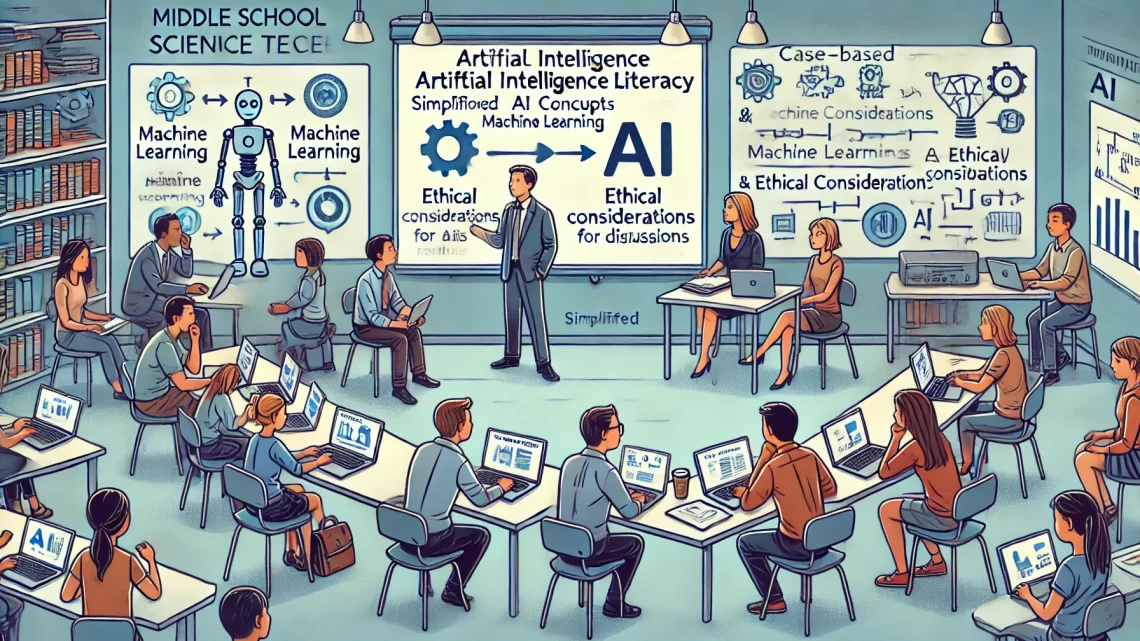
Mastering Cutting-Edge Bioinformatics Techniques: A Comprehensive Guide
February 22, 2025Introduction
In the rapidly evolving realm of bioinformatics, mastering advanced techniques is akin to solving a highly intricate scientific puzzle. Researchers must adeptly utilize an array of sophisticated methodologies such as high-throughput sequencing, genome-wide association studies (GWAS), and epigenomic data integration to decode complex biological processes and pinpoint genetic variants linked to diseases. The seamless integration of multi-omics data and the development of efficient computational pipelines play a crucial role in transforming raw data into meaningful biological insights. But how do these advanced methodologies elevate bioinformatics research? The answer lies in a systematic and meticulous approach to data analysis.
Key Takeaways
- Leverage machine learning models to improve sensitivity and specificity in structural variant detection.
- Integrate multi-omics datasets to obtain a comprehensive view of regulatory networks and genetic associations.
- Utilize advanced data visualization tools for dynamic exploration and interpretation of genomic data.
- Develop and implement integrative analysis pipelines to streamline variant calling, annotation, and quality control.
- Employ both de novo and reference-based transcriptome assembly techniques for precise isoform detection and quantification.
High-Throughput Sequencing Analysis
High-throughput sequencing (HTS), commonly known as next-generation sequencing (NGS), has revolutionized genomics by enabling the rapid and comprehensive examination of entire genomes. This cutting-edge technology provides an unparalleled level of detail in genomic data, significantly enhancing our ability to detect genetic variations, mutations, and disease-associated markers.
Key Considerations in High-Throughput Sequencing
Sequencing Quality and Read Depth
- Sequencing Quality: Refers to the accuracy of nucleotide base calls during sequencing. High-quality sequencing data is essential for identifying genetic variants with precision. Phred scores are widely used to assess sequencing accuracy, offering confidence levels for each base call.
- Read Depth (Coverage): The number of times a specific nucleotide is read during sequencing. Higher coverage increases the reliability of variant detection by minimizing the risk of sequencing errors and improving the identification of rare variants. Cancer genomics, for instance, requires deep sequencing to detect low-frequency mutations crucial for targeted therapies.
