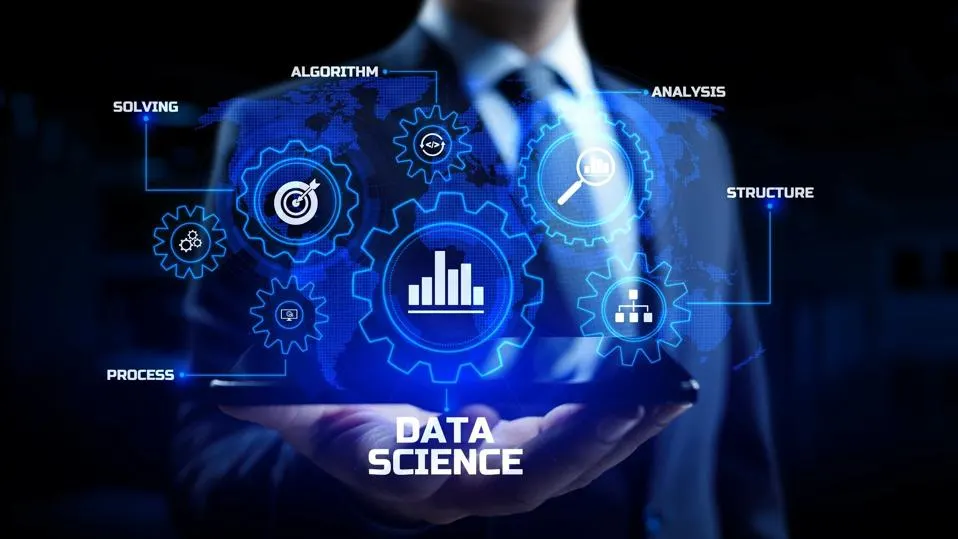
The Intersection of Data Science and Bioinformatics: A Revolution in Biological Data Analysis
October 11, 2024Data science and bioinformatics, although emerging as distinct fields, have increasingly intertwined due to the common challenge they face: managing and analyzing vast amounts of complex data. Bioinformatics, a multidisciplinary science at the intersection of biology, computer science, mathematics, and statistics, focuses on understanding biological phenomena using computational methods. In contrast, data science is a more generalized discipline that seeks to extract insights from structured and unstructured data across various fields, including finance, healthcare, engineering, and social sciences. Despite these differences in focus, the exponential growth of biological data—primarily driven by advancements in next-generation sequencing (NGS) and other high-throughput technologies—has made the integration of data science into bioinformatics not only natural but essential.
Table of Contents
The Emergence of Bioinformatics and Data Science
Bioinformatics began as a response to the rapid accumulation of biological data in the early 1990s. With the sequencing of genomes, such as the Human Genome Project, bioinformatics tools were created to store, process, and analyze vast biological datasets. Initially, the tools developed for bioinformatics were domain-specific, meaning they were designed to address biological problems like gene annotation, protein structure prediction, and phylogenetic analysis. The advent of high-throughput techniques, such as RNA sequencing (RNA-seq), proteomics, and metabolomics, further fueled the need for sophisticated computational solutions.
On the other hand, data science evolved from a mix of disciplines, such as statistics, computer science, and machine learning, primarily focusing on deriving actionable insights from data. Unlike traditional statistics, data science emphasizes the importance of handling large datasets, extracting patterns, and building predictive models. It was not until the early 21st century that data science began to be applied extensively to bioinformatics problems.
The two fields began to merge as the scale of biological data surpassed the capacity of traditional bioinformatics tools. Genomic and proteomic datasets often exceed petabytes, requiring the storage, processing, and extraction capabilities that only modern data science algorithms and technologies can provide. This convergence has since led to a range of developments in both fields.
The Integration of Data Science into Bioinformatics
Today, data science plays an integral role in nearly all aspects of bioinformatics, enhancing the capability to interpret complex biological systems. Some of the most prominent areas where data science impacts bioinformatics include:
1. Genome Sequencing and Annotation
The analysis of genomic data involves vast quantities of DNA sequences. Data science provides the machine learning algorithms, data-mining techniques, and computational power necessary to map and annotate genomes quickly and accurately. Automated systems help identify genetic variants and mutations that can be linked to diseases or evolutionary traits. For example, machine learning models can predict functional regions of the genome, such as coding regions or regulatory elements, and help in detecting disease-causing mutations, paving the way for advances in precision medicine.
