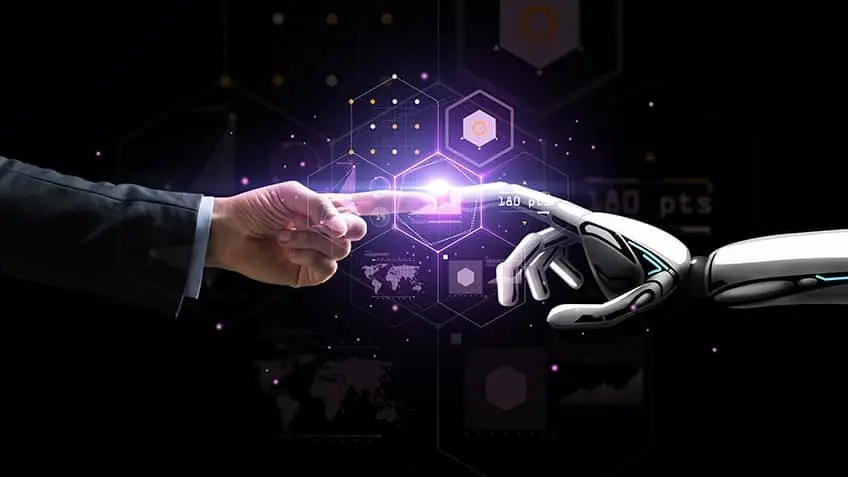
The Evolution of AI-Human Collaboration in the Digital Era
December 13, 2023Table of Contents
I. Introduction
A. Brief overview of the shifting perception of AI from replacement to augmentation
In recent years, the perception of artificial intelligence (AI) has undergone a significant transformation, evolving from a narrative centered around job replacement and automation to one focused on augmentation. Initially, there were concerns that AI technologies would replace human jobs across various industries, leading to widespread job displacement. However, as AI technologies have matured, a more nuanced understanding has emerged, highlighting the potential for collaboration between humans and AI to enhance productivity, creativity, and problem-solving.
This shift in perception recognizes that AI is not merely a tool for automation but a powerful tool for augmenting human capabilities. Instead of being viewed as a threat, AI is increasingly seen as a complementary force that can amplify human skills, enabling individuals to perform tasks more efficiently and effectively. The emphasis has moved from a zero-sum game of man versus machine to a collaborative partnership where AI acts as a supportive force to enhance human potential.
B. Importance of AI-human collaboration in shaping the future
The recognition of the importance of AI-human collaboration stems from the realization that harnessing the strengths of both humans and AI can lead to more innovative solutions and advancements. Human creativity, intuition, and emotional intelligence, combined with AI’s ability to analyze vast datasets, process information rapidly, and identify patterns, create a synergistic relationship. This collaboration has the potential to revolutionize various industries, from healthcare and finance to education and manufacturing.
Moreover, the effective integration of AI into human workflows has the capacity to address complex challenges that are beyond the scope of either humans or machines alone. As we navigate an era of rapid technological advancements, fostering a collaborative approach between humans and AI becomes crucial for staying competitive and ensuring that the benefits of AI are realized in a way that is ethical, inclusive, and sustainable.
In this context, understanding the dynamics of AI-human collaboration becomes paramount for policymakers, businesses, and individuals alike. This paper will delve into the nuances of this evolving relationship, exploring the potential benefits, challenges, and ethical considerations associated with the collaborative future of humans and artificial intelligence.
II. Explainable AI (XAI)
A. Definition and purpose of Explainable AI
Explainable AI (XAI) refers to the development and implementation of artificial intelligence systems in a manner that allows humans to understand how these systems arrive at their decisions or recommendations. The primary purpose of XAI is to make AI algorithms more transparent and interpretable, addressing the “black box” nature of many complex machine learning models. In essence, XAI seeks to provide insights into the reasoning processes of AI systems, making their outputs more understandable and accountable.
B. Importance of transparency in AI systems
Transparency in AI systems is crucial for several reasons. First and foremost, it helps build trust among users, stakeholders, and the general public. Understanding the inner workings of AI algorithms promotes confidence in the technology, especially in critical applications like healthcare, finance, and criminal justice.
Moreover, transparency is essential for identifying and addressing bias in AI models. By making the decision-making processes explicit, developers and users can identify potential sources of bias and take corrective measures, ensuring fairness and equity in AI applications.
Additionally, transparency is vital for compliance with regulatory frameworks. As governments and organizations develop guidelines for ethical AI use, having clear insights into AI models becomes necessary to ensure adherence to legal and ethical standards.
