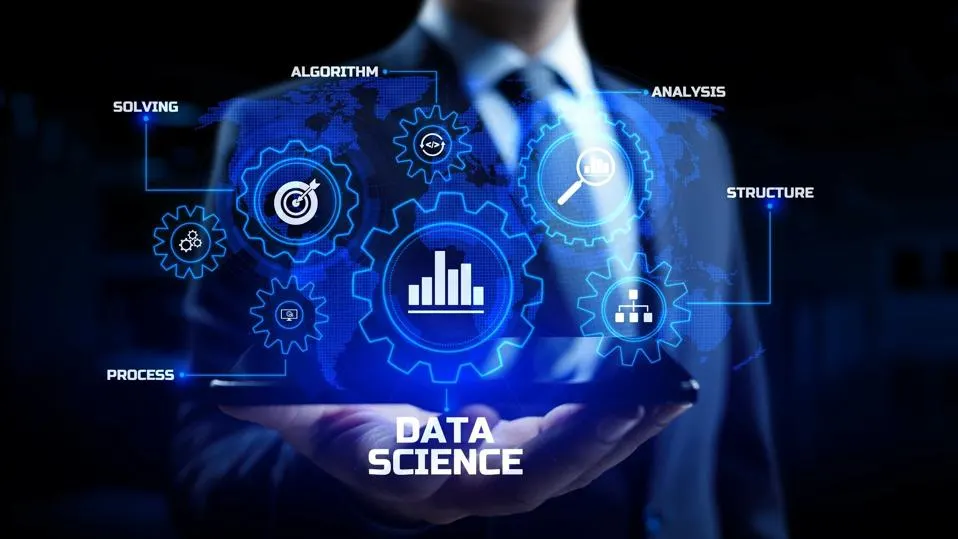
Predicting the Future: Exploring the Wonders of Predictive Analytics
December 6, 2023I. Introduction
Predictive analytics plays a pivotal role in bioinformatics and omics, offering a powerful toolset to unravel the complexities of biological data. In this section, we provide a brief overview of predictive analytics within the context of bioinformatics and omics, highlighting the significance of predicting future trends and patterns in biological data.
1. Predictive Analytics in Bioinformatics:
- Definition: Predictive analytics involves the use of statistical algorithms and machine learning models to analyze historical data and make predictions about future events or trends.
- Application in Bioinformatics: In bioinformatics, predictive analytics leverages advanced computational methods to analyze vast datasets, such as those generated through genomics, transcriptomics, proteomics, and metabolomics. By deciphering complex patterns within biological data, predictive analytics enables researchers to anticipate future outcomes and trends.
2. Omics Data and Predictive Analytics:
- Omics Data Types:
- Bioinformatics deals with various omics data, including genomics (DNA sequencing), transcriptomics (gene expression), proteomics (protein expression), and metabolomics (metabolite profiling). These data types provide a comprehensive view of biological systems.
- Challenges of Omics Data:
- Omics data is often high-dimensional and complex, presenting challenges for traditional analytical approaches. Predictive analytics offers a solution by harnessing the power of machine learning to extract meaningful insights from these intricate datasets.
3. Significance of Predicting Future Trends:
- Precision Medicine: Predictive analytics contributes to the advancement of precision medicine by identifying biomarkers and predicting patient responses to treatments. This facilitates personalized and targeted healthcare interventions.
- Disease Diagnosis and Prognosis: Predicting future trends in biological data aids in early disease diagnosis and prognosis. Machine learning models can analyze molecular profiles to identify patterns indicative of specific diseases and predict their progression.
- Biological Pathway Analysis: Understanding and predicting how biological pathways function is critical in deciphering the molecular mechanisms underlying diseases. Predictive analytics assists in modeling and predicting the interactions within these pathways.
- Drug Discovery: Predictive analytics accelerates drug discovery by forecasting the effectiveness and potential side effects of new compounds. This aids in prioritizing candidates for further testing, reducing costs and time in the drug development process.
- Optimizing Experimental Design: Researchers can use predictive analytics to optimize experimental design by predicting the likely outcomes of different interventions. This ensures efficient allocation of resources in laboratory experiments.
4. Transformative Impact on Research:
- Data-Driven Discoveries: Predictive analytics transforms biological research by enabling data-driven discoveries. Researchers can uncover hidden patterns and relationships within omics data, leading to novel hypotheses and insights.
