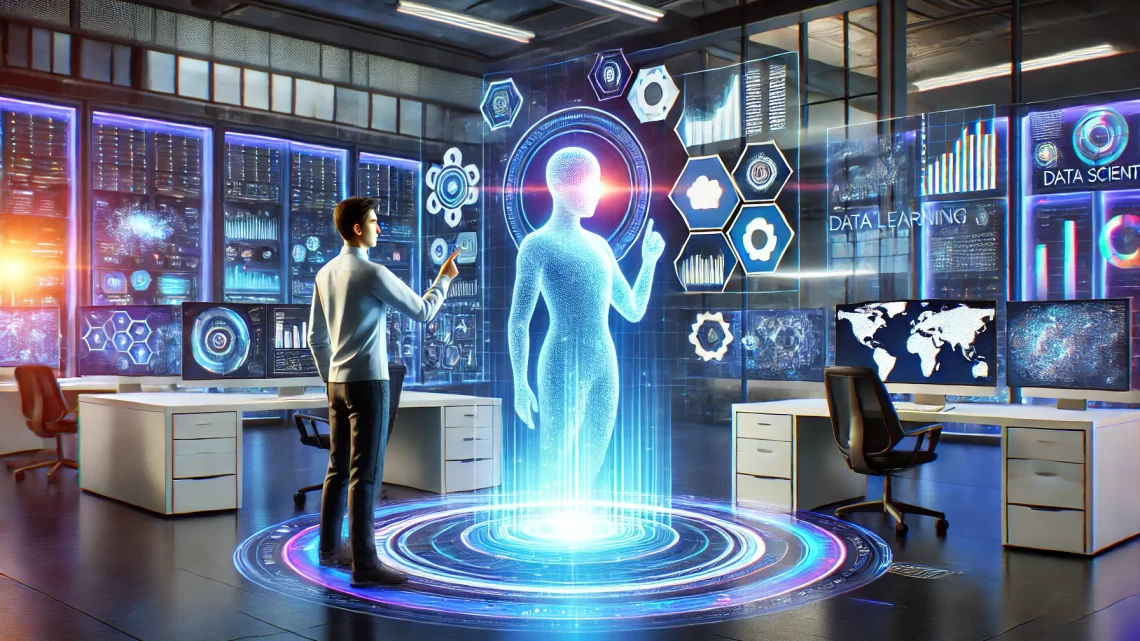
Translational bioinformatics and data science for biomarker discovery in mental health
December 18, 2024Mental health disorders, encompassing conditions such as Alzheimer’s disease, major depressive disorder (MDD), schizophrenia, bipolar disorder (BD), autism spectrum disorder (ASD), attention-deficit/hyperactivity disorder (ADHD), and post-traumatic stress disorder (PTSD), represent some of the most significant public health challenges of our time. As their prevalence continues to rise globally, the need for accurate, early diagnosis and effective personalized treatments has never been more critical. However, the complexity of these disorders, both in terms of their clinical presentation and their underlying molecular mechanisms, has made their study and treatment notoriously difficult. This is where the transformative power of bioinformatics and data science comes into play. By leveraging advances in genomics, proteomics, metabolomics, and other ‘omics’ technologies, researchers are uncovering new potential biomarkers that could revolutionize mental health diagnosis and therapy. This blog post delves into how bioinformatics and data science are driving the discovery of biomarkers for mental health disorders and shaping the future of personalized medicine.
Table of Contents
The Rise of Big Data in Mental Health Research
In recent years, the healthcare sector has experienced an explosion of data, ranging from electronic health records (EHR) and medical imaging to genomics, wearable devices, and mobile health applications. The influx of “Big Data” provides a double-edged sword for researchers—it presents both a massive opportunity for new insights into mental health and a significant challenge in terms of processing, integrating, and interpreting the data.