C. Building trust through transparent AI models
Building trust in AI systems involves not only creating transparent models but also fostering a culture of accountability and openness. Communicating how decisions are made, disclosing data sources, and actively addressing concerns related to privacy and bias contribute to establishing trust.
To achieve transparency, developers can use techniques such as providing clear documentation, creating interpretable model architectures, and implementing post-hoc explanation methods. These methods enable users to understand the factors influencing AI decisions, even in complex models like deep neural networks.
D. Real-world examples of XAI applications
- Healthcare Diagnosis: In healthcare, XAI is employed to explain the decisions made by AI models in diagnosing diseases or recommending treatments. This transparency is crucial for gaining acceptance from medical professionals and patients.
- Finance and Credit Scoring: Transparent AI models are used in financial institutions to explain credit scoring decisions. This is essential for ensuring fairness and compliance with anti-discrimination laws.
- Autonomous Vehicles: XAI is applied in the development of self-driving cars to explain the decision-making processes behind navigation and obstacle avoidance. This transparency is crucial for user acceptance and safety.
- Criminal Justice and Predictive Policing: In the context of law enforcement, XAI helps explain the factors influencing predictive policing algorithms, addressing concerns related to bias and fairness.
These examples highlight the diverse applications of Explainable AI across different industries, emphasizing its role in enhancing accountability, fairness, and user acceptance in AI systems.
III. Human-in-the-Loop AI
A. Definition and concept of Human-in-the-Loop AI
Human-in-the-Loop AI refers to a collaborative approach where human intelligence and expertise are integrated into the decision-making process of artificial intelligence systems. In this model, humans play an active role in guiding, validating, and improving the outputs of AI algorithms. The concept recognizes that while AI can automate certain tasks, human oversight and intervention are crucial for handling complex, ambiguous, or context-dependent situations.
This collaborative framework involves a continuous feedback loop where humans provide input, validate AI-generated outputs, and refine the system’s performance. The goal is to harness the strengths of both humans and AI, combining the efficiency and consistency of machine intelligence with the nuanced understanding, creativity, and ethical judgment of humans.
B. Advantages of combining human and AI strengths
- Enhanced Decision-Making: Human-in-the-Loop AI allows for more informed and context-aware decision-making. Humans bring subjective judgment, intuition, and ethical considerations to complement the analytical capabilities of AI.
- Adaptability to Complex Scenarios: Humans excel in handling situations with ambiguity, variability, and unforeseen challenges. Combining human judgment with AI enhances adaptability, making the system more robust in complex and dynamic environments.
- Mitigation of Bias and Ethical Concerns: Human oversight helps identify and address biases in AI models. This collaborative approach ensures that decisions align with ethical standards and societal norms, minimizing the risk of unintended consequences.
- Continuous Learning and Improvement: Humans contribute to the continuous learning process of AI systems by providing feedback, correcting errors, and updating algorithms based on evolving knowledge and circumstances.
C. Applications of Human-in-the-Loop AI in healthcare
- Medical Diagnosis: In healthcare, Human-in-the-Loop AI is employed in medical diagnosis. While AI algorithms can analyze medical images or data, healthcare professionals provide validation, interpretability, and contextual understanding, leading to more accurate diagnoses.
- Drug Discovery: Human-in-the-Loop AI is used in drug discovery processes. AI algorithms assist researchers in analyzing vast datasets, but human experts contribute their domain knowledge to validate and prioritize potential drug candidates.
- Telemedicine and Remote Monitoring: In telemedicine, AI tools can assist in remote patient monitoring. However, healthcare providers remain in the loop to interpret data, consider patient history, and make personalized treatment recommendations.
D. Case studies showcasing successful collaborations
- IBM Watson for Oncology: This system combines AI with the expertise of oncologists to recommend personalized treatment options for cancer patients. Oncologists review and validate the AI-generated insights, ensuring a collaborative approach to cancer care.
- Google’s DeepMind in Healthcare: DeepMind has collaborated with healthcare professionals to develop AI models for tasks such as predicting patient deterioration. Clinicians actively participate in the loop, providing insights and ensuring the responsible use of AI in healthcare settings.
These case studies exemplify successful implementations of Human-in-the-Loop AI, demonstrating how the collaboration between humans and AI can lead to more effective, accurate, and ethical outcomes, particularly in critical domains like healthcare.
IV. Tools and Techniques for Effective Collaboration
A. Overview of emerging tools for AI-human collaboration
- Collaborative Platforms: Platforms that facilitate communication and collaboration between human workers and AI systems. These platforms often include features for data annotation, model training, and result validation.
- Explainability Tools: Tools that provide insights into AI decision-making processes, making it easier for humans to understand and interpret the outputs of AI models. These tools contribute to building trust and transparency in AI systems.
