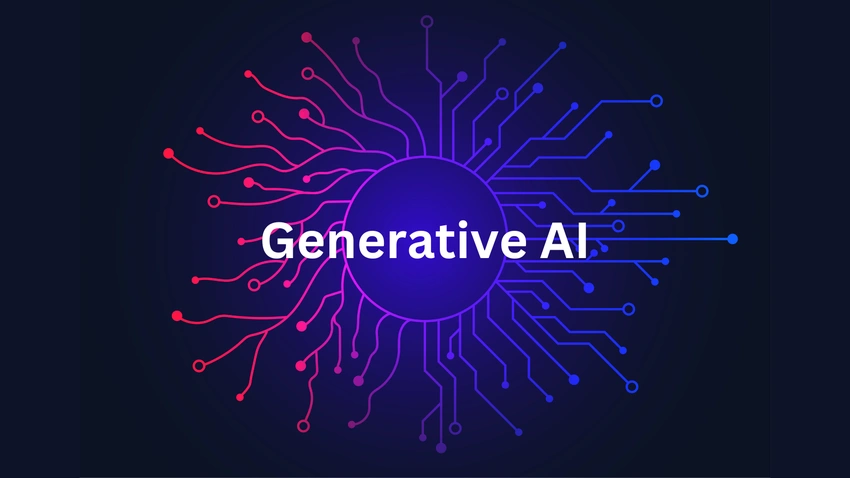
Revolutionizing Bioscience and Healthcare with Generative AI
December 13, 2023This blog post explores how Generative AI, specifically AI-generated text, images, and code, is revolutionizing bioscience, medical science, and related fields. It delves into applications, real-world examples, and ethical considerations, providing a comprehensive overview of the transformative impact of AI in these domains.
I. Introduction
Generative Artificial Intelligence (Generative AI) represents a subset of artificial intelligence focused on creating content, data, or even entire experiences. Unlike traditional AI models that are designed for specific tasks, generative models have the ability to generate new and original content by learning patterns and structures from existing data. This Introduction will provide a brief overview of Generative AI and highlight its significance in bioscience, medical science, and data-centric fields.
A. Generative AI Overview
Generative AI encompasses a class of algorithms and models designed to produce outputs that mimic or resemble human-generated content. These models leverage techniques such as neural networks, deep learning, and probabilistic frameworks to understand and replicate patterns within data. Notable examples of generative models include Generative Adversarial Networks (GANs), Variational Autoencoders (VAEs), and autoregressive models like OpenAI’s GPT (Generative Pre-trained Transformer) series.
B. Significance in Bioscience
- Drug Discovery and Design: Generative AI plays a crucial role in predicting and generating novel molecular structures for drug candidates. By learning from vast datasets of molecular information, these models can propose potential compounds, accelerating the drug discovery process.
- Protein Folding: Understanding protein folding is essential for deciphering biological functions and developing therapeutic interventions. Generative models aid in predicting protein structures, which is a complex task with implications for disease understanding and drug development.
C. Significance in Medical Science
- Medical Imaging: Generative models contribute to the enhancement and generation of medical images. This is particularly valuable in scenarios like magnetic resonance imaging (MRI) or computed tomography (CT) scans, where high-quality images are crucial for accurate diagnoses.
- Disease Prediction and Diagnosis: Generative models analyze patient data to predict disease risks and aid in early diagnosis. By learning patterns from diverse datasets, these models can provide insights into potential health issues, allowing for proactive medical interventions.
D. Significance in Data-Centric Fields
- Data Augmentation: Generative AI is employed to expand datasets, especially in scenarios where obtaining large labeled datasets is challenging. This helps improve the robustness and generalization capabilities of machine learning models.
- Anomaly Detection: In data-centric fields such as cybersecurity, generative models assist in identifying anomalies and potential threats by learning normal patterns within data and flagging deviations.
Generative AI stands at the forefront of innovation, transforming various fields by unleashing the potential to create and understand complex data patterns. Its applications in bioscience, medical science, and data-centric fields hold promise for accelerating research, improving diagnostics, and addressing challenges related to data scarcity. As technology continues to advance, the impact of generative AI is likely to expand, driving further breakthroughs in these critical domains.
