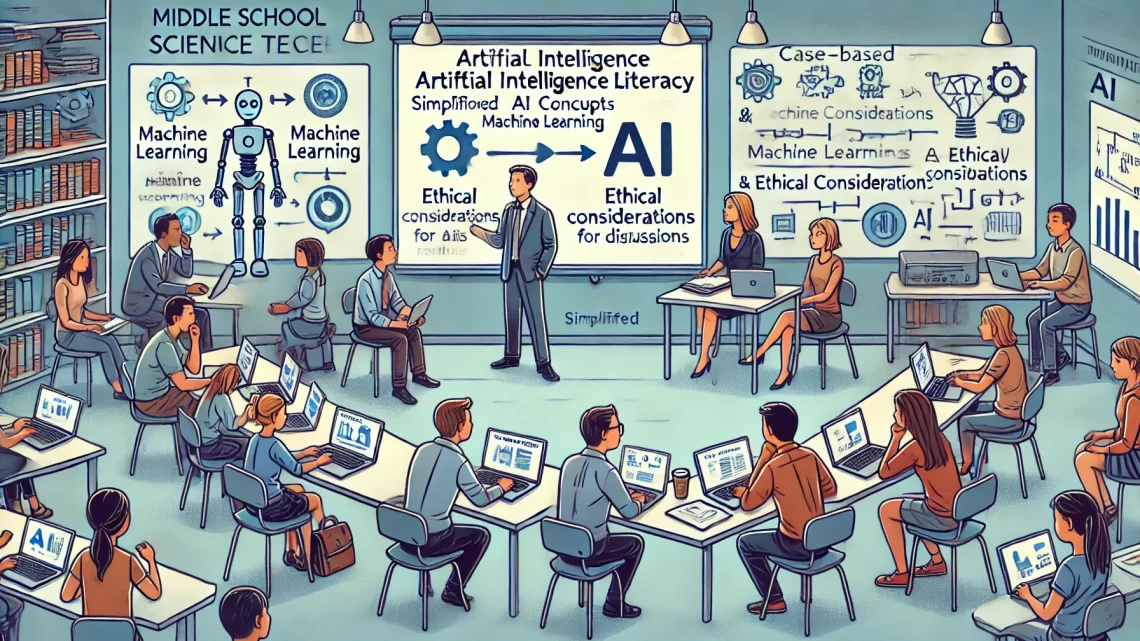
AI in Biomedical Literature Search
December 19, 2024Navigating Biomedical Literature: The Future of Search Tools and AI Integration
Biomedical literature serves as the foundation of medical knowledge, guiding clinical decisions, advancing research, and shaping healthcare policies. With the growing volume of published articles—over 36 million in PubMed alone—searching for relevant information has become a significant challenge for healthcare professionals and researchers alike. While PubMed remains the go-to tool for many, its reliance on keyword-based searches can limit its effectiveness for specialized queries. This blog explores the evolution of literature search tools, categorizing them by specific needs and highlighting the growing role of artificial intelligence (AI), particularly large language models (LLMs), in transforming how we search for and interact with biomedical literature.
The Limitations of Traditional Keyword-Based Searches
PubMed, though indispensable, processes keyword-based queries and returns raw article lists without additional analysis. While it’s an excellent starting point for general biomedical topics, it can be overwhelming for researchers who need to dive deeper into specific areas, such as COVID-19-related studies or rare genetic variants. A typical PubMed search retrieves hundreds or even thousands of articles, but fewer than 20% of those results are ever reviewed. This issue is compounded by the increasing volume of biomedical publications, with PubMed adding over 1 million articles annually.
Traditional keyword searches often fall short for complex queries or specialized research, where precision and context are essential. Therefore, new tools and strategies are emerging to complement PubMed and address these limitations.
Specialized Search Tools for Targeted Needs
As the need for more precise literature searches grows, specialized web-based tools have been developed to meet different search needs. These tools fall into five primary categories:
1. Evidence-Based Medicine (EBM)
EBM tools focus on identifying high-quality clinical evidence. These tools are essential for healthcare professionals and researchers involved in clinical decision-making, as they prioritize systematic reviews and randomized controlled trials (RCTs). EBM search engines such as PubMed Clinical Queries, Cochrane Library, and Trip Database are designed to handle both PICO (Population, Intervention, Comparison, Outcome) and natural language clinical questions. The goal is to streamline evidence synthesis, ranking higher-quality evidence above case reports or less rigorous studies. For efficient results, users should formulate clinical questions using the PICO framework and select tools that prioritize evidence quality.