This is where bioinformatics and data science come in, utilizing advanced algorithms, artificial intelligence (AI), and cloud computing to process and analyze these vast datasets. By applying machine learning (ML) and deep learning techniques, scientists are able to examine large-scale data from diverse sources such as DNA, mRNA, proteins, metabolites, and even environmental and lifestyle factors. These interdisciplinary fields are helping to extract meaningful biological insights, paving the way for the identification of novel biomarkers and improving our understanding of the complex biological processes underlying mental health disorders.
Biomarkers: The Key to Precision Mental Healthcare
Biomarkers—measurable biological indicators of disease—are essential to precision medicine, allowing clinicians to identify disease subtypes, predict progression, and tailor treatment to individual patients. For mental health disorders, where symptom presentation can vary widely, biomarkers could hold the key to improving diagnostic accuracy and treatment outcomes.
Currently, there are few approved biomarkers for mental health conditions, which underscores the importance of ongoing research. Identifying robust and reproducible biomarkers through genomic, proteomic, and metabolomic studies will enable the development of clinical decision support systems (CDSS) that can integrate with personalized treatment plans. These systems, powered by AI, could revolutionize how mental health disorders are managed, making treatment both more effective and more personalized.
Potential Biomarkers for Mental Health Disorders
1. Alzheimer’s Disease (AD)
Alzheimer’s disease (AD) is one of the most well-studied mental health disorders in terms of biomarkers. Recent proteomic studies have uncovered several potential protein-based biomarkers, including those associated with amyloid plaques and hyperphosphorylated tau proteins, which are central to AD pathophysiology. Genomic studies have identified mutations in genes like APP, TREM2, and PLD3, which contribute to both early-onset and late-onset AD. Moreover, next-generation sequencing (NGS) and microRNA studies have revealed additional genetic markers and potential therapeutic targets. Functional imaging, including MRI and PET scans, is also being used to detect changes in the brain related to amyloid deposition, enhancing early diagnosis.
2. Major Depressive Disorder (MDD)
MDD is a leading cause of disability worldwide, and its biomarkers are being actively explored. Proteomic studies have identified several serum proteins linked to inflammation, neurotransmitter signaling, and cellular communication in MDD patients. On the genomic front, studies have found that dysregulation of the hypothalamic-pituitary-adrenal (HPA) axis, as well as specific genetic variants in receptors like NR3C2 and CRHR1, are strongly associated with MDD. Furthermore, epigenomic changes, such as DNA methylation of genes like BDNF and SLC6A4, have also been implicated in the pathophysiology of depression.
3. Schizophrenia (SCZ)
Schizophrenia is another mental health disorder for which bioinformatics is providing new insights into potential biomarkers. Proteomic research has revealed alterations in proteins like HINT1, which is associated with brain function. NGS studies have highlighted mutations in neuregulin-1 (NRG1) and DISC1, which are linked to an increased risk of schizophrenia. Epigenomic modifications and copy number variations (CNVs) have further elucidated the genetic underpinnings of this complex disorder. By integrating these findings with metabolomics data, which points to disrupted glutamate metabolism, researchers are getting closer to identifying reliable biomarkers for schizophrenia.
4. Bipolar Disorder (BD)
Bipolar disorder presents another challenge for researchers aiming to find effective biomarkers. Proteomic studies have identified key proteins, such as B2RAN2 and ENG, that distinguish BD from MDD. Genomic studies have pointed to mutations in DISC1, while epigenetic modifications in the BDNF gene have also been noted. Metabolomics research has identified several metabolites, including lactate and trimethylamine oxide, that are potential biomarkers for BD. These findings are helping to refine the molecular distinctions between BD and other mental health conditions, particularly depression.
5. Autism Spectrum Disorder (ASD)
ASD is characterized by social communication deficits and repetitive behaviors, and its biomarker discovery is an area of active research. Proteomic studies have highlighted proteins involved in neurodevelopment, such as p140Cap, while genomic studies have revealed mutations in the DISC1 gene. Epigenetic changes in genes like OXTR and MECP2 are also associated with ASD. Additionally, metabolites such as plasma amino acids and lipids have been linked to ASD outcomes, providing further potential diagnostic and therapeutic targets.