- Human-in-the-Loop Interfaces: Interfaces designed to incorporate human feedback seamlessly into AI processes. These interfaces enable humans to provide input, corrections, or guidance to enhance the performance of AI algorithms.
- Interactive Machine Learning (IML) Tools: Tools that allow users to interact with and steer the learning process of AI models in real-time. This ensures that human expertise is actively involved in shaping the behavior of the AI system.
B. Techniques for seamless integration of human and AI efforts
- Active Learning: Involves the iterative process of having a model query a human expert for information on data it finds challenging. This helps in targeted data labeling, improving the model’s performance with minimal human intervention.
- Human Feedback Loops: Establishing feedback loops where humans provide continuous input on AI-generated outputs. This iterative process helps refine and improve the model over time, adapting to changing conditions or requirements.
- Transfer Learning: Leveraging pre-trained AI models and fine-tuning them with domain-specific data and human input. This technique reduces the amount of data needed for training and allows the model to benefit from human expertise in specific domains.
- Ensemble Models: Combining predictions from multiple AI models and human experts. This approach leverages the strengths of both AI and human intelligence, enhancing the overall accuracy and reliability of the system.
C. Impact on efficiency and productivity in various industries
- Manufacturing: In manufacturing, AI-human collaboration improves efficiency by optimizing production processes, predicting maintenance needs, and enhancing quality control. Humans oversee and validate AI-driven decisions, ensuring seamless and error-free operations.
- Customer Service: AI-powered chatbots and virtual assistants work alongside human agents in customer service. AI handles routine queries, while humans tackle more complex and nuanced customer issues, resulting in quicker response times and improved customer satisfaction.
- Finance: In the financial industry, AI-human collaboration is applied in algorithmic trading, risk management, and fraud detection. Humans provide oversight to ensure compliance, interpret market trends, and address exceptional scenarios that AI may not have encountered.
- Education: AI in education involves personalized learning experiences tailored to individual students. Human teachers collaborate with AI systems to analyze student performance data, provide insights, and offer targeted interventions to enhance the learning process.
- Research and Development: In scientific research, AI assists researchers in analyzing vast datasets, identifying patterns, and predicting outcomes. Human researchers validate findings, interpret results, and contribute domain expertise, accelerating the pace of discovery.
The integration of AI tools and techniques into various industries not only enhances efficiency and productivity but also leverages the strengths of both humans and machines. This collaborative approach ensures that AI systems are applied judiciously, with human oversight and expertise guiding the decision-making process.
V. Ethical Considerations in AI-Human Collaboration
A. Addressing concerns related to job displacement
- Reskilling and Education Initiatives: Implementing programs to reskill and upskill the workforce to adapt to changing job requirements. This helps mitigate job displacement by preparing individuals for roles that complement AI technologies.
- Social Safety Nets: Establishing social safety nets to support workers who may be adversely affected by automation or AI-driven changes. This could include unemployment benefits, job placement programs, and other forms of assistance.
- Transparent Communication: Providing clear and transparent communication about the role of AI in the workplace and its potential impact on jobs. Open dialogue helps manage expectations and fosters a collaborative environment between humans and AI.
B. Ensuring fairness and inclusivity in collaborative systems
- Bias Mitigation: Actively addressing and mitigating biases in AI systems to ensure fair outcomes. This involves regular audits of algorithms, diverse and representative training datasets, and the use of fairness-aware techniques during model development.
- Inclusive Design: Incorporating principles of inclusive design to ensure that AI systems are accessible and beneficial to individuals across diverse backgrounds, abilities, and demographics.
- User Feedback and Input: Encouraging user feedback and input to identify potential biases or unintended consequences. This includes involving a diverse group of users in the testing and evaluation of AI systems to ensure fair and equitable performance.
C. Ethical guidelines for developers and organizations
- Transparency and Explainability: Developers should prioritize transparency and explainability in AI systems to build trust and allow users to understand how decisions are made.
- Privacy Protection: Implementing robust privacy measures to safeguard user data and ensure that AI applications comply with privacy regulations. This involves anonymizing data, obtaining informed consent, and minimizing data collection.
- Accountability and Responsibility: Developers and organizations should take responsibility for the ethical implications of their AI systems. This includes being transparent about limitations, actively addressing biases, and responding to concerns raised by users or stakeholders.
- Ethical Review Boards: Establishing ethical review boards or committees to assess the potential impact of AI applications on society, especially in sensitive domains such as healthcare, criminal justice, and finance.
- Adherence to Ethical Frameworks: Following established ethical frameworks and guidelines, such as those provided by international organizations or industry associations, to ensure responsible and ethical AI development and deployment.