2. Precision Medicine and Genomics
Precision medicine relies on understanding the genetic and molecular underpinnings of diseases, making access to accurate genomic information crucial. Tools like LitVar, DigSee, and OncoSearch specialize in retrieving and organizing genomic data, particularly for linking genes, variants, and diseases. These tools face challenges, such as handling the synonymous nature of genetic variants, but they help overcome this by normalizing variant synonyms across various publications. When researching genomic variants, starting with curated databases like UniProt and ClinVar is recommended, followed by specialized search engines for more current findings.
3. Semantic Search
Unlike traditional keyword searches, semantic search focuses on finding related textual units, not just exact keyword matches. This approach is particularly useful when dealing with natural language queries, where synonyms or context variations are common. Tools like LitSense, askMEDLINE, and BioMed Explorer use deep learning techniques to retrieve relevant sentences or question-answer pairs. These tools are valuable for researchers or clinicians who need precise, context-aware answers, as they can filter results based on meaning rather than keywords alone.
4. Literature Recommendation
Literature recommendation tools help users discover relevant articles related to their research topics. These tools typically function in two ways: topic-based systems curate articles around a specific research area, such as LitCovid for COVID-19, while article-based systems recommend papers related to a list of previously selected articles, like LitSuggest. Additionally, tools like Connected Papers and Litmaps offer interactive visualizations of article relationships, allowing researchers to explore the literature through citation networks and graphs.
5. Literature Mining
Literature mining tools use natural language processing (NLP) techniques to extract biomedical concepts, such as genes, diseases, and biological processes, and map their relationships to uncover new insights. Tools like PubTator, FACTA+, and Semantic MEDLINE employ named entity recognition (NER) and relation extraction (RE) to identify and visualize associations between concepts in biomedical literature. These tools are especially useful for identifying novel connections that may lead to new research directions or discoveries.
The Role of Large Language Models (LLMs)
AI technologies, particularly large language models (LLMs) like ChatGPT, are revolutionizing how we search for and synthesize biomedical literature. LLMs are already proving their potential in several areas:
- Evidence Synthesis: LLMs can assist in generating Boolean queries, synthesizing evidence, and summarizing large volumes of literature quickly. This can significantly reduce the time spent reviewing articles and help researchers focus on critical insights.
- Precision Medicine: By interacting with specialized databases and summarizing their contents, LLMs can directly answer queries related to genomic data, disease associations, and clinical outcomes, bridging the gap between complex datasets and accessible information.
- Semantic Search: LLMs excel in semantic search, providing relevant answers to natural language queries. They can process documents returned from traditional search engines and generate direct answers to questions, making literature searches more intuitive and efficient.
- Literature Mining: LLMs can help interpret knowledge graphs and suggest potential new associations between biomedical concepts, improving the discovery of novel insights.
However, LLMs come with a caveat: the potential for errors. Answers generated by LLMs must be verified for accuracy, as these models may make mistakes, especially when processing complex or specialized topics.
How to use AI for literature search, based on the provided sources:
Step | Action |
---|---|
1. Identify Your Information Need | Determine the specific type of information you are seeking. Categorize into areas like Evidence-Based Medicine (EBM), precision medicine and genomics, semantic search, literature recommendation, and literature mining. |
2. Start with General-Purpose Search Engines | Begin with PubMed for general biomedical topics, citation searches, or systematic reviews. For full-text searches, use PubMed Central (PMC) or Europe PMC. |
3. Explore Specialized AI-Powered Search Tools | Move to AI-powered tools based on your specific need: – For EBM: PubMed Clinical Queries, Cochrane Library, Trip Database – For Precision Medicine: LitVar, variant2literature, DigSee, OncoSearch – For Semantic Search: LitSense, askMEDLINE, BioMed Explorer – For Literature Recommendation: LitCovid, LitSuggest, Connected Papers – For Literature Mining: PubTator, FACTA+, Semantic MEDLINE, SciSight |
4. Leverage Large Language Models (LLMs) | Use LLMs like ChatGPT to enhance the search: – Generate Boolean queries – Summarize articles – Extract PICO elements for EBM – Provide direct answers to natural language questions using tools like Scite or Elicit |
5. Evaluate and Verify Results | Be cautious of potential errors in LLM-generated answers. Cross-reference AI-generated information with reliable sources. |
6. Use Task-Oriented Search Interfaces | Look for specialized search interfaces tailored to your task. These are more effective than general search boxes. |
7. Incorporate AI into Your Workflow | Integrate AI-powered tools into your regular research workflow. Start with a clear question, use appropriate tools, and verify the information retrieved. |
This table summarizes the steps for using AI tools effectively in a literature search across various fields of biomedical research.