II. AI-Generated Text in Bioscience
A. Creative Writing Applications
- Scientific Manuscripts and Papers: AI-generated text plays a significant role in assisting researchers and scientists in drafting scientific manuscripts. These systems, powered by large language models (LLMs), can generate coherent and contextually relevant content based on input data, making the writing process more efficient.
- Idea Generation and Hypothesis Formulation: LLMs can be employed to brainstorm ideas and formulate hypotheses. By analyzing existing research papers and relevant literature, these models can suggest novel research directions, contributing to the creative process in bioscience.
B. Legal Document Generation
- Contracts and Agreements: AI-generated text is increasingly used in the legal field for drafting contracts and agreements. LLMs can generate legally sound and contextually appropriate language, reducing the time and effort required for lawyers to produce accurate legal documents.
- Compliance Documents: In bioscience, where adherence to regulations and compliance is paramount, LLMs can assist in generating compliant documentation. This includes materials related to research ethics, patient consent forms, and regulatory submissions.
C. Role of Large Language Models (LLMs) like GPT-3
- Contextual Understanding: LLMs like GPT-3 excel in understanding context and generating coherent text. In bioscience, this capability is invaluable for producing accurate and contextually relevant content, ensuring that generated text aligns with the intricacies of scientific language.
- Customization and Adaptability: GPT-3’s ability to adapt to specific prompts and contexts allows for tailored content generation. In bioscience, researchers can leverage this feature to obtain text that aligns with their specific research focus, whether it be molecular biology, genetics, or clinical studies.
- Knowledge Integration: GPT-3, having been trained on diverse datasets, can integrate knowledge from various bioscience disciplines. This enables the generation of comprehensive and interdisciplinary content, fostering collaboration and knowledge transfer across different areas of bioscience.
- Ethical Considerations: The use of AI-generated text in bioscience raises ethical considerations, especially in areas like patient communication and consent forms. It is crucial to carefully review and validate AI-generated content to ensure accuracy, compliance with ethical standards, and avoidance of potential biases.
AI-generated text, facilitated by LLMs like GPT-3, is revolutionizing the way content is created in bioscience and legal domains. The creative applications in scientific writing and legal document generation showcase the versatility and adaptability of these models. As these technologies continue to evolve, it is essential to strike a balance between leveraging the efficiency they offer and addressing ethical considerations to maintain the integrity and accuracy of generated content in critical fields like bioscience.
III. AI-Generated Images: Transforming Bioscience and Design
A. DALL-E 2 and Google Imagen Applications
- DALL-E 2: Imaginative Image Synthesis
- Overview: DALL-E 2, an advanced version of the original DALL-E by OpenAI, specializes in generating images from textual descriptions. This has immense applications in bioscience, allowing researchers to visualize and conceptualize complex biological structures or experimental setups.
- Bioscience Applications:
- Molecular Visualization: DALL-E 2 can create visual representations of molecular structures described in research papers, aiding scientists in communicating their findings more effectively.
- Experimental Design: Researchers can use AI-generated images to pre-visualize and plan experiments, enhancing the efficiency of bioscience research.
- Google Imagen: Enhancing Visual Content Creation
- Overview: Google Imagen, a state-of-the-art image synthesis system, leverages generative models to create high-quality images. In bioscience, it can contribute to the generation of educational materials and visually engaging content.
- Applications:
- Educational Materials: Google Imagen can be used to generate illustrations and diagrams for educational materials, making complex bioscience concepts more accessible to students and the general public.
- Scientific Communication: Scientists can utilize AI-generated images to enhance the visual appeal of presentations and papers, effectively conveying their research findings.
B. Implications for Advertising and Marketing
- Visual Content Creation:
- AI-generated images revolutionize the creation of visual content for advertising and marketing purposes. These images can be tailored to specific branding requirements, ensuring unique and eye-catching visuals.
- Personalized Marketing:
- AI can analyze consumer data to generate images that resonate with individual preferences. This personalized approach in advertising can significantly enhance engagement and conversion rates.
- Efficiency in Campaigns:
- Automated image generation reduces the time and resources required for creating marketing materials. This efficiency allows marketers to adapt quickly to changing trends and demands in the market.
C. The Role of AI-Generated Images in Art and Design
- Creativity and Exploration:
- AI-generated images, especially those created by models like DALL-E, provide artists and designers with new avenues for creative exploration. These tools can inspire novel artistic concepts and expand the boundaries of traditional design.