- Iterative Learning Process: The iterative nature of predictive analytics allows researchers to continuously refine models based on new data. This adaptive approach enhances the accuracy and robustness of predictions over time.
In the intricate realm of bioinformatics and omics, predictive analytics emerges as a catalyst for breakthroughs. By harnessing the power of machine learning and statistical modeling, researchers gain the ability to foresee future trends and patterns in biological data, paving the way for transformative advancements in personalized medicine, disease understanding, and drug discovery. The subsequent sections will delve deeper into key concepts and practical applications within this dynamic intersection of predictive analytics and bioinformatics.
II. Understanding Predictive Analytics
A. Definition and Basics
1. Defining Predictive Analytics and Its Applications in Bioinformatics:
- Predictive Analytics Definition: Predictive analytics involves the use of statistical algorithms and machine learning techniques to analyze historical data and make predictions about future events or trends.
- Applications in Bioinformatics:
- In bioinformatics, predictive analytics finds applications in predicting biological outcomes, identifying biomarkers, understanding disease mechanisms, and optimizing experimental design. It enables researchers to extract actionable insights from complex omics data.
B. Predictive Modeling
2. Introduction to Predictive Modeling Techniques:
- Predictive Modeling Overview:
- Predictive modeling is the process of creating and validating a model to make predictions about future outcomes based on historical data. It involves the selection of appropriate variables and algorithms to generate accurate predictions.
- Applications in Bioinformatics:
- In bioinformatics, predictive modeling is applied to various omics data types for tasks such as disease classification, patient stratification, and drug response prediction. It aids in uncovering patterns and relationships within biological datasets.
3. Overview of Machine Learning Algorithms for Prediction:
- Supervised Learning:
- Definition: In supervised learning, models are trained on labeled datasets, where the input data is paired with corresponding output labels.
- Applications: Supervised learning is widely used for tasks like disease classification, drug response prediction, and biomarker identification.
- Unsupervised Learning:
- Definition: Unsupervised learning involves training models on unlabeled datasets, where the algorithm identifies patterns and relationships without explicit guidance.
- Applications: Unsupervised learning is applied to tasks such as clustering similar patient profiles based on omics data or identifying subtypes of diseases.
- Regression Analysis:
- Definition: Regression analysis predicts a continuous outcome variable based on one or more predictor variables.
- Applications: Regression models are utilized to predict quantitative outcomes, such as predicting gene expression levels or protein concentrations.
- Deep Learning:
- Definition: Deep learning involves neural networks with multiple layers (deep neural networks) and is particularly effective in handling complex, high-dimensional data.
- Applications: Deep learning models, such as convolutional neural networks (CNNs) and recurrent neural networks (RNNs), excel in tasks like image analysis and sequence prediction in genomics.
- Ensemble Methods:
- Definition: Ensemble methods combine the predictions of multiple models to improve overall accuracy and robustness.
- Applications: Ensemble methods, like random forests and gradient boosting, enhance prediction performance and are commonly used in bioinformatics tasks.
Understanding predictive analytics in the context of bioinformatics involves grasping the fundamentals of predictive modeling. This includes recognizing the diverse applications of machine learning algorithms in tasks ranging from disease classification to drug response prediction. The subsequent sections will delve deeper into practical implementations and considerations for successful predictive analytics in bioinformatics.
III. Predictive Analytics in Bioinformatics
A. Role in Omics Fields
1. Application of Predictive Analytics in Genomics, Proteomics, Transcriptomics, etc.:
- Genomics:
- Predictive analytics in genomics involves predicting genetic variations, identifying potential disease-associated mutations, and understanding the impact of genetic changes on phenotypes.
- Proteomics:
- In proteomics, predictive analytics is applied to predict protein structures, interactions, and functions. It aids in understanding the role of specific proteins in biological processes and diseases.
- Transcriptomics:
- Predictive analytics in transcriptomics focuses on predicting gene expression levels, alternative splicing patterns, and regulatory interactions. This contributes to understanding cellular responses and signaling pathways.
- Metabolomics:
- In metabolomics, predictive analytics is used to predict metabolic pathways, identify biomarkers, and understand the metabolic fingerprint associated with specific conditions or treatments.