6. Attention-Deficit/Hyperactivity Disorder (ADHD)
ADHD, a neurodevelopmental disorder, has been studied in terms of molecular markers as well. Research has shown alterations in neurotransmitter systems, with specific protein changes linked to GABA transmission. Genomic studies have highlighted genes like ABCB5 and GAK, while epigenomic research has pointed to changes in the CREB5 gene. Metabolomics has identified specific metabolites, including dopamine-related compounds, as potential biomarkers for ADHD.
The Role of Multiomics and Systems Biology
A multiomics approach, which integrates data from various biological disciplines, is transforming our understanding of mental health disorders. By combining genomics, proteomics, metabolomics, and other ‘omics’ data, researchers can gain a more comprehensive view of the biological mechanisms driving these diseases. Systems biology tools, such as StringDB and Enrichr, allow scientists to connect biomarkers with relevant biological processes, providing deeper insights into the pathogenesis of mental health disorders.
The Gut-Brain Axis and Mental Health
An exciting area of research gaining momentum is the gut-brain axis, which links the gut microbiome with brain function. Dysregulations in gut bacteria have been implicated in a range of mental health conditions, including neurodegenerative diseases and mood disorders. Exploring the gut-brain connection could open new avenues for treatment, with potential therapies like “psychobiotics” and fecal transplants offering innovative approaches to complement traditional treatments.
Clinical Applications and Future Directions
The integration of molecular technologies into clinical practice is paving the way for more personalized and precise treatments for mental health disorders. Pharmacogenomic testing, for example, can help clinicians predict which medications will be most effective for individual patients based on their genetic makeup. The future may also see the development of molecular diagnostic panels for specific mental health conditions, supported by AI-powered clinical decision support systems (CDSS) that provide real-time, personalized recommendations.
Despite the promising advancements, challenges remain, including the need for more actionable biomarkers, standardized diagnostic criteria, and increased research funding. However, the future holds immense potential, with breakthroughs in multiomics, AI, and systems biology set to drive the next wave of innovation in mental health research and treatment.
Conclusion
The intersection of bioinformatics, data science, and mental health research is transforming our approach to understanding and treating mental health disorders. By leveraging cutting-edge technologies and interdisciplinary research, scientists are uncovering new biomarkers that hold the key to more effective, personalized treatments. As the field continues to evolve, the integration of molecular data with clinical practice promises to improve patient outcomes and provide new hope for those affected by mental health conditions.
FAQ on Biomarkers in Mental Health
What are biomarkers and how can they be beneficial for mental health disorders? Biomarkers are measurable indicators of a biological state or condition. They can be used for various purposes in mental health, including diagnosing disorders, predicting their progression (prognostic), monitoring treatment responses, and identifying subtypes of diseases. Ideal biomarkers are reliable, reproducible, and confirmed by multiple independent studies. By uncovering underlying biological mechanisms of mental disorders, biomarkers can lead to the development of advanced clinical description support systems, facilitating personalized medicine through AI models.
What molecular technologies are being utilized to discover biomarkers for mental health conditions? Various molecular technologies are employed, including genomics, transcriptomics (RNA sequencing), proteomics, metabolomics, epigenomics, and multiomics. Genomics studies DNA variations, while transcriptomics studies gene expression. Proteomics focuses on protein analysis, while metabolomics examines metabolites and glycomics looks at carbohydrates. Epigenomics explores chemical modifications to DNA, and multiomics integrates these datasets for a comprehensive view. Specific techniques include next-generation sequencing (NGS), mass spectrometry, and various imaging techniques like MRI and PET scans. These methods analyze samples such as blood, serum, urine, brain tissue, and cerebrospinal fluid.
