The GHOSTS dataset tests a wide array of mathematical reasoning skills, covering several aspects of professional mathematical work. It is comprised of six subdatasets:
- Grad-Text: Questions based on exercises from graduate-level textbooks on functional analysis, topology, and probability theory, focusing on theorem proofs, and conceptual understanding.
- Holes-in-Proofs: Tasks requiring the completion of incomplete mathematical proofs, assessing the model’s ability to grasp the logic and structure of mathematical arguments.
- Olympiad-Problem-Solving: Problems from mathematical olympiads, challenging the models to generate original and insightful solutions.
- Symbolic-Integration: Focuses on symbolic computation skills, requiring the model to solve integration problems.
- MATH: Contains a variety of math problems from algebra, counting and probability, prealgebra, and precalculus.
- Search-Engine-Aspects: Tests the models ability to retrieve mathematical definitions and theorems, and to complete proof fragments by retrieving supporting definitions. This subdataset can be used to test the capability of the models to serve as a mathematical search engine.
- These subdatasets cover a variety of difficulty levels, question types, and out-of-distribution scenarios, making the benchmark comprehensive.
- How were ChatGPT and GPT-4 evaluated on the GHOSTS dataset, and what are the key findings? ChatGPT and GPT-4 were evaluated by submitting prompts from the GHOSTS dataset to each model, and the models’ outputs were then rated by human experts using a thorough methodology that included error codes and warning codes. This process involved a total of 1636 ratings, covering a variety of use cases from professional mathematician’s workflows, for example, the models were prompted to find mathematical objects given information about them. The key findings reveal that while these models can be surprisingly good at querying facts and acting as mathematical search engines or knowledge base interfaces, their performance drops considerably with increasing mathematical difficulty, especially for tasks at the graduate level. Although GPT-4 shows some improvements over ChatGPT, both models remain inconsistent and below the level of an average graduate student in mathematics. The models are often overly confident even when they make mistakes.
- What are the limitations of ChatGPT and GPT-4 when it comes to mathematical reasoning according to the study? The study identified several limitations in the mathematical reasoning abilities of ChatGPT and GPT-4. The models struggle with producing consistent, high-quality proofs and calculations, especially in advanced mathematics. They are prone to making algebraic mistakes, using incorrect logic, and overlooking edge cases. The models struggle with problems that require insightful and original solutions, such as those seen in olympiad math. In addition, they frequently fail on seemingly simple problems and can hallucinate solutions or offer irrelevant information. The researchers also observed that the models are often overconfident in their responses, making their mistakes difficult to detect without careful verification. Despite these limitations, the models exhibit promising capabilities in simpler cases, especially fact retrieval.
- What is the role of prompt engineering in the GHOSTS dataset, and how does it affect model performance? The GHOSTS dataset includes prompts created through various methods, some specifically using prompt engineering. For the Olympiad-Problem-Solving subdataset, several prompts were created using variations, and this allowed an analysis of the impact of prompt engineering on model performance. The study found that prompt engineering can slightly improve the models’ rating and reduce the frequency of certain error codes, such as errors that stem from a failure to understand the problem. However, even with prompt engineering, the models’ performance on difficult problems remains significantly inconsistent and far from perfect, indicating that prompt engineering alone isn’t enough to overcome the limitations of these LLMs when it comes to complex mathematical tasks.
- Besides GHOSTS, what are miniGHOSTS and microGHOSTS and why were they created? MiniGHOSTS and microGHOSTS are smaller subsets of the GHOSTS dataset created to enable more efficient and faster evaluation of mathematical capabilities in LLMs. MiniGHOSTS contains 170 prompts selected to match the mean and variance of ChatGPT’s performance on the full GHOSTS dataset, aiming to capture the “essence” of model performance with fewer data points, which reduces human evaluation costs. MicroGHOSTS is an even smaller set of 14 representative prompts designed for rapid pre-screening of LLMs. These subsets allow researchers to assess models with much lower evaluation costs. Each question of microGHOSTS comes with an answer, explanation, and common error modes to help evaluators who aren’t mathematicians.