- Epigenomics:
- Predictive analytics plays a role in epigenomics by predicting epigenetic modifications and their influence on gene expression. This contributes to unraveling the epigenetic regulation of cellular processes.
B. Real-World Examples
2. Case Studies Demonstrating Successful Predictive Analytics in Bioinformatics:
- a. Disease Classification:
- Example: Predictive models applied to gene expression data successfully classify cancer types, allowing for more precise diagnosis and personalized treatment strategies.
- b. Drug Response Prediction:
- Example: Predictive analytics models are used to predict how individual patients will respond to specific drugs, aiding in the selection of targeted and effective treatment plans.
- c. Biomarker Identification:
- Example: Predictive modeling identifies potential biomarkers associated with disease progression, facilitating early diagnosis and providing insights into underlying molecular mechanisms.
- d. Protein Structure Prediction:
- Example: Deep learning models applied to protein sequences contribute to accurate protein structure prediction, accelerating drug discovery and understanding protein functions.
- e. Patient Outcome Prediction:
- Example: Predictive analytics models analyze patient data to predict clinical outcomes, enabling healthcare professionals to make informed decisions about treatment strategies and interventions.
3. Impact on Research and Decision-Making:
- Accelerating Discoveries:
- Predictive analytics accelerates the pace of discoveries in bioinformatics by uncovering hidden patterns and relationships within omics data, leading to novel hypotheses and insights.
- Precision Medicine Advances:
- The application of predictive analytics in bioinformatics contributes to the realization of precision medicine, where treatments are tailored to individual patients based on their genetic makeup and omics profiles.
- Informed Decision-Making:
- Researchers and healthcare professionals make more informed decisions by leveraging predictive analytics. This includes selecting optimal treatment strategies, prioritizing drug candidates, and designing targeted experiments.
- Optimizing Resource Allocation:
- Predictive analytics assists in optimizing the allocation of resources by guiding researchers to focus on experiments and investigations that are most likely to yield meaningful results.
- Enhancing Patient Care:
- The use of predictive analytics in bioinformatics has a direct impact on patient care by enabling more accurate diagnostics, personalized treatment plans, and improved prognostic assessments.
Predictive analytics in bioinformatics transforms the way researchers and healthcare professionals leverage omics data. Real-world examples illustrate the successful application of predictive models in diverse areas, from disease classification to drug response prediction. The impact on research and decision-making underscores the transformative potential of predictive analytics in advancing our understanding of biological systems and improving healthcare outcomes.
IV. Key Predictive Analytics Techniques
A. Data Preprocessing
1. Cleaning and Preparing Biological Data for Predictive Modeling:
- Data Cleaning:
- Addressing missing values, handling outliers, and resolving inconsistencies in biological data to ensure its quality and reliability.
- Normalization and Scaling:
- Standardizing the scale of different features to ensure that no single feature disproportionately influences the predictive model.
- Data Integration:
- Integrating data from multiple omics sources to create a comprehensive dataset for predictive modeling.
- Handling Imbalanced Data:
- Addressing imbalances in the distribution of classes in datasets, especially in scenarios like disease prediction where positive and negative instances may be unevenly distributed.
B. Feature Selection
2. Identifying Relevant Features for Accurate Predictions:
- Filter Methods:
- Statistical techniques (e.g., correlation, chi-squared) to identify features that exhibit strong relationships with the target variable.
- Wrapper Methods:
- Evaluating subsets of features by training and testing models iteratively to identify the most informative set.
- Embedded Methods:
- Incorporating feature selection as part of the model training process, such as regularization techniques in linear models.
- Domain Knowledge Integration:
- Leveraging domain knowledge to guide the selection of biologically relevant features, enhancing the interpretability and biological significance of the model.
C. Model Selection
3. Choosing Appropriate Machine Learning Models for Different Bioinformatics Scenarios:
- Supervised Learning Models:
- a. Decision Trees and Random Forests: Suitable for classification tasks, especially when dealing with complex interactions and nonlinear relationships in the data.
- b. Support Vector Machines (SVM): Effective for binary and multiclass classification tasks, particularly when the data is not linearly separable.
- c. Logistic Regression: Commonly used for binary classification tasks and provides probabilities associated with predictions.
