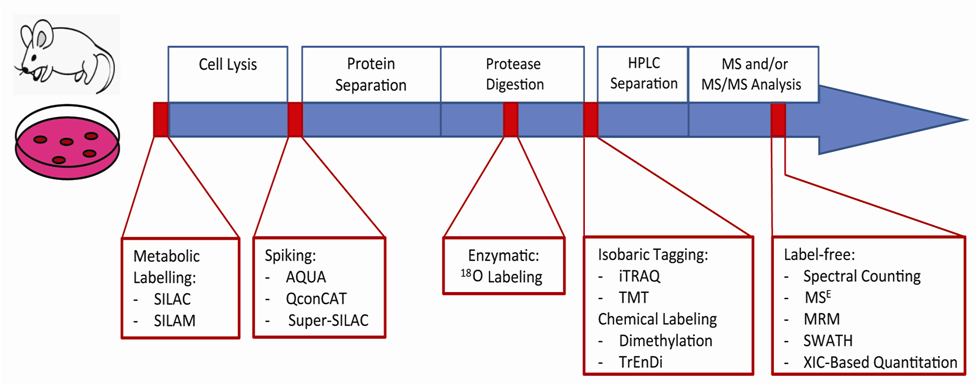
Quantitative Proteomics Techniques
March 20, 2024 Off By adminCertainly! Here’s an outline for a course on quantitative proteomics techniques:
Table of Contents
ToggleCourse Title: Quantitative Proteomics Techniques
Course Overview:
This course will explore techniques used for quantifying proteins in biological samples, focusing on label-free quantitation and stable isotope labeling methods. Students will learn the principles behind each technique.
Prerequisites:
Basic knowledge of biochemistry, molecular biology, and proteomics.
Introduction to Quantitative Proteomics
Overview of quantitative proteomics and its importance in biological research
Quantitative proteomics is a branch of proteomics that aims to determine and compare the abundance of proteins in biological samples under different conditions. This field plays a crucial role in biological research by providing insights into the dynamic changes of protein expression, post-translational modifications, and protein-protein interactions, which are essential for understanding various biological processes and diseases.
Key aspects of quantitative proteomics include:
- Protein Quantification Methods: Quantitative proteomics employs various methods to measure protein abundance, such as label-based techniques (e.g., SILAC, TMT, iTRAQ) and label-free methods (e.g., spectral counting, intensity-based quantification).
- Sample Preparation and Fractionation: Sample preparation techniques are used to extract proteins from biological samples, digest them into peptides, and separate them for analysis to reduce sample complexity and improve quantification accuracy.
- Mass Spectrometry (MS): Mass spectrometry is the core technology used in quantitative proteomics to identify and quantify proteins in a sample based on their mass-to-charge ratios. Advances in MS technology, such as high-resolution MS and tandem MS (MS/MS), have significantly enhanced the sensitivity and accuracy of protein quantification.
- Data Analysis and Bioinformatics: Analyzing quantitative proteomics data requires sophisticated bioinformatics tools and algorithms to process MS data, identify proteins, quantify their abundance, and perform statistical analysis to identify significant changes between samples.
- Applications in Biological Research: Quantitative proteomics is used in various biological research areas, including:
- Biomarker Discovery: Identifying proteins that are differentially expressed in diseased versus healthy states can lead to the discovery of diagnostic and prognostic biomarkers for diseases.
- Drug Discovery and Development: Understanding changes in protein expression in response to drug treatment can help identify drug targets and assess drug efficacy and toxicity.
- Functional Proteomics: Studying changes in protein abundance and modifications can provide insights into protein function, cellular signaling pathways, and regulatory mechanisms.
- Systems Biology: Integrating quantitative proteomics data with other omics data (e.g., genomics, transcriptomics) can provide a comprehensive view of biological systems and their responses to perturbations.
Quantitative proteomics continues to evolve with advancements in technology and methodology, enabling researchers to explore the complexity of the proteome and its role in health and disease.