- Collaboration between AI and Artists:
- Artists can collaborate with AI to enhance their creative processes. The combination of human intuition and AI capabilities can lead to the production of unique and thought-provoking artworks.
- Innovative Design Solutions:
- In design fields, AI-generated images can be used to prototype ideas and visualize design concepts quickly. This accelerates the design process and facilitates experimentation with different visual elements.
AI-generated images, as demonstrated by DALL-E 2, Google Imagen, and similar technologies, are transforming bioscience, advertising, marketing, art, and design. The ability to generate visual content based on textual descriptions or data inputs offers unprecedented opportunities for innovation and efficiency in various creative and professional domains. As these technologies continue to evolve, their impact on visual storytelling, communication, and creative expression will likely become even more profound. However, ethical considerations, including authenticity and responsible use, must be carefully addressed to ensure the responsible deployment of AI-generated images in these diverse fields.
IV. AI-Generated Code: Accelerating Software Development
A. Automation of Coding Tasks
- Code Generation:
- AI can automate routine coding tasks, generating code snippets or even entire functions based on input specifications. This accelerates the development process and reduces the burden on programmers for repetitive or boilerplate code.
- Bug Detection and Correction:
- AI-powered tools can analyze code for potential bugs and errors. By identifying issues early in the development cycle, these tools contribute to improved code quality and reduce the time spent on debugging.
B. Efficiency and Speed in Software Development
- Rapid Prototyping:
- AI-generated code facilitates rapid prototyping, allowing developers to quickly test and iterate on ideas. This agility is particularly valuable in bioscience where software prototypes are essential for modeling and simulation.
- Enhanced Productivity:
- Automation of coding tasks enhances overall productivity by freeing up developers to focus on higher-level design and problem-solving. This can lead to faster project completion and more efficient resource utilization.
C. Real-world Applications in Bioscience and Health Informatics
- Bioinformatics Software:
- AI-generated code is instrumental in developing bioinformatics tools for analyzing biological data. These tools can handle complex algorithms, data processing, and visualization, aiding researchers in extracting meaningful insights from large datasets.
- Health Information Systems:
- In health informatics, AI-generated code contributes to the development of efficient and secure information systems. These systems can manage electronic health records, streamline administrative processes, and enhance interoperability between healthcare systems.
- Clinical Decision Support Systems:
- AI-generated code is employed in creating clinical decision support systems. These systems assist healthcare professionals by analyzing patient data and providing evidence-based recommendations, contributing to more informed decision-making.
D. Ethical Considerations and Challenges
- Quality Assurance:
- Despite advancements, AI-generated code may require careful review and testing to ensure it meets quality and security standards. Developers must maintain oversight to prevent potential vulnerabilities.
- Bias in Code Generation:
- The training data used for AI models may introduce biases into the generated code. In bioscience and health informatics, where accuracy and fairness are paramount, addressing and mitigating biases is crucial.
AI-generated code is transforming software development, bringing unprecedented efficiency and speed to the process. In bioscience and health informatics, where the demand for sophisticated software solutions is high, AI accelerates the creation of powerful tools for data analysis, simulation, and decision support. As the technology continues to mature, it is essential for developers and stakeholders in these domains to navigate ethical considerations, ensuring that AI-generated code aligns with industry standards, security protocols, and ethical guidelines. The collaboration between human expertise and AI capabilities holds the key to unlocking new possibilities and advancing software development in critical fields.
V. Applications in Bioscience and Medical Science
A. Omics and the Role of AI-Generated Content
- Genomics:
- AI in Genomic Sequencing: AI is instrumental in genomic sequencing, aiding in the interpretation of vast amounts of genomic data. It identifies patterns, mutations, and potential associations, contributing to a deeper understanding of genetic factors in health and disease.
- AI-Generated Annotations: AI-generated content assists in annotating genomic sequences, providing valuable insights into gene functions, regulatory elements, and potential links to diseases.
- Transcriptomics:
- Differential Gene Expression Analysis: AI facilitates the analysis of transcriptomic data, identifying differentially expressed genes and revealing molecular pathways involved in specific biological processes.
- Predictive Models for Gene Regulation: AI-generated models predict gene regulatory networks, helping researchers understand how genes interact and influence cellular functions.