Which mental health disorders are being studied for biomarker identification and what are some specific findings? A wide range of mental health disorders are being studied, including major depressive disorder (MDD), schizophrenia (SCZ), bipolar disorder (BD), autism spectrum disorder (ASD), attention deficit hyperactivity disorder (ADHD), post-traumatic stress disorder (PTSD), anxiety disorders, and neurodegenerative diseases like Alzheimer’s disease (AD). Specific findings include:
MDD: Alterations in serum proteins related to cell communication, inflammation, and metabolism; increased HINT1 protein levels in the brain (while SCZ exhibits lowered levels); dysregulated genes involved in glutamate transmission, GABA signaling, and serotonin pathways; HPA axis dysregulation; and various SNPs in genes like CRHR1, NR3C1, NR3C2 related to stress response and cognition.
SCZ: Altered HINT1 protein levels, NMDA receptor and GABA abnormalities, disrupted gene expression patterns, and mutations in genes like NRG1, ZNF804A, and CRMP2; dysregulation of oligodendrocytes and glutamatergic neurotransmission.
BD: Changes in protein levels of B2RAN2 and ENG (potential biomarkers to differentiate BD from MDD); dysregulated genes and proteins in pathways like FXR/RXR activation, LXR/RXR activation, acute phase response signaling, and endocytosis.
ASD: Variations in apolipoproteins and fibronectin, an increase in glyoxalase I (Glo1) protein levels; dysregulation of genes DIO2, Cirbp, DNMT3A, DNMT3B, TET1, TET3, methylation changes in genes like OXTR, GAD1, EN2, RELN and MECP2.
ADHD: Changes in gene expression such as ABCB5, RGS2, GAK, GIT1; and higher rates of copy number variations (CNVs) in the genome and methylation changes in CREB5 and MAD1L1.
PTSD: dysregulated immune response and HPA axis pathways; alterations in microRNAs associated with HPA axis regulation through FKBP5; lower mitochondrial DNA copy numbers; 13 significant metabolite changes including glycerophospholipids and an endocannabinoid signaling metabolite; methylation changes in FKBP5 and SLC6A4.
AD: Amyloid plaques and structural abnormalities in brain imaging; lipid panel in peripheral blood; dysregulated genes ITGB5, RPH3A, GNAS, THY1 and SEPT6 , and abnormal AMP expression.
What is the role of gene expression studies in biomarker discovery for mental health disorders?
Gene expression studies, often performed through RNA sequencing, can reveal how the levels of messenger RNA (mRNA) are altered in different mental health conditions. For example, in MDD, various dysregulated genes have been identified in different brain regions. In SCZ and BD, altered mRNA levels of RELN have been observed. Differentially expressed genes identified in gene expression studies may serve as biomarkers and provide insights into potential therapeutic targets.
How do microRNAs contribute to biomarker discovery in mental health?
MicroRNAs (miRNAs) are small non-coding RNA molecules that regulate gene expression. Changes in miRNA expression have been linked to many mental health disorders. For instance, miR-132 affects BDNF expression (a key protein in brain plasticity) and is also implicated in neuropsychiatric disorders, while miR-182 is found to be activated in BD and healthy controls but down-regulated in MDD and SCZ patients. In PTSD, microRNAs related to HPA axis regulation and immune response have been found to be dysregulated. These findings suggest miRNAs could be promising biomarkers and therapeutic targets.
How is the gut microbiome being explored as a potential biomarker in mental health? The gut microbiome, composed of trillions of microorganisms in the gastrointestinal tract, has a bidirectional relationship with the brain via the gut-brain axis. Dysregulations in gut microbiota have been linked to neurodegenerative diseases, mood disorders, and depression. The microbiome affects human behavior, brain function, and immunity and influences metabolism, appetite, and immunity. Research is exploring the use of therapies like psychobiotics and fecal transplants to improve mental health via microbiome modulation. Specific alterations in gut microbiota profiles have been noted in MDD, SCZ, BD, ASD, and ADHD.
