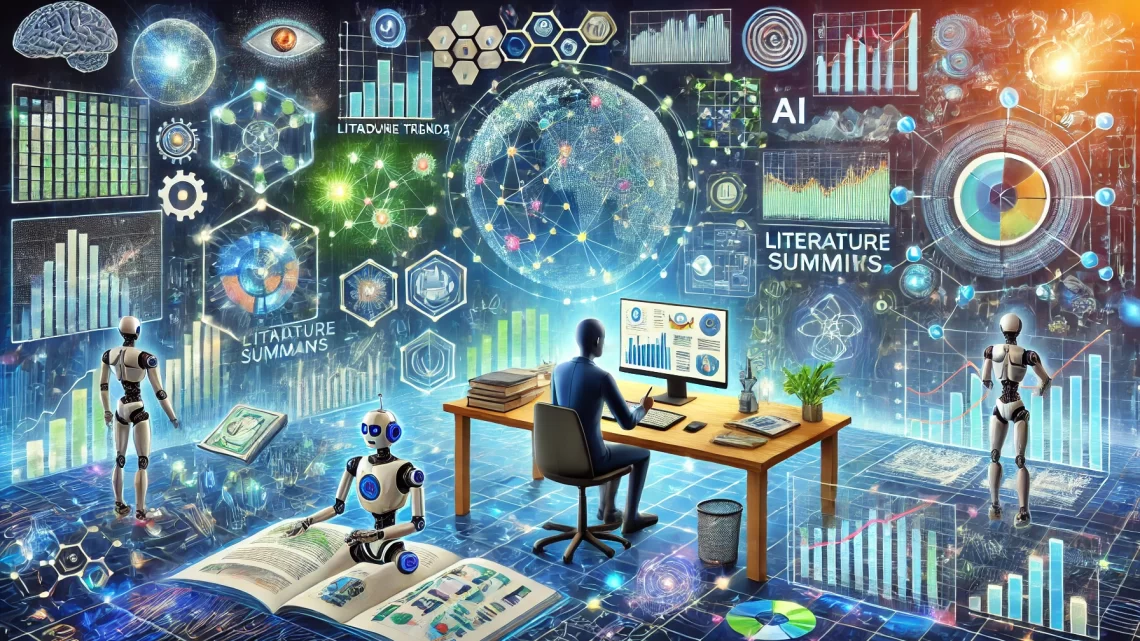
Step-by-Step Guide: Thinking in Parallel for Bioinformatics
January 10, 2025Parallel computing is essential in bioinformatics due to the large volumes of data and computationally intensive tasks. This guide provides a step-by-step approach to help you transition from serial to parallel programming, with practical examples and tools.
1. Understand Parallel Computing Basics
Parallel computing involves breaking down a problem into smaller tasks that can be executed simultaneously. Key concepts include:
- Data Parallelism: Split data into chunks and process each chunk independently.
- Task Parallelism: Execute different tasks concurrently.
- Embarrassingly Parallel Problems: Tasks that can be executed independently without communication (e.g., processing 12 million SNPs).
2. Identify Parallelizable Tasks
Look for tasks that can be divided into independent subtasks:
- Genomic Data Processing: Split by chromosome, gene, or sample.
- Sequence Alignment: Process multiple sequences or regions in parallel.
- Statistical Analysis: Run bootstrapping or permutation tests concurrently.
3. Use Simple Parallelization Techniques
Start with straightforward methods to parallelize tasks.
Example: Splitting Data and Running in Parallel
If you have a file with 12 million SNPs, split it into smaller files and process them in parallel.
Step 1: Split the File
split -l 3000000 snps.txt snp_chunk_
This splits snps.txt
into chunks of 3 million lines each.
Step 2: Process in Parallel
Use a loop to process each chunk in parallel:
for chunk in snp_chunk_*; do process_snps.sh "$chunk" & done wait
&
runs each task in the background.wait
ensures all tasks complete before proceeding.
4. Use GNU Parallel for Automation
GNU Parallel is a powerful tool for running jobs in parallel.
Install GNU Parallel
sudo apt-get install parallel
Example: Process SNPs in Parallel
cat snps.txt | parallel --jobs 4 process_snps.sh {}
--jobs 4
: Run 4 jobs in parallel.{}
: Placeholder for each input line.
5. Parallelize in Python
Use Python’s multiprocessing
module for parallel execution.
Example: Parallel Processing of SNPs
from multiprocessing import Pool def process_snps(snp_chunk): # Your processing logic here return processed_data if __name__ == "__main__": snp_chunks = [chunk1, chunk2, chunk3, chunk4] # List of SNP chunks with Pool(4) as p: # Use 4 processes results = p.map(process_snps, snp_chunks)
6. Parallelize in R
Use the parallel
package in R for parallel computing.
Example: Parallel Bootstrapping
library(parallel) # Define a function for bootstrapping bootstrap <- function(i) { sample_data <- sample(original_data, replace = TRUE) return(mean(sample_data)) } # Run in parallel num_cores <- detectCores() - 1 # Use all but one core results <- mclapply(1:1000, bootstrap, mc.cores = num_cores)
7. Use Cluster Computing
For large-scale tasks, use cluster computing frameworks like:
- Hadoop: For distributed data processing.
- Spark: For in-memory distributed computing.
Example: Running a Hadoop Job
hadoop jar hadoop-streaming.jar \ -input input_data \ -output output_data \ -mapper mapper.py \ -reducer reducer.py
8. Optimize Parallel Performance
- Load Balancing: Ensure tasks are evenly distributed across processors.
- Minimize Communication Overhead: Reduce data transfer between tasks.
- Profile and Debug: Use profiling tools to identify bottlenecks.
9. Learn from Examples
Study parallel implementations in bioinformatics tools:
- BWA: Parallelizes sequence alignment.
- GATK: Uses parallel processing for variant calling.
- DESeq2: Parallelizes statistical analysis in R.
10. Practice and Experiment
- Start Small: Parallelize simple tasks first.
- Experiment: Try different parallelization techniques and tools.
- Iterate: Refine your approach based on performance and results.
By following these steps, you can effectively transition to parallel programming in bioinformatics, improving the efficiency and scalability of your analyses.