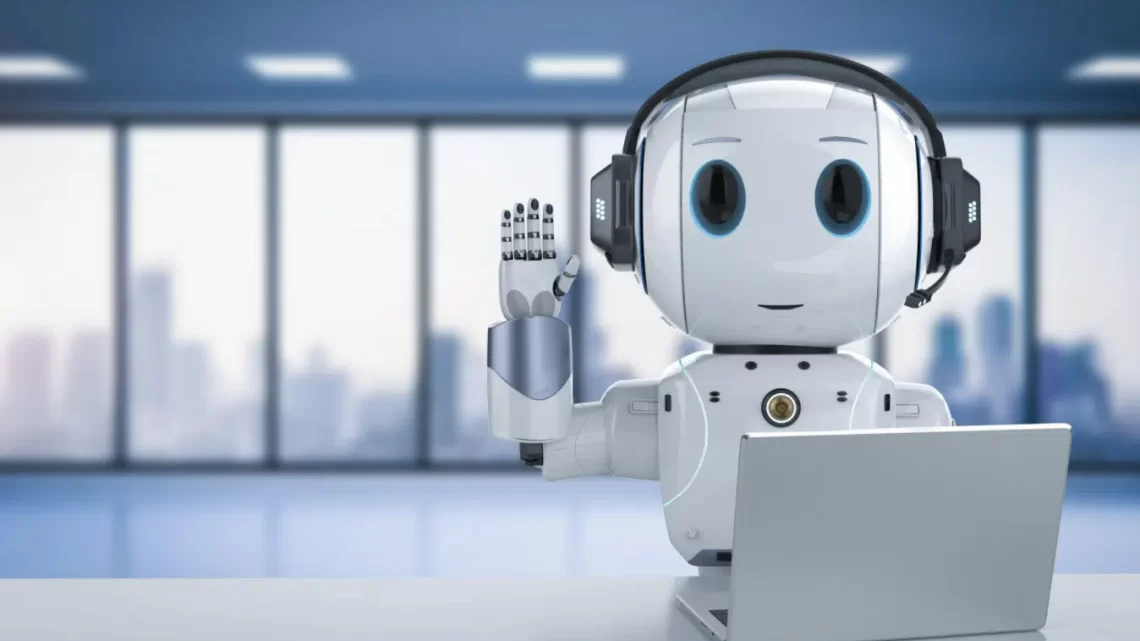
Role of artificial intelligence and machine learning in Bioinformatics
May 17, 2023Artificial intelligence (AI) and machine learning (ML) have had a significant impact on the field of bioinformatics by providing effective tools and methods for analysing complex biological data. AI and ML play the following important functions in bioinformatics:
AI and ML algorithms excel at analysing large-scale biological datasets, including genomics, transcriptomics, proteomics, and metabolomics data. These algorithms enable the discovery of biological insights and predictive models by identifying patterns, correlations, and associations within the data. ML algorithms are capable of classifying samples, identifying biomarkers, predicting protein structures, and identifying genetic variants.
AI and ML have revolutionised sequence analysis tasks, including sequence alignment, motif discovery, and variant calling. Hidden Markov Models (HMMs), Support Vector Machines (SVMs), and deep learning models including convolutional neural networks (CNNs) and recurrent neural networks (RNNs) are utilised to analyse DNA and protein sequences, predict functional elements, annotate genes, and identify sequence variants.
Genomic Medicine and Precision Medicine: AI and ML play a crucial role in genomic medicine by integrating genomic data with clinical data to enhance disease diagnosis, prognosis, and treatment. Models based on machine learning can predict disease risk, stratify patients into subgroups, guide personalised treatment decisions, and identify potential drug targets. Natural language processing (NLP) and other AI techniques aid in the extraction of pertinent information from medical literature and electronic health records.
AI and ML have revolutionised drug discovery by accelerating the identification of potential drug candidates and the repurposing of existing medications for new indications. ML models are capable of analysing vast chemical libraries, predicting drug-target interactions, and ranking compounds for further experimental validation. De novo drug design has utilised generative models, such as generative adversarial networks (GANs) and variational autoencoders (VAEs).
Protein Structure Prediction and Folding: AI and ML have made substantial contributions to protein structure prediction and folding, thereby resolving the age-old “protein folding problem.” The accuracy of deep learning models, such as recurrent and graph neural networks, in predicting protein structures from amino acid sequences is remarkable. These procedures contribute to the comprehension of protein functions, protein-ligand interactions, and drug design.
Biomedical images, such as microscopy images, medical imaging, and histopathology images, are analysed using AI and ML algorithms. Deep learning-based approaches, such as CNNs and convolutional neural networks with attention mechanisms, enable automated image segmentation, object detection, classification, and feature extraction, resulting in enhanced disease diagnosis and prognosis.
Systems Biology and Network Analysis: Using AI and ML techniques, biological networks such as gene regulatory networks, protein-protein interaction networks, and metabolic networks are modelled and analysed. These models help to clarify complex biological processes, identify key regulators, and comprehend behaviour at the system level. Network inference and pathway analysis are aided by ML algorithms like Bayesian networks, random forests, and deep learning models.
The integration of AI and ML with bioinformatics advances our comprehension of biological systems, enables more accurate predictions, and accelerates the development of personalised medicine and biotechnology applications. To assure the responsible and ethical application of AI and ML in bioinformatics, it is essential to address challenges such as data quality, interpretability, and ethical considerations.