- Deep Learning Models:
- a. Convolutional Neural Networks (CNNs): Applied to image data, such as in genomics for sequence analysis and image-based tasks.
- b. Recurrent Neural Networks (RNNs): Suitable for sequential data, such as time-series gene expression data.
- Ensemble Methods:
- a. Random Forests: Combining the predictions of multiple decision trees to improve overall model performance and robustness.
- b. Gradient Boosting: Building models sequentially, each correcting the errors of the previous one, leading to high accuracy.
- Unsupervised Learning Models:
- a. K-Means Clustering: Applied to group similar biological samples based on their features.
- b. Principal Component Analysis (PCA): Reducing the dimensionality of high-dimensional data to identify underlying patterns.
- Hybrid Models:
- a. Neural Networks with Attention Mechanisms: Combining deep learning with attention mechanisms to focus on relevant features in large-scale biological data.
- b. Transfer Learning: Leveraging knowledge learned from one bioinformatics task to improve performance on a related task.
Selecting the appropriate predictive analytics techniques is crucial for successful outcomes in bioinformatics. Effective data preprocessing ensures the quality of biological data, feature selection identifies the most relevant variables, and model selection tailors the approach to the specific bioinformatics scenario. The subsequent sections will further explore the implementation of these techniques and considerations for their application in predictive analytics within the realm of bioinformatics.
V. Tools and Technologies
A. Bioinformatics Tools
1. Integration of Predictive Analytics into Popular Bioinformatics Tools:
- Bioconductor:
- Description: Bioconductor is an open-source and widely used software platform for the analysis and comprehension of high-throughput genomic data.
- Predictive Analytics Integration: Various Bioconductor packages integrate predictive modeling techniques for tasks such as differential expression analysis, classification, and pathway analysis.
- Galaxy:
- Description: Galaxy is an open, web-based platform for data-intensive biomedical research that provides a user-friendly interface for data analysis workflows.
- Predictive Analytics Integration: Galaxy allows the integration of machine learning tools and algorithms for predictive analytics tasks within its workflow environment.
- Orange Bioinformatics:
- Description: Orange is an open-source data visualization and analysis tool that is extensible for bioinformatics applications.
- Predictive Analytics Integration: Orange Bioinformatics incorporates machine learning components, enabling users to perform predictive analytics on biological datasets.
B. Omics Data Platforms
2. Platforms that Support Predictive Analytics in Omics Research:
- TCGA (The Cancer Genome Atlas):
- Description: TCGA is a comprehensive resource that provides multi-dimensional omics data for various cancer types.
- Predictive Analytics Integration: Researchers can leverage TCGA data for predictive analytics applications, including predicting patient outcomes, identifying biomarkers, and exploring cancer subtypes.
- GEO (Gene Expression Omnibus):
- Description: GEO is a public repository that hosts a wealth of functional genomics datasets, including gene expression data.
- Predictive Analytics Integration: Researchers can use GEO data for predictive modeling tasks such as disease classification and identification of gene expression patterns.
- ENCODE (Encyclopedia of DNA Elements):
- Description: ENCODE is a project that aims to identify all functional elements in the human genome.
- Predictive Analytics Integration: ENCODE provides omics data that can be utilized for predictive analytics tasks, including predicting regulatory elements and understanding the functional impact of genomic variations.
- LINCS (Library of Integrated Network-based Cellular Signatures):
- Description: LINCS is a project that profiles the effects of perturbations on gene expression and other cellular features.
- Predictive Analytics Integration: LINCS data can be used for predictive modeling to understand the relationships between perturbations and cellular responses.
- OMICtools:
- Description: OMICtools is a platform that curates bioinformatics tools and databases, including those for omics data analysis.
- Predictive Analytics Integration: OMICtools provides a curated list of tools that incorporate predictive analytics techniques for various omics applications.
These tools and platforms offer a diverse set of resources for researchers in bioinformatics who wish to integrate predictive analytics into their workflows. From dedicated bioinformatics tools that support machine learning to comprehensive omics data platforms, these technologies empower researchers to apply advanced analytics techniques to decipher complex biological data. The subsequent sections will delve into practical considerations and best practices for utilizing these tools in predictive analytics workflows within the field of bioinformatics.