Principles of protein quantitation: absolute vs. relative quantitation
Protein quantitation in proteomics can be broadly categorized into two approaches: absolute quantitation and relative quantitation. Here’s an overview of the principles of each approach:
- Absolute Quantitation:
- Principle: Absolute quantitation determines the exact amount or concentration of a specific protein in a sample. It provides quantitative information about the abundance of a protein in absolute terms (e.g., nanograms per milliliter).
- Methodologies: Absolute quantitation methods often involve the use of known standards or reference materials. Isotope dilution mass spectrometry (IDMS) is a common technique where a known amount of isotopically labeled internal standard is added to the sample, and the ratio of labeled to unlabeled peptides is used to quantify the target protein.
- Advantages: Provides precise and accurate measurements of protein abundance, allowing for direct comparison between different samples and conditions.
- Applications: Useful for quantifying proteins in biomarker discovery, clinical diagnostics, and studying protein dynamics in biological systems.
- Relative Quantitation:
- Principle: Relative quantitation compares the abundance of proteins between different samples or conditions, without determining the exact concentration of individual proteins.
- Methodologies: Label-based (e.g., SILAC, TMT, iTRAQ) and label-free methods (e.g., spectral counting, intensity-based quantification) are commonly used for relative quantitation. These methods measure the relative changes in protein abundance between samples based on isotopic labeling or spectral features.
- Advantages: Allows for the comparison of protein abundance changes across different experimental conditions, such as diseased versus healthy states or treated versus untreated samples.
- Applications: Useful for identifying differentially expressed proteins, studying protein interactions, and elucidating biological pathways and processes.
Both absolute and relative quantitation approaches have their advantages and limitations, and the choice of method depends on the research goals and the specific requirements of the study. In many cases, a combination of both approaches may be used to gain a comprehensive understanding of protein abundance and dynamics in biological systems.
Challenges and considerations in quantitative proteomics
Quantitative proteomics, while powerful, comes with several challenges and considerations that researchers need to address to ensure accurate and reliable results. Some of the key challenges and considerations include:
- Dynamic Range: The dynamic range of protein abundance in biological samples is vast, with highly abundant proteins potentially masking the detection of low-abundance proteins. Techniques such as fractionation and enrichment can help address this issue.
- Sample Complexity: Biological samples are complex mixtures of proteins with varying concentrations, isoforms, and post-translational modifications (PTMs), making accurate quantitation challenging. Sample preparation and fractionation strategies are critical for reducing complexity.
- Quantitation Accuracy and Precision: Quantitative proteomics methods must be accurate and precise to reliably quantify protein abundance. Factors such as sample handling, instrument variability, and data analysis can impact accuracy and precision.
- Normalization: Proper normalization is essential for comparing protein abundance between samples. Normalization strategies should account for variations in sample loading, protein digestion efficiency, and instrument response.
- Protein Isoforms and PTMs: Proteins can exist in multiple isoforms and undergo various PTMs, leading to increased complexity in quantitation. Specialized techniques and databases are needed to account for isoforms and PTMs.
- Data Analysis and Bioinformatics: Analyzing quantitative proteomics data requires sophisticated bioinformatics tools and algorithms to process MS data, identify proteins, quantify their abundance, and perform statistical analysis. Proper data validation and statistical methods are crucial for robust results.
- Quantitation Standards and Controls: The use of quantitation standards and controls is essential for ensuring the accuracy and reliability of quantitative proteomics data. Isotopically labeled standards and internal controls can help validate quantitation results.
- Reproducibility and Replicability: Quantitative proteomics experiments should be reproducible and replicable to ensure the reliability of the results. Proper experimental design, sample preparation, and data analysis practices are essential for achieving reproducibility.
- Quality Control: Implementing quality control measures throughout the proteomics workflow is crucial for identifying and addressing potential sources of error. Regular calibration of instruments, use of quality control samples, and monitoring of data quality are important for maintaining high data quality.
- Biological Variability: Biological variability between samples can introduce noise and confound quantitation results. Proper experimental design, including sufficient biological replicates, can help account for biological variability and improve the reliability of results.