Best Practices for Efficient Literature Search
To maximize the effectiveness of biomedical literature searches, here are some best practices:
- Start with PubMed: For general biomedical topics and a broad overview, PubMed is an excellent starting point. For full-text articles, use PubMed Central (PMC).
- Formulate Focused Queries: Use the PICO format for clinical questions and leverage advanced search options to narrow down results.
- Explore Specialized Tools: Depending on your specific needs—whether it’s evidence-based medicine, genomics, or semantic search—choose the right tool for the job.
- Prioritize Quality: When conducting clinical research, ensure that you use tools that prioritize high-quality evidence, such as those designed for EBM.
- Stay Updated: The field of biomedical literature search is evolving rapidly. New tools and technologies are constantly being developed, so staying informed is key.
- Verify Results: Especially when using LLMs, always verify the findings and summaries generated by AI-powered tools.
Table : Web-based biomedical literature search tools.
Resource | Website | Brief Description |
---|---|---|
General-purpose search engines | ||
PubMed | https://pubmed.ncbi.nlm.nih.gov/ | General-purpose biomedical literature search engine. |
PubMed Central | https://www.ncbi.nlm.nih.gov/pmc/ | Supporting full-text search. |
Europe PMC | https://europepmc.org/ | Searching both abstracts and full-texts. |
Information assembly and synthesis for evidence-based medicine | ||
PubMed Clinical Queries | https://pubmed.ncbi.nlm.nih.gov/clinical/ | Searching clinical studies with various type and scope filters. |
Cochrane Library | https://www.cochranelibrary.com/ | Searching high-quality systematic reviews. |
Trip Database | https://www.tripdatabase.com/ | General EBM search engine. |
Information linking for precision medicine and genomics | ||
LitVar | https://www.ncbi.nlm.nih.gov/research/litvar | Searching relevant information for all synonyms to the given variant. |
Variant2literature | https://www.taigenomics.com/console/v2l | Searching information linking variants to literature. |
DigSee | http://210.107.182.61/geneSearch/ | Finding evidence sentences for the given gene, disease, biological processes triplet. |
OncoSearch | http://oncosearch.biopathway.org/ | Searching sentences that mention gene expression changes in cancers. |
Semantic search for similar sentences or question answers | ||
LitSense | https://www.ncbi.nlm.nih.gov/research/litsense/ | Searching relevant sentences to the given query. |
COVID-19 Challenges and Directions | https://challenges.apps.allenai.org/ | Searching COVID-19 challenges and future directions for the given topic. |
askMEDLINE | https://pubmedhh.nlm.nih.gov/ask/index.php | Answering the query question with documents or text snippets in literature. |
COVID-19 Research Explorer | https://covid19-research-explorer.appspot.com/biomedexplorer/ | Answering the original question and follow-up questions with text snippets in literature. |
BioMed Explorer | https://sites.research.google/biomedexplorer/ | AI-powered tool for literature exploration and analysis. |
Literature recommendation for specific topics or similar articles | ||
LitCovid | https://www.ncbi.nlm.nih.gov/research/coronavirus/ | Literature hubs for COVID-19. |
WHO COVID-19 Research Database | https://www.who.int/emergencies/diseases/novel-coronavirus-2019/globalresearch-on-novel-coronavirus-2019-ncov | WHO’s database for COVID-19 research. |
iSearch COVID-19 Portfolio | https://icite.od.nih.gov/covid19/search/ | Searching NIH COVID-19 portfolio. |
Corona Central | https://coronacentral.ai/ | AI-based tool for COVID-19-related literature recommendations. |
COVID-SEE | https://covid-see.com/search | Searching COVID-19-related literature and data. |
COVIDScholar | https://covidscholar.org/ | Searching COVID-19 literature for related articles. |
LitSuggest | https://www.ncbi.nlm.nih.gov/research/litsuggest/ | Scoring article candidates based on user-provided positive and negative articles. |
BioReader | https://services.healthtech.dtu.dk/service.php?BioReader-1.2 | Personalized literature recommendations based on topics of interest. |
Connected Papers | https://www.connectedpapers.com/ | Recommending relevant articles to one or more seed articles using the citation graph. |
Litmaps | https://www.litmaps.com/ | Visualizing and exploring the connections between academic papers. |
Literature mining for knowledge discovery | ||
PubTator | https://www.ncbi.nlm.nih.gov/research/pubtator/ | Highlighting biomedical concepts in the retrieved documents. |
Anne O’Tate | http://arrowsmith.psych.uic.edu/cgi-bin/arrowsmith_uic/AnneOTate.cgi | Ranking the extracted concepts from the search results. |