- Proteomics:
- Protein Structure Prediction: AI aids in predicting protein structures, crucial for understanding their functions and potential therapeutic targets. This accelerates drug discovery and development processes.
- Identification of Post-Translational Modifications: AI assists in identifying post-translational modifications of proteins, offering insights into cellular signaling and disease mechanisms.
B. CRISPR Advancements and AI Collaboration
- CRISPR-Cas9 Genome Editing:
- Target Identification: AI helps identify optimal target sites for CRISPR-Cas9 genome editing, improving the precision and efficiency of gene editing processes.
- Off-Target Prediction: AI models predict potential off-target effects of CRISPR edits, enhancing the safety and accuracy of genome editing applications.
- AI Collaboration in CRISPR Research:
- Data Analysis: AI algorithms analyze vast datasets generated by CRISPR experiments, identifying trends, patterns, and potential therapeutic applications.
- Optimization of CRISPR Experiments: AI guides the optimization of experimental parameters in CRISPR research, reducing the time and resources required for experimentation.
C. Precision Medicine and Health Informatics
- Personalized Treatment Plans:
- Genomic Profiling: AI analyzes individual genomic data to tailor treatment plans based on genetic predispositions, optimizing therapeutic interventions in precision medicine.
- Clinical Decision Support: AI-generated insights aid healthcare providers in making informed decisions, considering patient-specific factors and optimizing treatment strategies.
- Health Informatics:
- Electronic Health Records (EHRs): AI enhances the management and analysis of EHRs, extracting valuable information for research, diagnosis, and treatment planning.
- Predictive Analytics: AI models predict disease risks and treatment outcomes based on a patient’s health history, contributing to proactive and personalized healthcare.
D. Ethical Considerations and Challenges
- Data Privacy and Security:
- Ensuring the privacy and security of sensitive genomic and health data is a critical consideration in the application of AI in bioscience and medical science.
- Interpretability:
- The interpretability of AI-generated insights in complex biological systems remains a challenge. Researchers must carefully validate and interpret the results to ensure accuracy and reliability.
The integration of AI in bioscience and medical science is revolutionizing research, diagnosis, and treatment strategies. From genomics to precision medicine, AI-generated content and insights are advancing our understanding of complex biological systems. Collaboration between AI and cutting-edge technologies like CRISPR-Cas9 is accelerating progress in genetic research and genome editing. As the field continues to evolve, it is essential to navigate ethical considerations and ensure that AI applications align with patient privacy, data security, and the highest standards of scientific integrity. The synergy between AI and bioscience holds immense potential for transformative breakthroughs in personalized medicine and improved healthcare outcomes.
VI. Data Science and Coding Revolution
A. Data-Centric Applications of Generative AI
- Data Augmentation:
- Enhancing Training Datasets: Generative AI is used to augment datasets, generating additional instances of data for training machine learning models. This is particularly valuable in bioscience, where large and diverse datasets are crucial for accurate model training.
- Image Synthesis:
- Generating Realistic Images: Generative models like GANs contribute to the synthesis of realistic images, benefiting applications such as medical imaging in bioscience. These synthesized images can be used to train models for better generalization.
- Natural Language Generation (NLG):
- Automated Report Writing: NLG models generate human-like text, aiding in the automated generation of reports and summaries in bioscience research. This accelerates the analysis and dissemination of research findings.
B. Collaborative Efforts in Bioscience Data Analysis
- Data Sharing and Integration:
- Collaborative Platforms: Generative AI supports collaborative efforts by facilitating data sharing and integration across research teams. It enables the creation of platforms that allow scientists to collectively analyze and interpret complex bioscience data.
- Interdisciplinary Research:
- Bridging Knowledge Gaps: Generative models can assist in interdisciplinary research by generating content that bridges knowledge gaps between different bioscience domains. This promotes collaboration and a more comprehensive understanding of complex biological systems.
C. AI-Generated Code in Driving Innovation
- Automated Model Development:
- Model Architecture Exploration: AI-generated code automates the exploration of various model architectures, accelerating the development of machine learning models for bioscience applications.
- Optimizing Algorithm Implementation:
- Algorithmic Efficiency: AI-generated code optimizes the implementation of algorithms, enhancing the efficiency of data analysis processes in bioscience research.
