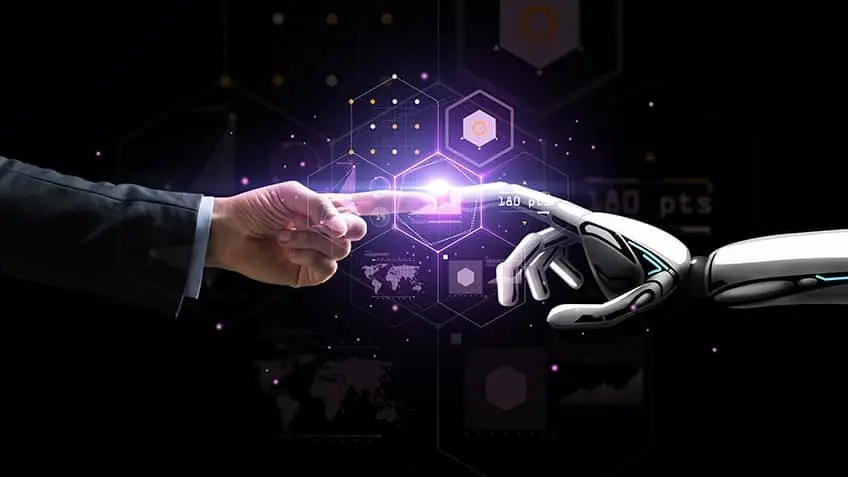
10 Key Trends in AI and Machine Learning for 2024
March 5, 2024Following the introduction of ChatGPT in November 2022, 2023 marked a significant milestone in artificial intelligence. The advancements made over the past year, ranging from a thriving open-source ecosystem to sophisticated multimodal models, have set the stage for substantial progress in AI.
While generative AI continues to captivate the technology industry, there is a growing sense of nuance and maturity as organizations pivot from experimental to practical applications. This year’s trends underscore a deepening sophistication and prudence in AI development and deployment strategies, with a focus on ethics, safety, and the evolving regulatory environment.
Here are the top 10 trends in AI and machine learning to watch for in 2024
Multimodal AI
Multimodal AI extends beyond traditional single-mode data processing by incorporating various types of input, such as text, images, and sound. This approach mirrors human abilities to process diverse sensory information.
During a November 2023 presentation at the EmTech MIT conference, Mark Chen, head of frontiers research at OpenAI, highlighted the importance of multimodal interfaces. He stated, “The world’s interfaces are multimodal. We aim for our models to see and hear as we do, and to generate content that resonates with more than one of our senses.”
OpenAI’s GPT-4 model exemplifies multimodal capabilities, allowing it to process visual and audio inputs. Chen illustrated this by describing a scenario where a user takes photos inside a refrigerator and asks ChatGPT to recommend a recipe based on the ingredients in the images. This interaction could also include an audio component if the user utilizes ChatGPT’s voice mode to verbally request the recipe.
While most generative AI projects today are text-centric, Matt Barrington, Americas emerging technologies leader at EY, emphasized the potential of integrating text, images, and video. He explained, “The true potential of these capabilities will emerge when you can integrate text and conversation with images and video, combining all three and applying them across a range of industries.”
Multimodal AI has diverse practical applications. In healthcare, for example, multimodal models can analyze medical images alongside patient history and genetic data to enhance diagnostic precision. Within organizations, these models can empower employees by extending basic design and coding capabilities to individuals without formal training in those fields.
Barrington noted, “I’m terrible at drawing. But now, I can. I have a decent command of language, so… I can tap into a capability like [image generation], and those ideas that I could never put on paper, I can now have AI bring to life.”
Introducing multimodal capabilities can also enhance models by providing them with new data sources to learn from. Chen explained, “As our models become more adept at understanding language and approach the limits of what they can learn solely from text, we aim to expose them to unprocessed inputs from the world so they can interpret the world independently and draw conclusions from sources such as video or audio data.”