Addressing these challenges and considerations requires careful experimental design, method optimization, and data analysis, as well as ongoing advancements in technology and methodology in the field of quantitative proteomics.
Label-Free Quantitation
Principles of label-free quantitation
Label-free quantitation (LFQ) is a method used in quantitative proteomics to compare the abundance of proteins across different samples without the use of chemical labels. Instead, LFQ relies on the comparison of peptide signal intensities or spectral counts between samples to estimate relative protein abundances. Here are the key principles of label-free quantitation:
- Peptide Signal Intensity: In LFQ, the abundance of a protein is inferred from the intensity of the peptides derived from that protein in mass spectrometry (MS) analyses. The more abundant a protein is in a sample, the higher the intensity of its peptides in the MS spectra.
- Retention Time Alignment: To accurately compare peptide intensities between samples, retention time alignment is performed to ensure that peptides with the same sequence are aligned across different runs. This is crucial for accurate quantitation.
- Normalization: Normalization is essential in LFQ to correct for technical variation between samples, such as differences in sample loading or instrument performance. Common normalization methods include total peptide amount normalization, median normalization, and normalization to internal standards.
- Peptide and Protein Inference: Peptides detected in MS analyses are matched to proteins using bioinformatics tools. Peptides that are unique to a protein or are shared with a limited number of proteins are used for quantitation to avoid ambiguity.
- Data Analysis: LFQ data analysis involves processing raw MS data to identify peptides and quantify their abundance. Statistical methods are then applied to compare protein abundances between samples and identify differentially expressed proteins.
- Advantages:
- Label-free quantitation is cost-effective since it does not require the use of isotopic labels.
- It allows for the analysis of a large number of samples in a single experiment.
- LFQ is suitable for complex samples where labeling may be challenging.
- Challenges:
- LFQ is generally less accurate and precise compared to label-based quantitation methods.
- Variability in peptide ionization and detection can affect the accuracy of quantitation.
- Data analysis for LFQ can be complex, requiring sophisticated bioinformatics tools and algorithms.
Despite these challenges, label-free quantitation remains a widely used method in quantitative proteomics, particularly for large-scale studies and exploratory research. Ongoing developments in MS technology and data analysis algorithms continue to improve the accuracy and reliability of label-free quantitation methods.
Data acquisition methods: spectral counting, peak intensity
Label-free quantitation (LFQ) is a method used in quantitative proteomics to determine the relative abundance of proteins in complex biological samples without the need for stable isotope labeling. LFQ relies on the measurement of peptide ion intensities or spectral counts across multiple LC-MS/MS runs to estimate protein abundance. Here’s an overview of the principles of LFQ:
Principles of Label-Free Quantitation:
- Peptide Ion Intensity: In LFQ, the abundance of a protein is inferred from the intensity of the peptide ions derived from that protein. The more abundant a protein is, the more peptide ions it will generate, leading to higher intensities in the mass spectrometer.
- Retention Time Alignment: LFQ requires accurate alignment of peptide retention times across multiple LC-MS/MS runs to ensure that the same peptide from different runs is correctly matched. This is crucial for accurate quantitation.
- Data Normalization: Since the absolute intensities of peptide ions can vary between runs due to factors like sample loading and instrument variability, LFQ involves normalization procedures to correct for these differences. Common normalization methods include total ion current (TIC) normalization or median normalization.
- Protein Inference: LFQ relies on the identification of peptides and their assignment to proteins. Peptides are typically identified by matching experimental MS/MS spectra to theoretical spectra generated from protein sequence databases. The peptides are then mapped back to their respective proteins.
- Statistical Analysis: After normalization and protein inference, statistical methods are applied to determine the significant differences in protein abundance between samples or conditions. Common statistical tests include t-tests, ANOVA, or more sophisticated methods for analyzing multiple comparisons.
Advantages of Label-Free Quantitation:
- Cost-Effectiveness: LFQ does not require the use of isotopic labels, which can be expensive.
- Dynamic Range: LFQ can cover a wider dynamic range of protein abundance compared to some labeling methods.