- Innovation in Experimentation:
- Rapid Prototyping: AI-generated code facilitates rapid prototyping of experimental setups and data analysis pipelines. This accelerates innovation by enabling scientists to test hypotheses and iterate quickly.
D. Ethical Considerations and Challenges
- Bias in Data and Models:
- Ensuring that generative AI applications are free from biases is crucial, especially in bioscience where unbiased data analysis is essential for accurate research outcomes.
- Data Privacy:
- Collaborative data analysis efforts must prioritize data privacy, and measures should be in place to protect sensitive bioscience data shared among research teams.
Generative AI, with its data-centric applications and collaborative potential, is driving a revolution in bioscience data analysis. From augmenting datasets to automating code generation, these technologies enhance the efficiency and innovation in data science. Collaborative efforts are facilitated by generative models, fostering interdisciplinary research and knowledge exchange in bioscience. As AI-generated code continues to advance, its ethical deployment is crucial to maintain the integrity and reliability of results in bioscience research. The synergy between data science and generative AI holds the promise of transformative breakthroughs, shaping the future of bioscience and scientific discovery.
VII. Ethical Considerations in AI-Generated Content
A. Addressing Biases in AI Models
- Bias Identification and Mitigation:
- Rigorous efforts should be made to identify and mitigate biases present in AI models, especially in applications related to bioscience and medical science. This includes biases in training data, model architecture, and the interpretation of results.
- Diverse and Representative Training Data:
- Ensuring that training datasets used for AI models are diverse and representative of various demographics is crucial. In bioscience, this is particularly important to avoid disparities in healthcare research and applications.
- Continuous Monitoring:
- Regular monitoring of AI models in real-world applications is necessary to detect and address emerging biases. Continuous improvement and updates to models should be based on ongoing evaluations of their ethical implications.
B. Ensuring Responsible Use in Bioscience and Medical Applications
- Patient Privacy and Consent:
- Bioscience and medical applications often involve sensitive patient data. Strict adherence to privacy regulations and obtaining informed consent for the use of data in AI applications is essential to uphold ethical standards.
- Transparent Communication:
- Transparent communication about the role of AI in bioscience and medical processes is necessary. Patients, researchers, and healthcare professionals should be informed about the use of AI-generated content, ensuring trust and informed decision-making.
- Human Oversight:
- While AI can automate processes, human oversight is critical, especially in medical decision-making. Final decisions should be made by healthcare professionals, with AI-generated insights serving as supportive tools.
C. Ethical Guidelines for AI-Generated Content
- Accuracy and Reliability:
- AI-generated content in bioscience and medical applications should meet high standards of accuracy and reliability. Transparent reporting of uncertainties and limitations is essential to prevent misleading interpretations.
- Interpretability:
- AI models generating content should be designed for interpretability, allowing users to understand how conclusions are reached. This is particularly important in medical applications where decisions impact patient well-being.
- Fairness and Equity:
- Developers and researchers should strive to create AI models that promote fairness and equity in bioscience and medical contexts. This includes avoiding biases and ensuring that AI-generated insights are applicable across diverse populations.
Ethical considerations play a pivotal role in the responsible development and deployment of AI-generated content, especially in bioscience and medical applications. Addressing biases, ensuring patient privacy, and promoting transparency are essential elements in building trust and maintaining ethical standards. The development of clear ethical guidelines for AI-generated content in these fields is crucial to guide researchers, developers, and healthcare professionals toward responsible and impactful use of artificial intelligence in scientific and medical domains. As technology advances, a proactive and ethical approach is essential to harness the benefits of AI while safeguarding against potential risks and ethical challenges.
VIII. Future Trends and Innovations
A. Predictions for the Continued Evolution of Generative AI
- Advancements in Model Scale:
- Future iterations of generative models are likely to continue growing in scale and complexity. Larger models, potentially surpassing the capabilities of current ones, may lead to improved performance and the generation of more intricate and contextually accurate content.
- Improved Fine-Tuning and Customization:
- The ability to fine-tune and customize generative models for specific domains or tasks will likely improve. This could enable more precise and tailored applications in fields like bioscience, healthcare, and other specialized areas.
- Multimodal Capabilities:
- The integration of multimodal capabilities, where models can handle and generate content across different modalities such as text, images, and possibly even more complex data types, is anticipated. This could further enhance the versatility of generative AI.