- What are some typical error modes and failure cases that the study observed in ChatGPT’s responses? The study identified numerous error modes in ChatGPT’s responses across the different subdatasets. These include:
- Missing Information (e1): Omitting examples or steps necessary for a complete solution.
- Vague/Wrong Statements (e2, e3): Making imprecise or incorrect statements, sometimes to a small degree, sometimes to a large degree.
- Computational Errors (e4): Performing incorrect mathematical calculations, even basic arithmetic.
- Logical Errors (e5): Using faulty logic or an incorrect flow of arguments in proofs, with subtypes like missing steps (e5_2), unconsidered edge cases (e5_3), or unsupported inferences (e5_4).
- Ignoring Constraints (e6): Failing to adhere to specific constraints given in the problem statement. In addition to the specific error modes, the study also highlighted issues with ChatGPT and GPT-4 that are not specific to any one error code, such as a tendency to attempt induction proofs unnecessarily, a failure to use elementary techniques when they are sufficient, and a tendency to use more complex math than is necessary.
- How can the GHOSTS dataset be used by other researchers and what is the overall conclusion of the study? The GHOSTS dataset, including its mini and micro versions, is intended as a public resource for the evaluation of LLMs on advanced mathematical tasks. The dataset can be used to evaluate the models’ overall mathematical reasoning performance across various areas of mathematics. The comprehensive nature of the dataset in its size, types of questions asked, and breadth of covered mathematical fields will allow researchers to gain insight into how the models behave in different contexts. The authors encourage other researchers to use the data to discover new trends and patterns in the models’ responses, and to contribute to the field by creating better methods of evaluating the mathematical reasoning performance of LLMs. The overall conclusion is that while ChatGPT and GPT-4 have some impressive mathematical abilities in fact retrieval, and in acting as knowledge base interfaces or mathematical search engines, they are still not reliable for consistently generating correct, detailed, and complex proofs or calculations. The study highlights the need for more research to improve the mathematical reasoning abilities of LLMs
Glossary of Key Terms
- LLM (Large Language Model): A type of AI model trained on large amounts of text data to understand and generate human-like text. Examples include ChatGPT and GPT-4.
- GHOSTS Dataset: A natural-language mathematics dataset created by working mathematicians to measure advanced mathematical abilities of LLMs. It contains graduate-level mathematics problems and aims to provide a holistic overview of mathematical reasoning.
- miniGHOSTS Dataset: A reduced subset of the GHOSTS dataset used for rapid evaluation of language models with a dataset size of 170 prompts. The subset was selected to match the mean and variance of ChatGPT’s performance on the full GHOSTS dataset.
- microGHOSTS Dataset: A further reduction of the miniGHOSTS dataset containing 14 questions designed for rapid pre-screening. These questions are representative of typical problems in each subdataset, and typically cause struggle for Chat-GPT.
- Prompt Engineering: The process of crafting or modifying questions or instructions given to an AI model (such as an LLM) to improve the quality and accuracy of the model’s responses.
- MSC (Mathematics Subject Classification): A hierarchical alphanumeric classification system used to categorize mathematical research papers and publications across different fields of mathematics.
- Natural Language Mathematics: The use of ordinary language, as opposed to formal symbolic systems, to express and discuss mathematical concepts, problems, and proofs.
- Out-of-Distribution: Data that is significantly different from the data used to train a model, often used to test a model’s generalization capabilities.
- Error Codes: A set of codes used to classify various types of incorrect responses by ChatGPT. Used for analyzing model performance.
- Warning Codes: A set of codes used to classify non-essential or potential issues in ChatGPT’s response that do not necessarily result in an incorrect answer. Used for analyzing model performance.
- Ground Truth: The reference standard or established correct answer, often used as a basis for comparison to evaluate the accuracy of a model’s performance.
- Mathematical Reasoning: The ability to apply logical and analytical thinking to solve mathematical problems, including identifying patterns, creating proofs, and using concepts from various domains.
- Symbolic Integration: The method of finding an algebraic solution for the indefinite integral of a given function.
Reference
Frieder, S., Pinchetti, L., Griffiths, R. R., Salvatori, T., Lukasiewicz, T., Petersen, P., & Berner, J. (2024). Mathematical capabilities of chatgpt. Advances in neural information processing systems, 36.