By addressing job displacement concerns, ensuring fairness and inclusivity, and adhering to ethical guidelines, the collaboration between humans and AI can be guided by principles that prioritize the well-being of individuals and communities. This ethical foundation is crucial for the responsible and sustainable integration of AI technologies into various aspects of society.
VI. Future Trends and Innovations
A. Predictions for the continued evolution of AI-human collaboration
- Enhanced Human-AI Interfaces: The development of more intuitive and natural interfaces that facilitate seamless interaction between humans and AI, making collaboration more user-friendly and efficient.
- Increased Personalization: AI systems will become more adept at personalizing interactions based on individual preferences, behaviors, and needs, leading to more tailored and effective collaborations.
- AI-Driven Creativity: Advances in AI capabilities to actively participate in creative processes, such as content creation, design, and innovation, augmenting human creativity rather than replacing it.
- Ubiquitous AI Integration: AI technologies will become more integrated into daily life, work, and decision-making processes, creating a pervasive presence that requires ongoing collaboration between humans and AI.
- Explainability Improvements: Continued efforts to enhance the explainability of AI models, ensuring that humans can understand and trust the decisions made by increasingly complex algorithms.
B. Emerging technologies shaping the landscape
- Edge Computing for AI: The use of edge computing to bring AI capabilities closer to the source of data, reducing latency and enabling real-time decision-making in various applications.
- Generative AI Models: Advancements in generative models that can create realistic content, such as images, text, and videos, opening up new possibilities for creative collaboration between humans and AI.
- Natural Language Processing (NLP) Breakthroughs: Progress in natural language processing, allowing AI systems to understand and generate human-like language with greater accuracy and contextual understanding.
- Augmented Reality (AR) and Virtual Reality (VR): Integration of AR and VR technologies with AI to create immersive and collaborative environments, transforming how humans interact with AI applications.
- AI for Scientific Discovery: Increased use of AI in scientific research and discovery, accelerating breakthroughs in fields such as medicine, materials science, and environmental studies.
C. Potential societal impacts and implications
- Job Transformation: While some jobs may be displaced, there will be a simultaneous transformation of job roles, with an increased focus on tasks that require uniquely human skills such as creativity, empathy, and complex problem-solving.
- Ethical and Legal Challenges: As AI becomes more integrated into various aspects of society, addressing ethical concerns and navigating legal frameworks surrounding AI-human collaboration will become increasingly complex.
- Education and Skill Development: The need for continuous education and skill development to prepare the workforce for evolving job requirements in the era of AI-human collaboration.
- Economic Disparities: The potential for AI-driven economic disparities, as organizations and individuals with access to advanced AI technologies may have a competitive advantage over those without.
- Healthcare and Well-being: AI-human collaboration has the potential to revolutionize healthcare, with advancements in personalized medicine, early diagnosis, and treatment, but ethical considerations around data privacy and security will be crucial.
The future of AI-human collaboration holds exciting possibilities but also presents challenges that require thoughtful consideration. Balancing innovation with ethical, legal, and societal implications will be essential to ensure that these technologies contribute positively to the well-being of individuals and society at large.
VII. Conclusion
A. Recap of key points on AI-human collaboration
In conclusion, the evolution of AI-human collaboration has shifted from concerns about job displacement to a recognition of the potential for augmentation and synergy between human and artificial intelligence. Key points discussed include:
- The changing perception of AI from replacement to augmentation.
- The importance of transparency in AI systems through Explainable AI (XAI).
- The concept of Human-in-the-Loop AI, emphasizing the collaboration between humans and AI for enhanced decision-making.
- Tools and techniques for effective collaboration, spanning from collaborative platforms to interactive machine learning tools.
- Ethical considerations, addressing job displacement concerns, ensuring fairness, and providing ethical guidelines for developers and organizations.
- Future trends and innovations, predicting continued evolution, emerging technologies, and potential societal impacts.
B. Encouraging a positive outlook on the future of technology
While challenges exist, the positive outlook on the future of AI-human collaboration is grounded in the belief that these technologies can enhance human capabilities, drive innovation, and address complex challenges. The collaboration between humans and AI has the potential to revolutionize industries, improve efficiency, and contribute to societal well-being.
C. Call-to-action for readers to engage with the content and share their thoughts
As we navigate the future of AI-human collaboration, it’s crucial for individuals, organizations, and policymakers to actively engage with these discussions. Share your thoughts, insights, and experiences related to AI collaboration. Stay informed about emerging trends, ethical considerations, and best practices in the field. By fostering an open dialogue and collaboration, we can collectively shape a future where AI technologies complement human endeavors, contributing to a more inclusive, innovative, and ethically grounded society.