What are multiomics studies and how are they beneficial in the field of mental health research?
Multiomics studies combine data from different ‘omic’ levels, including genomics, transcriptomics, proteomics, metabolomics, and epigenomics, to provide a more comprehensive understanding of disease mechanisms. By integrating these complex data sets, multiomics analyses can discover interactions between different molecular layers that are not visible when each type is studied alone. For example, multiomics analyses in MDD have found intricate molecular changes relating to the pathophysiology of MDD, potentially leading to improved therapeutic strategies. In SCZ, these approaches can highlight potential therapeutic targets such as nuclear FGFR1 signaling dysregulation, and in ADHD, can reveal connections between genetic variation, gene expression, and methylation patterns.
How is the integration of molecular technologies into the clinic impacting the diagnosis and treatment of mental health disorders?
The integration of molecular technologies is revolutionizing the clinical management of mental health disorders. Traditional diagnostic methods such as questionnaires and clinical assessments are now being complemented by molecular omics technologies. Pharmacogenomic testing is emerging as a tool to predict response to antidepressant drugs by analyzing genes involved in drug metabolism and action. New insights have also led to the use of drugs that regulate the epigenome by influencing DNA methylation and histone acetylation, offering more targeted treatments. Ultimately, the increased knowledge derived from biomarkers and molecular technologies should facilitate the creation of personalized treatment plans and improve outcomes for those suffering from mental health disorders.
Glossary of Key Terms
- Biomarker: A measurable substance or characteristic in the body that can indicate a normal or disease process, or response to a treatment.
- Proteomics: The study of the entire set of proteins in a cell, tissue, or organism, including their structure, function, and interactions.
- Transcriptomics: The study of the complete set of RNA transcripts in a cell, including their levels of expression, which indicates which genes are active.
- Genomics: The study of an organism’s complete set of DNA, including gene sequences, variations, and their functions.
- Epigenomics: The study of heritable changes in gene expression that do not involve alterations to the underlying DNA sequence. These changes include methylation and histone modification.
- Metabolomics: The study of small molecules, or metabolites, in a biological system, providing insights into metabolic pathways and their dysregulation.
- Multiomics: An approach that integrates data from multiple omics technologies (e.g., genomics, proteomics, metabolomics) to achieve a more comprehensive understanding of biological systems.
- MicroRNA (miRNA): Small non-coding RNA molecules that regulate gene expression by targeting mRNA for degradation or translational repression.
- HPA axis: The hypothalamic-pituitary-adrenal axis, a complex neuroendocrine system that controls the body’s response to stress.
- Copy Number Variation (CNV): Variations in the number of copies of a DNA sequence, which can be associated with disease.
- Gut-Brain Axis: The bidirectional communication system between the gastrointestinal tract and the brain, influencing behavior, cognition, and mental health.
- RDoC (Research Domain Criteria): A framework by the National Institute of Mental Health (NIMH) that approaches the study of mental illness based on observable behavior and neurobiological measures rather than traditional diagnostic categories.
- SNP (Single Nucleotide Polymorphism): A variation in a single nucleotide at a specific position in the genome.
- CDSS (Clinical Description Support Systems): Systems that are empowered by sophisticated AI models to advance personalized medicine.
- GWAS (Genome-Wide Association Study): An examination of a genome-wide set of genetic variants in different individuals to see if any variant is associated with a trait.
- RNA-seq: A sequencing technique used to analyze the transcriptome, or the total RNA present in a cell or tissue.
- EWAS (Epigenome-Wide Association Study): An analysis that looks at epigenetic markers (like DNA methylation) across the entire genome to associate them with traits or diseases.
Reference
Bhuvaneshwar, K., & Gusev, Y. (2024). Translational bioinformatics and data science for biomarker discovery in mental health: an analytical review. Briefings in Bioinformatics, 25(2), bbae098.