VI. Applications in Precision Medicine
A. Personalized Treatment Plans
1. How Predictive Analytics Contributes to Precision Medicine:
- Individualized Patient Profiling:
- Predictive analytics analyzes an individual’s omics data, including genomics, transcriptomics, and other relevant data types, to create a comprehensive profile. This profiling considers the unique genetic makeup, molecular characteristics, and variations in gene expression.
- Treatment Response Prediction:
- Predictive models leverage patient data to predict how individuals will respond to specific treatments. This enables healthcare professionals to tailor treatment plans based on the predicted efficacy and potential side effects for each patient.
- Drug Selection and Dosing Optimization:
- Predictive analytics assists in selecting the most effective drugs for individual patients based on their molecular profiles. Additionally, it contributes to optimizing drug dosages to ensure therapeutic benefits while minimizing adverse effects.
- Identification of Biomarkers:
- By analyzing omics data, predictive analytics identifies biomarkers that indicate disease susceptibility, progression, or treatment response. These biomarkers play a crucial role in guiding personalized treatment decisions.
B. Disease Prediction and Prevention
2. Predicting Diseases and Preventing Them Through Analytics:
- Early Disease Prediction:
- Predictive analytics models analyze omics data to identify patterns associated with early stages of diseases. This allows for the early detection of potential health issues, facilitating timely intervention and treatment.
- Risk Stratification:
- Predictive models assess an individual’s risk of developing specific diseases based on their genetic and molecular profiles. This stratification enables targeted preventive measures for individuals at higher risk.
- Lifestyle and Environmental Factors:
- Integrating lifestyle and environmental data with omics information allows predictive analytics to consider holistic factors influencing health. This comprehensive approach enhances the accuracy of disease prediction models.
- Preventive Interventions:
- Predictive analytics guides the design of personalized preventive interventions, including lifestyle modifications, screenings, and vaccination strategies. This proactive approach aims to prevent the onset or progression of diseases.
- Population Health Management:
- Predictive analytics contributes to population health management by identifying groups at higher risk for specific diseases. This information informs public health strategies, resource allocation, and preventive interventions at a broader level.
3. Integrating Predictive Analytics into Clinical Practice:
- Clinical Decision Support Systems:
- Predictive analytics models can be integrated into clinical decision support systems, providing healthcare professionals with real-time insights and recommendations for personalized treatment plans.
- Patient Empowerment:
- Predictive analytics empowers patients by providing them with personalized information about their health risks, treatment options, and lifestyle recommendations. This fosters active participation in healthcare decisions.
- Continuous Monitoring and Adaptation:
- Predictive models can be employed for continuous monitoring of patients, adapting treatment plans based on evolving data. This dynamic approach ensures that treatment strategies remain aligned with the individual’s changing health status.
- Ethical Considerations:
- The ethical use of predictive analytics in precision medicine involves ensuring patient privacy, informed consent, and transparent communication about the uncertainties associated with predictions.
Predictive analytics plays a pivotal role in advancing precision medicine by tailoring treatment plans based on individual variations and predicting disease risks. From personalized treatment strategies to proactive disease prevention, the applications of predictive analytics in precision medicine contribute to a paradigm shift in healthcare, moving towards more targeted and effective interventions. The subsequent sections will explore considerations and challenges associated with the integration of predictive analytics in precision medicine and disease prediction.
VII. Challenges and Future Trends
A. Overcoming Challenges
1. Addressing Common Challenges in Predictive Analytics in Bioinformatics:
- Data Quality and Integration:
- Challenge: Omics data often comes from diverse sources, and ensuring its quality and seamless integration pose challenges.
- Solution: Robust data preprocessing strategies, quality control measures, and standardized data formats facilitate effective data integration.
- Interpretability and Explainability:
- Challenge: Many predictive models in bioinformatics, particularly deep learning models, are perceived as “black boxes,” making it challenging to interpret their predictions.
- Solution: Integrating explainability techniques and model-agnostic interpretability methods enhance the understanding of model predictions.
- Limited Sample Size:
- Challenge: Omics datasets, especially in rare diseases, may have limited sample sizes, impacting the robustness of predictive models.
- Solution: Employing techniques like transfer learning, data augmentation, or ensemble methods to optimize model performance with limited data.
- Biological Complexity:
- Challenge: The intricate nature of biological systems introduces complexity in modeling relationships between variables.