Quality Control and Error Correction
To maintain high sequencing accuracy, researchers implement stringent quality control protocols. Bioinformatics tools such as FastQC, Trimmomatic, and Cutadapt are commonly used for filtering, trimming, and correcting sequencing errors, ensuring data reliability before downstream analysis.
Genome-Wide Association Studies (GWAS)
Genome-wide association studies are a powerful approach for identifying genetic variants linked to complex diseases. By analyzing vast genetic datasets, GWAS allows researchers to pinpoint single nucleotide polymorphisms (SNPs) associated with specific phenotypic traits, providing valuable insights into disease mechanisms.
Methodology and Data Processing
- Genotyping Arrays: These high-throughput platforms scan the genome for millions of SNPs, enabling large-scale genetic analysis.
- Statistical Modeling: GWAS employs sophisticated statistical models, such as linear regression and mixed models, to identify phenotype-genotype associations while controlling for confounders like population stratification.
- Genetic Mapping: Identified SNPs are mapped to genes and regulatory regions, facilitating functional annotation and identification of potential therapeutic targets.
Significance of Population Diversity in GWAS
Expanding GWAS datasets to include diverse populations improves result generalizability and minimizes biases toward specific genetic backgrounds. Multi-ethnic cohort studies enhance the robustness of genetic associations, leading to more accurate risk predictions and therapeutic interventions.
Epigenomic Data Integration
Integrating epigenomic data into bioinformatics analysis enables researchers to uncover gene regulatory mechanisms influencing complex traits and diseases. Epigenetic modifications, such as DNA methylation and histone modifications, provide an additional layer of gene expression regulation beyond genetic sequences.
Key Techniques for Epigenomic Analysis
- Chromatin Accessibility Mapping: Techniques like ATAC-seq and DNase-seq identify open chromatin regions that correlate with active regulatory elements.
- DNA Methylation Analysis: Tools such as Bismark and EpiTools enable precise profiling of methylation patterns, shedding light on gene silencing mechanisms.
- ChIP-seq for Histone Modifications: Model-based Analysis of ChIP-Seq (MACS) detects histone modification enrichment, facilitating the discovery of novel regulatory elements.
Applications in Disease Research
Epigenomic profiling allows for comparative analyses between healthy and diseased tissues, identifying epigenetic alterations associated with conditions such as cancer and neurodegenerative disorders. These insights pave the way for targeted epigenetic therapies.
Structural Variant Detection
Structural variants (SVs), including insertions, deletions, and translocations, play a significant role in genetic disorders and cancer. Detecting these variants accurately requires specialized bioinformatics approaches.
Machine Learning in Structural Variant Detection
- Predictive Algorithms: Machine learning models, such as convolutional neural networks (CNNs) and support vector machines (SVMs), enhance the detection of complex SVs.
- Data Integration: Combining multiple data sources, such as read-pair, split-read, and read-depth methods, improves SV discovery accuracy.
- Variant Annotation: Tools like ANNOVAR and VEP integrate functional data to assess the impact of detected variants on gene function and disease susceptibility.
Transcriptome Assembly Techniques
Transcriptome assembly reconstructs the complete set of RNA transcripts in a cell, providing crucial insights into gene expression and regulation.
De Novo Assembly vs. Reference-Based Assembly
De Novo Assembly
- Useful for organisms without a reference genome.
- Requires kmer selection and contig ordering to build transcript sequences from short reads.
- Tools: Trinity, SOAPdenovo-Trans
Reference-Based Assembly
- Aligns RNA-seq reads to an existing genome, ensuring higher accuracy.
- Reference Selection: The choice of reference genome is critical for optimal alignment.
- Tools: HISAT2, STAR, StringTie
Quality Control and Functional Annotation
- Assessment Tools: TransRate and RSEM evaluate assembly reliability.
- Functional Annotation: Databases such as UniProt and Pfam aid in assigning biological significance to transcripts.
Conclusion
Advanced bioinformatics techniques empower researchers to navigate the complexities of genomic and transcriptomic data with precision. From high-throughput sequencing to GWAS and epigenomic integration, these methodologies offer transformative insights into genetic regulation, disease mechanisms, and potential therapeutic targets. As computational tools evolve, the integration of machine learning, multi-omics data, and robust quality control measures will further refine bioinformatics analyses, driving groundbreaking discoveries in biomedical research.