- Flexibility: LFQ can be applied to various types of samples and experimental conditions without the need for specialized labeling protocols.
Challenges and Considerations:
- Quantitation Accuracy: LFQ may suffer from quantitation inaccuracies, especially for low-abundance proteins or in complex samples.
- Data Reproducibility: LFQ requires careful experimental design and data processing to ensure reproducibility across different runs.
- Normalization: Correct normalization is crucial for accurate quantitation in LFQ, and improper normalization can lead to biased results.
- Peptide Identification: Proper peptide identification and assignment to proteins are essential for reliable quantitation in LFQ. Misidentification or ambiguous assignments can lead to errors in quantitation.
In summary, LFQ is a powerful and cost-effective method for relative quantitation in proteomics, but it requires careful experimental design, data processing, and validation to ensure accurate and reliable results.
Data normalization and statistical analysis for label-free quantitation
Data normalization and statistical analysis are crucial steps in label-free quantitation (LFQ) proteomics to ensure accurate and reliable results. Here’s an overview of these steps:
Data Normalization:
- Total Ion Current (TIC) Normalization: One common normalization method in LFQ is to normalize the intensities of all peptides in a sample based on the total ion current. This helps correct for variations in sample loading and instrument performance.
- Median Normalization: Another approach is to normalize the peptide intensities based on the median intensity across all peptides in a sample. This method is robust against outliers and can help correct for systematic biases.
- Normalization Factor Calculation: The normalization factor is calculated for each sample to adjust the peptide intensities. This factor is often calculated based on the median or total ion current of each sample.
- Normalization Across Runs: To compare protein abundances across multiple LC-MS/MS runs, it’s essential to normalize the data across runs. This can be done by aligning the retention times of peptides and then normalizing the intensities based on the aligned peptides.
Statistical Analysis:
- Differential Expression Analysis: After normalization, statistical tests are applied to identify proteins that are significantly differentially expressed between experimental conditions. Common statistical tests include t-tests, ANOVA, or more advanced methods for multiple comparisons.
- Fold Change Calculation: The fold change is calculated as the ratio of the abundance of a protein in one condition to its abundance in another condition. Proteins with a significant fold change are considered differentially expressed.
- False Discovery Rate (FDR) Correction: To account for multiple hypothesis testing, FDR correction methods (e.g., Benjamini-Hochberg procedure) are often applied to control the rate of false positives in the results.
- Visualization: Visualization tools such as volcano plots, heatmaps, and clustering techniques are used to visualize the results of the statistical analysis and identify patterns in the data.
- Biological Interpretation: Finally, the differentially expressed proteins are interpreted in the context of the biological question being addressed, considering their known functions, pathways, and interactions.
Overall, proper data normalization and statistical analysis are critical for obtaining reliable and biologically meaningful results in label-free quantitation proteomics studies. These steps help ensure that any observed differences in protein abundance are not due to technical variations but are reflective of true biological differences.
Stable Isotope Labeling Techniques
Principles of stable isotope labeling: SILAC, iTRAQ, TMT
Stable isotope labeling is a method used in quantitative proteomics to measure the relative abundance of proteins between different samples. It involves introducing isotopically labeled forms of amino acids or peptides into proteins, allowing for accurate quantification using mass spectrometry. Three common stable isotope labeling techniques are SILAC (Stable Isotope Labeling by Amino acids in Cell culture), iTRAQ (Isobaric Tags for Relative and Absolute Quantitation), and TMT (Tandem Mass Tags). Here’s an overview of each technique:
- SILAC (Stable Isotope Labeling by Amino acids in Cell culture):
- Principle: SILAC involves culturing cells in media containing isotopically labeled amino acids (e.g., heavy lysine or arginine) for several cell divisions. As cells divide, the labeled amino acids are incorporated into newly synthesized proteins, leading to a complete labeling of the proteome.
- Quantitation: After labeling, cells from different experimental conditions are combined, lysed, and subjected to proteomic analysis. The relative abundance of proteins between samples is determined by comparing the intensities of peptide pairs (labeled and unlabeled) in the mass spectra.