- Solution: Collaborative efforts between biologists and data scientists, along with the incorporation of domain knowledge, enhance the modeling of biologically relevant relationships.
- Ethical Considerations:
- Challenge: Privacy concerns, informed consent, and responsible data use are critical ethical considerations in predictive analytics.
- Solution: Implementing strict privacy protocols, obtaining informed consent, and adhering to ethical guidelines ensure responsible and transparent use of biological data.
B. Emerging Trends
2. Exploring the Future of Predictive Analytics in Omics Research:
- Single-Cell Omics Predictions:
- Trend: Advancements in single-cell omics technologies are paving the way for predictive analytics at the single-cell level, providing unprecedented insights into cellular heterogeneity.
- Integration of Multi-Omics Data:
- Trend: Future trends involve the integration of data from various omics layers, such as genomics, transcriptomics, proteomics, and metabolomics, to enhance the comprehensiveness of predictive models.
- Explainable AI in Bioinformatics:
- Trend: The incorporation of explainable artificial intelligence (XAI) techniques in bioinformatics models is gaining prominence to enhance the interpretability of complex predictive models.
- Dynamic Predictive Models:
- Trend: Dynamic predictive models that can adapt to changing conditions, patient responses, or evolving biological states are emerging to provide more accurate and adaptable predictions.
- Collaborative and Open Science Initiatives:
- Trend: Collaborative efforts and open science initiatives are expected to grow, fostering data sharing, benchmarking, and the development of standardized protocols in predictive analytics research.
- Precision Public Health:
- Trend: The application of predictive analytics in precision public health involves tailoring interventions and public health strategies based on predictive models, addressing population-level health challenges.
- Integration with Electronic Health Records (EHRs):
- Trend: Integrating predictive analytics models with electronic health records enables real-time clinical decision support, enhancing personalized patient care.
- Advancements in AI Hardware:
- Trend: Continued advancements in AI hardware, such as specialized processors for deep learning, contribute to the scalability and efficiency of predictive analytics in handling large omics datasets.
Predictive analytics in bioinformatics is evolving rapidly, driven by ongoing technological advancements and interdisciplinary collaborations. Overcoming challenges related to data quality, interpretability, and ethical considerations will be crucial for the successful implementation of predictive models. The future trends in omics research point toward more sophisticated and adaptable predictive analytics approaches that contribute to a deeper understanding of biological systems and personalized healthcare.
VIII. Conclusion
In conclusion, the wonders of predictive analytics in bioinformatics have ushered in a transformative era in understanding, interpreting, and leveraging the vast and intricate world of biological data. The application of advanced computational techniques and machine learning algorithms to omics datasets has opened new avenues for breakthroughs in research, healthcare, and precision medicine.
Predictive analytics has become an indispensable tool in tailoring personalized treatment plans through individualized patient profiling, treatment response prediction, and biomarker identification. The ability to predict diseases at early stages and stratify risks empowers healthcare professionals and individuals to take proactive measures for prevention and intervention. The integration of predictive analytics into clinical practice is shaping the landscape of precision medicine, offering more targeted and effective healthcare solutions.
While predictive analytics unfolds the wonders of bioinformatics, it is essential to address challenges related to data quality, interpretability, and ethical considerations. Ongoing efforts in overcoming these challenges will contribute to the responsible and ethical use of predictive models in the realm of bioinformatics.
Looking toward the future, emerging trends promise even more sophisticated applications of predictive analytics in omics research. From single-cell omics predictions to the integration of multi-omics data and the rise of precision public health, the potential for predictive analytics to revolutionize our understanding of biology and healthcare is boundless. Collaborative initiatives, open science practices, and the integration of predictive models with electronic health records are shaping the trajectory of predictive analytics in bioinformatics.
In the spirit of exploration and innovation, this dynamic field invites researchers, healthcare professionals, and data scientists to continue pushing boundaries, asking new questions, and envisioning novel applications of predictive analytics. As we stand at the intersection of data science and biology, the journey of predictive analytics in bioinformatics holds the promise of unraveling deeper insights into the mysteries of life and improving the well-being of individuals and populations alike.
Embark on the exciting journey of predictive analytics in bioinformatics, where data-driven discoveries pave the way for a future where precision and personalized insights guide our understanding of biology and shape the future of healthcare.