FACTA+ | http://www.nactem.ac.uk/facta/index.html | Finding directly and indirectly associated concepts to the given concept. |
Semantic MEDLINE | https://ii.nlm.nih.gov/SemMed/semmed.html | Displaying graphs of biomedical concepts and their relations extracted from the retrieved documents. |
SciSight | https://scisight.apps.allenai.org/ | Exploring biomedical literature and visualizing relationships. |
PubMedKB | https://www.pubmedkb.cc/ | Knowledge base for biomedical literature. |
LION LBD | https://lbd.lionproject.net/ | Exploring biomedical literature with knowledge graph visualization. |
(Experimental) literature search systems augmented by LLMs | ||
Scite | https://hippocratic-medical-questions.herokuapp.com/ | Finding relevant articles to users’ questions and using LLMs to answer the questions with retrieved articles. |
Elicit | https://elicit.org/ | AI-powered research tool for literature and data analysis. |
Consensus | https://consensus.app/ | Answering complex research questions with data-driven insights. |
This table provides a concise overview of various biomedical literature search tools, their websites, and brief descriptions of their functions.
The Future of Biomedical Literature Search
The future of biomedical literature search is bright, with AI playing an increasingly prominent role. We can expect to see integrated systems that combine the best features of traditional search engines and newer AI-driven tools. Task-oriented interfaces, better ranking algorithms, and more interactive result presentation formats, such as knowledge graphs and LLM-generated summaries, will make it easier for researchers to find and interpret relevant information.
Conclusion
As biomedical research continues to grow at an unprecedented rate, efficient literature search tools are more important than ever. While PubMed remains a cornerstone of biomedical research, specialized tools and AI technologies like LLMs are reshaping how we search for, retrieve, and analyze information. By understanding the limitations of traditional methods and embracing new tools and AI advancements, healthcare professionals and researchers can navigate the ever-expanding landscape of biomedical literature more efficiently and effectively.
Table outlining the Timeline of Main Events & Developments related to biomedical literature search tools:
Year | Event/Development |
---|---|
Pre-2017 | PubMed primarily uses a recency-based ranking system, displaying articles in reverse chronological order. Keyword searches return lists of raw articles without further analysis. |
2007 | Manual curation of genomic databases is becoming insufficient due to the volume of data (Baumgartner et al). |
2009 | A study of PubMed search logs begins to analyze user behavior (Islamaj Dogan et al). |
2011 | Lu surveys web tools for searching biomedical literature. |
2013 | DigSee is developed as a disease gene search engine (Kim et al). PubTator is introduced (Wei et al). tmVar is released (Wei et al). |
2014 | OncoSearch is released as a cancer gene search engine (Lee et al). Keepanasseril conducts an environmental scan of PubMed alternatives. |
2015 | Europe PMC is released (Europe PMC). The Precision Medicine Initiative is introduced (Collins, Varmus). |
2016 | Wildgaard and Lund compare third-party PubMed/Medline tools. Jacome et al propose a biomedical search engine framework. A study is published describing the BioCreative V chemical-disease relation (CDR) task (Wei et al). Marshall et al evaluate the RobotReviewer for automatically assessing bias. |
2017 | PubMed introduces the “Best Match” AI-based relevance ranking model. |
2018 | LitVar is released (Allot et al). Fiorini et al report on how user intelligence is improving PubMed. Nye et al introduce a corpus to support language processing for medical literature. |
2019 | LitSense is released (Allot et al). BioReader is released (Simon et al). LitVar is updated (Lin et al). Marshall and Wallace write a practical guide for machine learning in research synthesis. Pyysalo et al release LION LBD. Cochrane Handbook for Systematic Reviews of Interventions published (Higgins et al). |
2020 | LitCovid is launched (Chen et al). The Coronavirus pandemic rapidly increases research output and presents challenges for traditional search methods. Hope et al present SciSight. Callaway et al present powerful charts on the Coronavirus pandemic in Nature. |
2021 | LitCovid is updated and becomes a key resource for COVID-19 research (Chen et al). LitSuggest is released (Allot et al). Anne O’Tate is released (Smalheiser et al). Lever and Altman introduce CoronaCentral. Verspoor et al introduce COVID-SEE. UniProt is updated (UniProt C). Li et al report on the surge in publications on the COVID-19 pandemic. |