- Advantages: SILAC provides accurate and precise quantitation, high reproducibility, and minimal sample handling, making it suitable for studying dynamic changes in protein expression.
- iTRAQ (Isobaric Tags for Relative and Absolute Quantitation):
- Principle: iTRAQ involves labeling peptides from different samples with isobaric tags, which are tags that have the same mass but different isotopic compositions. Each tag contains a reporter group that is released during fragmentation in the mass spectrometer, allowing for quantitation of the relative abundance of peptides.
- Quantitation: After labeling, peptides from different samples are combined, fractionated, and analyzed by LC-MS/MS. The relative abundance of peptides is determined by comparing the intensities of reporter ions in the mass spectra.
- Advantages: iTRAQ enables multiplexing of up to 8 samples in a single experiment, providing high-throughput quantitation of complex samples.
- TMT (Tandem Mass Tags):
- Principle: Similar to iTRAQ, TMT also uses isobaric tags to label peptides from different samples. TMT allows for multiplexing of up to 16 samples in a single experiment.
- Quantitation: After labeling, peptides from different samples are combined, fractionated, and analyzed by LC-MS/MS. The relative abundance of peptides is determined by comparing the intensities of reporter ions in the mass spectra.
- Advantages: TMT provides high-throughput quantitation and allows for the analysis of a large number of samples in a single experiment.
In summary, stable isotope labeling techniques such as SILAC, iTRAQ, and TMT are powerful tools for quantitative proteomics, providing accurate and reproducible quantitation of protein expression in complex biological samples. Each technique has its advantages and limitations, and the choice of method depends on the specific experimental requirements and research goals.
Sample preparation and labeling protocols
Sample preparation and labeling protocols are critical steps in stable isotope labeling techniques such as SILAC, iTRAQ, and TMT in quantitative proteomics. These protocols ensure that samples are properly prepared, labeled, and processed for accurate quantitation using mass spectrometry. Here’s an overview of sample preparation and labeling protocols for each technique:
- SILAC (Stable Isotope Labeling by Amino acids in Cell culture):
- Sample Preparation:
- Cells are cultured in media containing either light (unlabeled) or heavy (isotopically labeled) forms of essential amino acids (e.g., lysine or arginine) for several cell divisions.
- After labeling, cells are harvested, lysed, and proteins are extracted using standard methods.
- Labeling Protocol:
- Cells are typically cultured for at least 5-7 cell divisions to ensure complete labeling of the proteome.
- The ratio of heavy to light amino acids in the media determines the labeling efficiency and should be optimized for each cell type.
- Considerations:
- Careful control of labeling conditions and media composition is essential to ensure uniform labeling across samples.
- Sample Preparation:
- iTRAQ (Isobaric Tags for Relative and Absolute Quantitation):
- Sample Preparation:
- Proteins from different samples are digested into peptides using a protease (e.g., trypsin) to generate peptides for labeling.
- Labeling Protocol:
- Peptides from different samples are labeled with iTRAQ reagents according to the manufacturer’s instructions.
- Each iTRAQ reagent contains a specific mass reporter group that is released during MS/MS fragmentation for quantitation.
- Considerations:
- Careful optimization of labeling efficiency and reaction conditions is necessary to ensure complete labeling and minimal labeling bias.
- Sample Preparation:
- TMT (Tandem Mass Tags):
- Sample Preparation:
- Similar to iTRAQ, proteins from different samples are digested into peptides using a protease.
- Labeling Protocol:
- Peptides from different samples are labeled with TMT reagents according to the manufacturer’s instructions.
- Each TMT reagent contains a specific mass reporter group that is released during MS/MS fragmentation for quantitation.
- Considerations:
- Optimization of labeling efficiency and reaction conditions is crucial for accurate quantitation.
- Sample Preparation:
In summary, sample preparation and labeling protocols for SILAC, iTRAQ, and TMT are critical for accurate and reproducible quantitation in quantitative proteomics. Careful optimization of labeling conditions, reaction parameters, and sample handling is essential to ensure reliable results.