Agentic AI
Agentic AI is a significant advancement that shifts AI from reactive to proactive. AI agents are sophisticated systems that exhibit autonomy, proactivity, and the ability to act independently. Unlike traditional AI systems, which primarily respond to user inputs and follow predetermined programming, AI agents are designed to understand their environment, set goals, and act to achieve those objectives without direct human intervention.
For example, in environmental monitoring, an AI agent could be trained to collect data, analyze patterns, and initiate preventive actions in response to hazards such as early signs of a forest fire. Similarly, a financial AI agent could actively manage an investment portfolio using adaptive strategies that react to changing market conditions in real time.
This advancement is highlighted by computer scientist Peter Norvig, a fellow at Stanford’s Human-Centered AI Institute, who noted that while 2023 was focused on interacting with AI, 2024 will showcase agents capable of completing tasks for users, such as making reservations, planning trips, and connecting with other services.
Combining agentic and multimodal AI opens up new possibilities. For instance, an application designed to identify the contents of an uploaded image can now be created without the need to train a separate image recognition model. This integration allows for the no-code development of computer vision applications, similar to how prompting enabled the development of text-based applications.
Open source AI
Developing large language models and other powerful generative AI systems is a costly endeavor, requiring significant compute resources and vast amounts of data. However, leveraging open source models can help developers reduce costs and expand access to AI technologies. Open source AI refers to publicly available models, typically offered for free, that enable organizations and researchers to build upon existing code and contribute to the development of AI tools.
According to GitHub data from the past year, there has been a notable increase in developer engagement with AI, particularly in the field of generative AI. In 2023, generative AI projects made their way into the top 10 most popular projects on the platform for the first time, with projects like Stable Diffusion and AutoGPT attracting thousands of first-time contributors.
At the beginning of the year, open source generative models were limited in number and often lagged behind proprietary options like ChatGPT in terms of performance. However, the landscape expanded significantly throughout 2023, with the introduction of powerful open source alternatives such as Meta’s Llama 2 and Mistral AI’s Mixtral models. This expansion could potentially change the dynamics of the AI landscape in 2024 by providing smaller, less resourced entities with access to advanced AI models and tools that were previously out of reach.
“It gives everyone easy, fairly democratized access, and it’s great for experimentation and exploration,” commented Barrington.
Open source approaches can also promote transparency and ethical development, as the availability of the code allows for greater scrutiny, leading to the identification of biases, bugs, and security vulnerabilities. However, experts have expressed concerns about the potential misuse of open source AI to create disinformation and other harmful content. Additionally, building and maintaining open source projects, especially complex and compute-intensive AI models, can be challenging.
Retrieval-augmented generation
In 2024, AI regulation is becoming a focal point, with laws, policies, and industry frameworks rapidly evolving both in the U.S. and globally. This shift is driven by concerns about ethics and security, particularly regarding deepfakes, AI-generated content, and potential misuse of AI in fraud and manipulation.
The EU’s AI Act, which is close to adoption, would be the world’s first comprehensive AI law. It aims to ban certain uses of AI, impose obligations on developers of high-risk AI systems, and require transparency from companies using generative AI. Noncompliance could lead to significant fines. Additionally, existing regulations like GDPR are also expected to have a significant impact, particularly regarding the right to be forgotten and data erasure in the context of AI systems.
While the U.S. lacks comprehensive federal AI legislation like the EU’s AI Act, recent executive orders and agency actions suggest a growing focus on AI regulation. President Biden’s executive order, for example, mandates safety testing for AI developers and imposes restrictions to protect against risks in engineering dangerous biological materials.
Organizations are advised not to wait for formal regulations to think about compliance. Engaging with clients and proactively addressing potential regulatory requirements can help businesses stay ahead of the curve. With the EU potentially leading the way in AI regulation, other regions, including the U.S., may need to adapt to these new standards.