2022 | Jin et al survey biomedical question answering. Yan et al release PhenoRerank. Lahav et al present a search engine for scientific challenges and directions. Li et al release PubMedKB. Tsuruoka et al find indirect associations between biomedical concepts. ChatGPT and other generative large language models (LLMs) emerge, demonstrating substantial performance in NLP tasks and the beginnings of influence on literature search. |
2023 | LitVar is updated to version 2.0 (Allot et al). Allot et al update LitCovid. Dagdelen et al launch COVIDScholar. Suster et al evaluate classifiers for medical evidence assessment and automate the quality assessment of medical evidence. Jin et al. publish an article matching patients to clinical trials with LLMs. Shaib et al work to summarize medical evidence using LLMs. Wang et al explore the capacity of ChatGPT to write boolean queries. Europe PMC contains 42.7 million abstracts and 9.0 million full-text articles. The study presented in this document is published, surveying specialized literature search tools and the impact of AI, including LLMs. |
Future | Development of LLM-powered tools to assist with literature screening and summarization for systematic reviews. Integration of LLMs to enhance PICO element extraction. Use of LLMs to alleviate access difficulties to information in databases. Exploration of using LLMs to explain literature recommendations. Use of LLMs to interpret knowledge graphs. Development of tools to automatically triage user information needs and provide the right tools. Future search interfaces to handle semi-structured information or non-text modalities. Integration of transparent and interpretable ranking algorithms in search engines. Future literature search engines to include LLM-generated overviews of returned articles. |
This timeline includes key milestones in the development of biomedical literature search engines and tools, particularly with the integration of AI and LLM technologies.
Frequently Asked Questions on Biomedical Literature Search Tools
What are the main challenges in biomedical literature search that necessitate specialized tools beyond PubMed?
While PubMed is a valuable general-purpose tool, several challenges hinder its effectiveness for specialized needs. These include the sheer volume of publications (over 36 million articles, growing annually), the difficulty of formulating complex queries using keywords, limitations of keyword-based searches when seeking meaning or concepts instead of exact text matches, the lack of direct access to full-text articles, and the inability to effectively prioritize search results by evidence quality. These limitations often lead to clinicians and researchers missing crucial information, making specialized tools essential for more targeted and efficient searches.
How do Evidence-Based Medicine (EBM) search tools improve upon traditional literature search for clinical questions?
EBM search tools are designed to help clinicians find high-quality clinical evidence. They accomplish this by allowing users to structure queries based on PICO elements (Population, Intervention, Comparison, and Outcome), which helps specify the search intent, thus returning studies directly related to the clinical question. These tools often prioritize results based on the quality of evidence, such as highlighting systematic reviews and randomized controlled trials over case reports, which is important because evidence from different sources varies in reliability. Some EBM tools also use predefined filters for specific types of studies, further refining searches.
Why are specialized tools needed for precision medicine and genomics literature searches and what are some core features of these tools?
Precision medicine and genomics rely on understanding genomic variants, and these can be described in many ways (synonyms). Keyword-based searches are inadequate for finding all mentions of a variant or gene because they cannot account for synonymous representations. Specialized tools such as LitVar use text mining to normalize variant synonyms, and these tools often index both abstracts and full texts of articles. Some tools also link genes, diseases, and biological processes. Some, like OncoSearch, specialize in showing gene expression changes in cancers. These tools enhance precision by extracting variant information directly from literature, supplementing curated databases.
What is semantic search, and how does it differ from traditional keyword-based search in the context of biomedical literature?