B. Emerging Technologies Shaping Bioscience and Healthcare
- Quantum Computing:
- Quantum computing holds the potential to revolutionize computational tasks in bioscience and healthcare. It may significantly accelerate complex simulations, drug discovery processes, and optimization problems in genomics.
- Augmented Reality (AR) and Virtual Reality (VR):
- AR and VR technologies may find increased applications in medical training, surgery simulations, and patient education. These immersive technologies can enhance visualization and understanding of complex biological processes.
- Blockchain for Healthcare Data Security:
- Blockchain technology may play a crucial role in securing healthcare data. Its decentralized and tamper-resistant nature could improve data integrity, privacy, and the secure sharing of medical information.
C. Potential Societal Impacts and Implications
- Ethical Considerations and Regulation:
- As generative AI becomes more sophisticated, there will be a need for robust ethical frameworks and regulations. Addressing issues such as bias, privacy, and responsible use will become even more critical to prevent unintended consequences.
- Job Displacement and Skill Shifts:
- The widespread adoption of AI, including generative models, may lead to shifts in job requirements and responsibilities. It is essential to proactively address potential job displacement and invest in retraining and upskilling the workforce.
- Increased Accessibility to Healthcare:
- The integration of advanced technologies in healthcare, facilitated by AI and other emerging technologies, may lead to improved accessibility and affordability of healthcare services. Telemedicine, remote diagnostics, and AI-driven personalized medicine could benefit a broader population.
- Accelerated Research and Innovation:
- The synergy between generative AI and other emerging technologies may accelerate research and innovation in bioscience and healthcare. This could lead to breakthroughs in drug discovery, personalized medicine, and the understanding of complex biological systems.
The future of generative AI holds exciting possibilities, with continued advancements in model capabilities, customization, and multimodal applications. The convergence of AI with emerging technologies like quantum computing, AR/VR, and blockchain is poised to reshape the landscape of bioscience and healthcare. While these innovations offer tremendous potential benefits, it is crucial to navigate ethical considerations, societal impacts, and regulatory frameworks to ensure responsible and equitable deployment. As these technologies unfold, proactive collaboration among scientists, policymakers, and industry stakeholders will be essential to harness their full potential for the betterment of society.
IX. Conclusion
In summary, the transformative role of Generative AI in bioscience is evident across various facets, from creative content generation to data analysis and code development. This technology has demonstrated significant contributions in drug discovery, molecular visualization, and personalized medicine, accelerating the pace of research and innovation.
Key points to recap:
- Creative Applications: Generative AI, exemplified by models like DALL-E and GPT-3, is reshaping creative content generation, from scientific manuscripts to artistic expressions, enhancing communication and understanding in bioscience.
- Data Science Revolution: AI-generated content plays a crucial role in data-centric applications, automating coding tasks, optimizing algorithms, and fostering collaborative efforts in bioscience data analysis.
- Medical Applications: In medical science, Generative AI contributes to personalized treatment plans, clinical decision support, and the optimization of health informatics systems, ushering in a new era of precision medicine.
- Ethical Considerations: Addressing biases, ensuring responsible use, and adhering to ethical guidelines are paramount to harnessing the benefits of Generative AI in bioscience and medical applications while avoiding unintended consequences.
Looking forward, the future of Generative AI in bioscience promises continued evolution, with advancements in model scale, customization, and multimodal capabilities. Emerging technologies like quantum computing and AR/VR are poised to further revolutionize healthcare and research.
Encouraging a positive outlook, the integration of AI in bioscience holds the potential to overcome challenges, drive breakthroughs, and improve healthcare outcomes. The collaboration between human expertise and AI capabilities creates a synergistic force that propels us into a future where scientific discovery is accelerated and healthcare is more personalized and accessible.
As we navigate this evolving intersection of AI and bioscience, the call-to-action is clear: embrace curiosity, explore the possibilities, and actively contribute to the responsible and ethical development of these technologies. Researchers, developers, policymakers, and enthusiasts alike can shape the future of bioscience by leveraging the power of Generative AI to push the boundaries of knowledge and make meaningful advancements for the benefit of humanity. The journey ahead is exciting, and the contributions of individuals in this space will play a vital role in shaping a positive and impactful future.