Data analysis strategies for stable isotope labeling experiments
Data analysis for stable isotope labeling experiments such as SILAC, iTRAQ, and TMT involves several key steps to process and interpret the mass spectrometry data for quantitation. Here’s an overview of the data analysis strategies for each technique:
- SILAC (Stable Isotope Labeling by Amino acids in Cell culture):
- Data Processing:
- Raw mass spectrometry data are processed using proteomics software (e.g., MaxQuant, Proteome Discoverer) to identify peptides and proteins.
- SILAC pairs (light and heavy-labeled peptides) are identified, and their intensities are extracted from the MS data.
- Quantitation:
- The ratio of heavy to light peptide intensities is calculated for each SILAC pair, representing the relative abundance of the protein.
- Statistical methods (e.g., t-tests, ANOVA) are applied to determine significant differences in protein abundance between samples.
- Considerations:
- Normalization methods are used to correct for variations in labeling efficiency and sample loading.
- Data Processing:
- iTRAQ (Isobaric Tags for Relative and Absolute Quantitation):
- Data Processing:
- Raw mass spectrometry data are processed to identify peptides and reporter ions from iTRAQ-labeled samples.
- Intensities of reporter ions are extracted from the MS data for quantitation.
- Quantitation:
- The relative abundance of peptides is calculated based on the intensities of reporter ions.
- Statistical methods are applied to identify significantly differentially expressed proteins.
- Considerations:
- Normalization methods are used to correct for variations in labeling efficiency and sample handling.
- Data Processing:
- TMT (Tandem Mass Tags):
- Data Processing:
- Raw mass spectrometry data are processed to identify peptides and reporter ions from TMT-labeled samples.
- Intensities of reporter ions are extracted from the MS data for quantitation.
- Quantitation:
- The relative abundance of peptides is calculated based on the intensities of reporter ions.
- Statistical methods are applied to identify significantly differentially expressed proteins.
- Considerations:
- Normalization methods are used to correct for variations in labeling efficiency and sample handling.
- Data Processing:
In summary, data analysis for stable isotope labeling experiments involves processing raw mass spectrometry data, quantifying protein abundance based on labeled peptides or reporter ions, and applying statistical methods to identify differentially expressed proteins. Careful normalization and quality control are essential for accurate and reliable quantitation.
Advanced Quantitative Proteomics Methods
Targeted proteomics approaches: selected reaction monitoring (SRM), parallel reaction monitoring (PRM)
Targeted proteomics approaches such as Selected Reaction Monitoring (SRM) and Parallel Reaction Monitoring (PRM) are powerful methods for quantifying specific proteins or peptides in complex biological samples. These techniques offer higher sensitivity, specificity, and quantitative accuracy compared to traditional shotgun proteomics methods. Here’s an overview of SRM and PRM:
- Selected Reaction Monitoring (SRM):
- Principle: SRM involves the selective detection of predefined target peptides based on their mass-to-charge ratio (m/z) and fragmentation pattern.
- Workflow:
- Predefined target peptides (transitions) are selected based on their unique m/z values and fragmentation patterns using prior knowledge or discovery-based proteomics.
- The mass spectrometer is programmed to selectively monitor these transitions during the analysis.
- The intensity of the transitions is used to quantify the abundance of the target peptides.
- Advantages:
- High sensitivity and specificity: SRM can detect low-abundance peptides in complex samples with high specificity.
- Quantitative accuracy: SRM provides accurate and reproducible quantitation over a wide dynamic range.
- Multiplexing: SRM can monitor multiple peptides simultaneously, enabling the quantitation of multiple proteins in a single experiment.
- Parallel Reaction Monitoring (PRM):
- Principle: PRM is similar to SRM but uses high-resolution mass spectrometry (e.g., Orbitrap) to monitor all fragment ions of selected precursor ions simultaneously.
- Workflow:
- Predefined target peptides are selected based on their m/z values and fragmentation patterns.