Semantic search goes beyond exact keyword matches, focusing on the meaning of the search terms. It locates text units (e.g. sentences) that are semantically related to the query, even if they do not contain the exact words used in the query. Traditional keyword-based searches return articles based on term overlap, potentially missing articles that use synonyms or related concepts. For example, a semantic search for “renal” would also retrieve articles using “kidney” because the two terms share similar meanings. This approach enables the retrieval of more complete and nuanced information. Some tools focus on similar sentence searches, while others may address question answering, such as askMEDLINE, or provide broader biomedical text-answering, such as BioMed Explorer.
How do literature recommendation systems help researchers navigate the vast amount of biomedical literature and what are the two main types of these systems?
Literature recommendation systems assist researchers in finding related publications without relying on complicated keyword-based queries. There are two main types of literature recommendation systems. Topic-based systems like LitCovid, are curated databases or hubs for specific research topics, which simplify finding literature within that area. Article-based systems like LitSuggest and BioReader, suggest articles based on the similarity to one or more seed articles. Some tools such as Connected Papers and Litmaps use visual representations of the relationships between papers through citation graphs. These systems expand researchers’ ability to find relevant work and explore related fields more effectively.
What is literature mining, and how does it aid in knowledge discovery within biomedical publications?
Literature mining uses natural language processing (NLP) techniques to extract biomedical concepts (e.g., genes, diseases) and their relationships from the literature. This involves tasks like named entity recognition (NER) and relation extraction (RE), helping to create knowledge graphs. These tools reveal hidden associations between concepts and support hypothesis generation. For instance, the literature mining tools can help a researcher find all articles about gene x, disease y, and relation z to find hidden associations. Systems like PubTator, FACTA+, Semantic MEDLINE, and LION LBD provide interactive visualizations to explore knowledge. These approaches accelerate literature-based discovery (LBD) by predicting previously unknown relationships.
What is the potential role of Large Language Models (LLMs) like ChatGPT in revolutionizing biomedical literature search?
LLMs have the potential to transform biomedical literature search in multiple ways. In Evidence-Based Medicine (EBM), LLMs can assist in formulating better Boolean queries, summarize and synthesize articles for systematic reviews, and even improve PICO element extraction. In precision medicine, they can provide access to information stored in specialized databases and directly summarize their contents. LLMs can also answer biomedical questions using natural language queries. In terms of literature recommendation, LLMs may help explain why articles are being suggested. Although these tools are promising, LLM answers can be susceptible to errors and need to be carefully verified.
How can researchers choose the best literature search tools for their specific information needs?
The best approach involves considering what kind of information needs to be found. If users are looking for high-quality clinical evidence, an EBM-focused tool using PICO elements is preferable. If they need genomic information, a tool focused on variant synonyms should be selected. For finding semantically related text, they might choose a semantic search engine, or for exploring related articles, a literature recommendation system is best. For novel knowledge discovery, a literature mining tool would help. Researchers should be aware of the various specialized tools available and choose the tool that best addresses their specific search purpose.
Glossary of Key Terms
- Artificial Intelligence (AI): The capability of a computer or a robot controlled by a computer to do tasks that are usually done by humans because they require human intelligence and discernment.
- Biomedical Literature Search: The process of retrieving scientific articles related to biomedicine to satisfy specific information needs.
- Boolean Operators: Words (e.g., AND, OR, NOT) used to combine or exclude keywords in a search query to make it more specific.
- Evidence-Based Medicine (EBM): A medical practice that relies on scientific evidence derived from high-quality clinical studies.
- Large Language Models (LLMs): Artificial intelligence algorithms, such as ChatGPT, designed to process and generate natural language text.
- Literature Mining: Using natural language processing techniques to extract concepts, relations, and knowledge from the literature to uncover novel insights.
- MeSH Terms: A controlled vocabulary of standardized terms used to index articles in PubMed and related databases, making searches more accurate and comprehensive.
- Named Entity Recognition (NER): An NLP technique that identifies and classifies named entities, such as genes or diseases, in text.
- Precision Medicine (PM): A medical approach that tailors treatment to individual patient characteristics, including genes, lifestyle, and environment.
- PubMed: A widely used, free, biomedical literature search engine maintained by the US National Library of Medicine (NLM).
- PubMed Central (PMC): A free archive of full-text articles available on PubMed.