2. Phylogenetics and Comparative Genomics
Through data science techniques, bioinformatics can handle comparative genomics and phylogenetics—analyzing the evolutionary relationships between species. This involves constructing phylogenetic trees and identifying genetic similarities or differences. Data science models not only expedite this process but also increase the accuracy of predictions, allowing biologists to trace evolutionary events more precisely. Advanced algorithms allow researchers to study genetic drift, gene flow, and evolutionary bottlenecks, providing deeper insights into the genetic evolution of species.
3. Protein Structure Prediction
One of the most challenging problems in bioinformatics is understanding protein folding and predicting protein structures. Data science, especially with the advent of deep learning models like AlphaFold, has revolutionized this area. These models learn from vast datasets of known protein structures and predict the three-dimensional conformation of proteins, critical for drug design and understanding protein functionality in biological processes.
4. Transcriptomics and Gene Expression Analysis
High-throughput transcriptomics technologies, such as RNA-seq, generate massive datasets of gene expression. Data science enables the analysis of these datasets to identify which genes are active, their regulation patterns, and their associations with diseases or developmental stages. Techniques such as clustering algorithms, dimensionality reduction, and statistical modeling have made it possible to extract meaningful biological signals from noisy data.
5. Metagenomics
Data science also plays a crucial role in metagenomics, the study of microbial communities in diverse environments. Machine learning algorithms analyze complex microbial datasets to explore community structure, functional capabilities, and interactions between microbial species and their environments. These insights have significant implications for understanding microbiomes, such as the human gut microbiome, and their role in health and disease.
Data Science’s Major Role in Bioinformatics
The impact of data science in bioinformatics is undeniable, and its major role can be summarized as follows:
- Handling Big Data: Modern biological research produces large datasets that require scalable storage and processing solutions. Data science provides tools like Hadoop and Spark to manage and analyze large-scale biological data.
- Machine Learning for Predictive Models: Machine learning, a core element of data science, enables the creation of predictive models that assist in drug discovery, gene function prediction, and the identification of disease biomarkers. These models often outperform traditional statistical approaches in complex biological datasets.
- Data Integration: Biological research often involves integrating data from multiple sources—genomic, proteomic, transcriptomic, and clinical. Data science facilitates this integration, allowing for comprehensive analyses that consider the multi-layered nature of biological systems.
- Data Visualization: One of the strengths of data science is its ability to visualize complex data. Visualization tools enable bioinformaticians to identify patterns and relationships in their data that may not be immediately apparent, helping drive new hypotheses and discoveries.
The Future of Data Science and Bioinformatics Integration
As data science continues to evolve, its integration with bioinformatics will become even more pronounced. Here are some future trends we can expect:
1. AI-Driven Discovery
With the advancement of artificial intelligence (AI) and deep learning, the future of bioinformatics will see AI models automating many aspects of biological research. AI can accelerate drug discovery by simulating molecular interactions and predicting the effects of drug candidates on target proteins. It will also refine personalized medicine, enabling treatments tailored to an individual’s genetic makeup, lifestyle, and disease history.
2. Personalized and Precision Medicine
The integration of genomic, transcriptomic, and clinical data through data science will revolutionize personalized medicine. Machine learning models will predict disease risks and therapeutic outcomes for individuals based on their unique genetic profiles. Data science will enable clinicians to tailor interventions precisely, offering more effective treatments and minimizing adverse effects.
3. Multi-Omics and Systems Biology
The future of biological research lies in the integration of multi-omics data, where genomics, proteomics, metabolomics, and other biological layers are analyzed together. Data science will play a central role in developing systems biology approaches that analyze these multiple data types simultaneously, providing a holistic view of cellular processes and disease mechanisms.
4. Ethics and Data Security
As data science and bioinformatics increasingly handle sensitive health and genetic data, ethical concerns related to privacy, consent, and data ownership will intensify. Ensuring data security and ethical standards will become a major focus in future bioinformatics research.
5. Cloud Computing and Edge Analytics
Cloud computing has already transformed how biological data is stored and analyzed. The future will see an expansion of edge analytics, where data is processed closer to its collection point. This will be critical for real-time applications, such as monitoring disease outbreaks or analyzing genomic data in clinical settings.
Conclusion
In conclusion, the convergence of data science and bioinformatics represents one of the most exciting frontiers in modern science. While both fields started separately, the explosion of biological data necessitated their integration, making data science indispensable for bioinformatics research. This integration has already revolutionized genomics, proteomics, transcriptomics, and drug discovery, and the future holds even more promise. As AI, machine learning, and cloud computing continue to advance, the union of these two fields will undoubtedly lead to groundbreaking discoveries, revolutionizing medicine, agriculture, and biotechnology in the years to come.