- The mass spectrometer is used to isolate the precursor ions and fragment them, recording the full MS/MS spectra.
- Quantification is performed by integrating the peak areas of the fragment ions corresponding to the target peptides.
- Advantages:
- Higher specificity: PRM’s high-resolution MS enables better discrimination between target peptides and background noise.
- Improved quantitation: PRM provides more accurate and reproducible quantitation compared to conventional MS/MS methods.
- Flexibility: PRM allows for retrospective data analysis, as all fragment ions are recorded, enabling the identification and quantification of additional peptides.
Both SRM and PRM are widely used in targeted proteomics for biomarker discovery, validation, and pathway analysis. These techniques offer advantages over traditional shotgun proteomics, especially in terms of sensitivity, specificity, and quantitative accuracy, making them valuable tools in biological and clinical research.
Data-independent acquisition (DIA) methods: SWATH-MS
Targeted proteomics approaches, such as Selected Reaction Monitoring (SRM) and Parallel Reaction Monitoring (PRM), offer highly specific and sensitive quantification of proteins of interest. These methods are particularly useful when analyzing a small set of proteins with known peptide sequences. Here’s an overview of SRM and PRM:
- Selected Reaction Monitoring (SRM):
- Principle: SRM monitors specific precursor-to-fragment ion transitions corresponding to target peptides. This targeted approach provides high selectivity and sensitivity for the quantification of proteins.
- Workflow:
- First, a mass spectrometer selects a specific precursor ion representing the target peptide.
- Next, the selected precursor ion is fragmented, and specific fragment ions are monitored for quantification.
- SRM assays require prior knowledge of the peptide sequence and fragment ions, making them ideal for targeted protein quantification.
- Advantages:
- High specificity and sensitivity.
- Good quantification accuracy and reproducibility.
- Suitable for validating candidate biomarkers and analyzing protein isoforms.
- Parallel Reaction Monitoring (PRM):
- Principle: PRM is a variant of targeted proteomics that allows for the simultaneous monitoring of all fragment ions from a selected precursor ion, providing comprehensive data for quantification.
- Workflow:
- Similar to SRM, a specific precursor ion is selected, fragmented, and all resulting fragment ions are monitored.
- PRM does not require pre-selection of specific fragment ions, making it more suitable for comprehensive and unbiased quantification.
- Advantages:
- Higher selectivity and sensitivity compared to conventional MS methods.
- Allows for the detection and quantification of low-abundance proteins.
- Provides comprehensive data for each targeted peptide, improving quantification accuracy.
Both SRM and PRM are powerful targeted proteomics approaches that offer high specificity and sensitivity for protein quantification. The choice between SRM and PRM depends on the research question, the number of proteins of interest, and the desired level of quantification accuracy and coverage.
Related posts:
AI Revolution in Protein Structure Prediction
A.IProtein Structure Determination and Prediction
proteomicsComprehensive Guide to Proteomics Types: Delving into Expression, Functional, and Structural Proteom...
GuidesMass Spectrometry in Proteomics: A Comprehensive Guide for 2023
GuidesMastering 2D-GE in Proteomics: A Comprehensive Guide to Two-Dimensional Gel Electrophoresis
GuidesUsing AI to Harness the Power of Proteomics and Transcriptomics for Disease Diagnosis
A.IGenerative AI in De Novo Drug Design
A.IProteomics 2024: From Early Foundations to Modern Breakthroughs in Personalized Medicine
proteomicsUnlocking the World of Proteomics Data Analysis: A Student's Guide
GuidesAI-Driven Innovations in Proteomics
A.IGenomics vs. Proteomics: Understanding the Key Differences
genomicsEmerging Trends in Proteomics Analysis
proteomicsProteomics in Drug Discovery: A Revolution in Pharma
proteomicsPersonalized Medicine and Proteomics: The Perfect Pair
proteomicsEssential Imaging Software for Mass Spectrometry and Molecular Visualization
bioinformaticsIntroduction to Protein-Protein Interactions in Neurodegenerative Diseases
proteomics