- PICO Elements: Components of a structured clinical question in EBM: Population, Intervention, Comparison, and Outcome.
- Ranking Algorithm: An algorithm used by search engines to determine the order in which search results are presented, based on relevance to the query.
- Relation Extraction (RE): An NLP technique that identifies and classifies relationships between extracted concepts in a text.
- Retrieval Augmentation: A method used by LLMs to generate answers by referencing relevant documents or text snippets in the literature.
- Semantic Search: A search technique that aims to return results based on the meaning and context of a query instead of just keyword matches.
- Systematic Review: A comprehensive and unbiased analysis of all relevant research studies to address a specific question or topic.
- Text Mining: A process of using natural language processing (NLP) techniques to analyze and extract information from unstructured text.
Biomedical Literature Search in the Age of AI: A Study Guide
Quiz
Instructions: Answer each question in 2-3 sentences.
- What is the primary challenge that motivates the need for specialized literature search tools beyond PubMed?
- Describe the function of “Best Match” in PubMed and why it was introduced.
- What are the PICO elements in the context of evidence-based medicine, and why are they important for search queries?
- How do search engines for precision medicine and genomics address the issue of multiple representations for the same variant?
- Explain the difference between keyword-based search and semantic search.
- What are the two main types of literature recommendation systems discussed in the article, and how do they differ?
- Describe the process of literature mining and its goal in biomedical research.
- How can large language models (LLMs) potentially improve the process of evidence synthesis in evidence-based medicine?
- What are some of the drawbacks associated with using LLMs for generating answers to biomedical questions?
- Why is it important for future literature search engines to incorporate interpretable ranking algorithms?
Answer Key
- The exponential growth of biomedical literature makes it difficult to identify relevant information using general-purpose search engines like PubMed. This motivates a need for tools that address specific information needs by going beyond keyword-based searching.
- “Best Match” is an AI-based ranking model in PubMed that prioritizes articles based on relevance rather than recency. It was introduced to improve the user experience by ensuring that the most significant articles appear at the top of the search results.
- PICO elements (Population, Intervention, Comparison, and Outcome) are components of a structured clinical question used in evidence-based medicine. They help to precisely define the information need and return more focused search results.
- Search engines for precision medicine and genomics address synonymy by using text mining tools to normalize variant names and convert them to a standardized format. This allows the retrieval of all articles that mention the variant and its various synonyms.
- Keyword-based search engines look for exact matches to the input query, while semantic search locates texts that are related to the query’s meaning. Semantic search can retrieve texts that do not have any word overlap but are semantically similar.
- Topic-based recommendation systems are curated databases for specific topics, like the COVID-19 pandemic, while article-based recommenders generate a list of articles similar to a set of “seed” articles. They differ in how the search is initiated, by topic or by a set of articles.
- Literature mining uses natural language processing techniques to extract biomedical concepts, such as genes and diseases, and their relations from research papers. The goal is to uncover novel insights and knowledge from large amounts of literature.
- LLMs can accelerate evidence synthesis by suggesting Boolean queries to assist literature screening during systematic reviews and by summarizing and synthesizing the resulting articles. They can also help in extracting PICO elements.
- LLM-generated answers to biomedical questions can be susceptible to errors, bias, and hallucination, which must be carefully verified before being used. This is largely due to the model’s reliance on patterns in data, which does not always translate to factually correct output.
- Transparent and interpretable ranking algorithms are important for future literature search engines to allow users to understand the rationale behind why certain articles are prioritized. This can build user trust and assist in the analysis of search results.
Essay Questions
Instructions: Answer each of the following questions in essay format, drawing on the material from the provided source.
- Discuss the evolution of PubMed as a literature search engine, including its limitations and the advancements made to address those limitations.
- Explain the five main categories of specialized literature search tools presented in the article, and describe how they meet specific information needs.
- Analyze the role of literature mining in the advancement of biomedical knowledge and explore the techniques and tools used for this purpose.
- Evaluate the potential impact of large language models like ChatGPT on the field of biomedical literature search, including both opportunities and challenges.
- Consider the future of biomedical literature search engines, discussing the needed improvements in search interfaces, ranking algorithms, and result display methods.
Reference
Jin, Q., Leaman, R., & Lu, Z. (2024). PubMed and beyond: biomedical literature search in the age of artificial intelligence. EBioMedicine, 100.