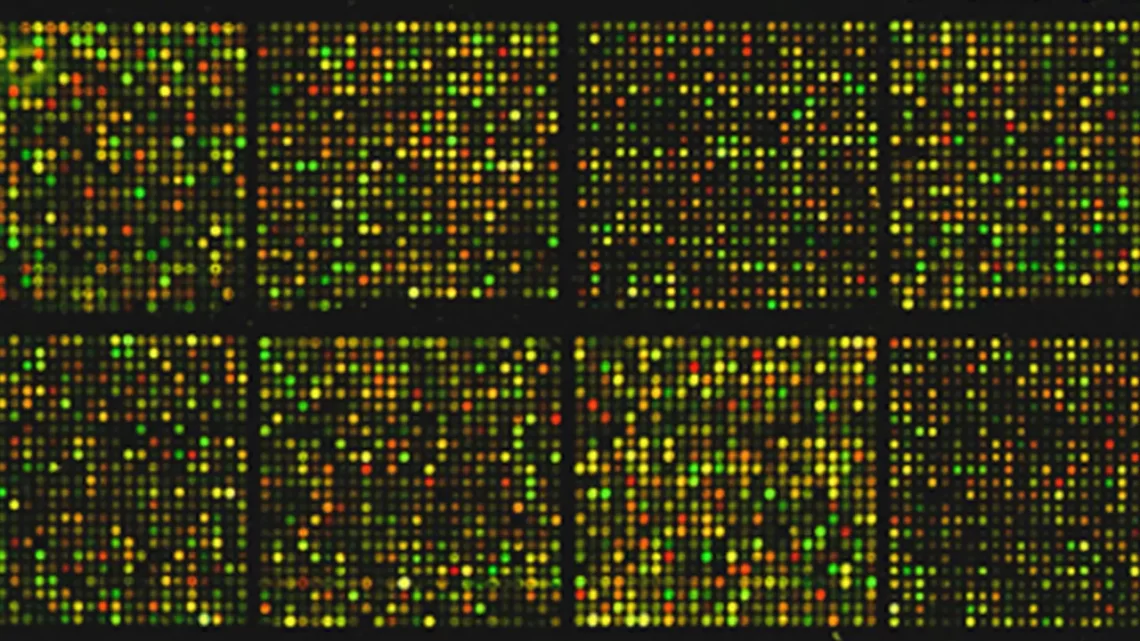
Microarray Bioinformatics in Cancer Research
December 19, 2024Microarrays in Cancer Research: Unveiling New Frontiers in Diagnosis and Treatment
Cancer research has long been at the forefront of biomedical advancements, and the advent of high-throughput technologies such as microarray bioinformatics has significantly contributed to this progress. Microarrays have revolutionized the way scientists analyze gene expression, providing valuable insights into the molecular mechanisms underlying various cancers. In this blog post, we will explore how microarray technology is applied in cancer research, its role in classifying cancers, predicting prognosis, and identifying therapeutic targets, with a particular focus on breast cancer and brain tumors.
Table of Contents
Understanding Microarrays: The Foundation of Cancer Research
Microarrays are a powerful tool used to measure the expression levels of thousands of genes simultaneously. The technology is based on base-pair hybridization, where mRNA is extracted from a sample, converted into complementary RNA (cRNA), and then labeled with a fluorescent dye. This cRNA is then hybridized onto a glass slide containing thousands of unique DNA sequences. The level of mRNA in the sample is determined by the intensity of the fluorescent signal emitted from the dye. A laser scanner quantifies these signals, and bioinformatics software is employed to analyze the data statistically.
This ability to measure the expression of many genes at once enables researchers to study gene activity on a global scale, which is essential in understanding complex diseases like cancer. The application of microarrays in cancer research has led to groundbreaking discoveries in how cancers develop, progress, and respond to treatment.
The Role of Microarrays in Cancer Research
Microarrays have proven invaluable in several aspects of cancer research, including understanding cancer biology, classifying cancers, predicting patient outcomes, and identifying potential therapeutic targets.
1. Understanding Cancer Biology
Microarrays allow researchers to examine gene expression profiles and identify the specific genes and pathways involved in cancer development and progression. By comparing gene expression across different cancer types, researchers can gain insights into the molecular underpinnings of cancer and how tumors evolve. For example, microarrays have helped identify critical genes that drive the development of breast cancer and brain tumors, shedding light on the complex biological processes involved.
2. Classifying Cancers
One of the most significant contributions of microarray technology to cancer research is its ability to classify cancers based on gene expression profiles. Traditional cancer classification relies heavily on histological features, but these methods have limitations. Microarrays provide a more comprehensive view by identifying unique molecular signatures that distinguish different cancer subtypes.
For instance, in breast cancer, microarray analysis has revealed distinct subtypes such as Luminal A, Luminal B, ERBB2, Basal-like, and Normal-like, each of which has different clinical outcomes and responses to therapy. Similarly, microarrays have been used to distinguish medulloblastomas from other brain tumors, providing better diagnostic accuracy.
3. Predicting Prognosis and Treatment Response
Microarray data can also be used to predict how a cancer patient will respond to treatment and the likelihood of metastasis. Gene expression signatures have been developed that can predict metastasis in breast cancer, survival in lung cancer, and treatment response in oligodendrogliomas. These signatures help clinicians make more informed decisions about treatment plans and provide personalized care based on the specific genetic profile of the tumor.
4. Identifying Therapeutic Targets
By identifying genes and pathways that are dysregulated in cancer, microarrays can help pinpoint potential therapeutic targets. For example, research on gliomas has revealed interactions with the MYC oncogene, opening avenues for targeted therapy. In lung cancer, microarray analysis has led to the identification of genes that predict patient survival, offering new possibilities for personalized treatment options.
A table summarizing the timeline of the main events in bioinformatics and microarray technology:
Timeline | Event Description |
---|---|
Early Bioinformatics Era | The field of bioinformatics emerges, utilizing mathematical, statistical, and computational methods to analyze biological data, addressing the need for data management and analysis due to large genomic data. |
Development of Microarray Technology | Microarray technology evolves, moving from profiling a selection of genes to measuring the entire transcriptome using base-pair hybridization principles. |
Microarray Applications in Research | Microarrays are used to explore gene functions, correlate gene expression with experimental conditions and phenotypes, identify biological pathways, drug targets, and develop therapies. |
Microarrays in Cancer Research | Researchers use microarrays to understand cancer-specific gene expression, classifying cancers by organ type, subtype, prognosis, treatment response, or site of metastasis. |
Breast Cancer Research | Perou and Botstein use microarrays to distinguish breast cancer subtypes, and Sørlie associates 5 subtypes with survival data. Molecular profiles linked to known cancer genes like TP53 and BRCA1. |
Breast Cancer Gene Signatures | A 70-gene expression signature predicts metastasis in lymph node-negative breast cancer, and other signatures predict response to Tamoxifen therapy and metastasis. |
Brain Tumor Research | Researchers apply microarray to distinguish medulloblastomas, identify pathways like Sonic Hedgehog, and analyze gliomas, oligodendrogliomas, and glioblastomas. |
Other Oncology Applications | Microarrays improve cancer diagnostics, tracking disease progression, heterogeneity, and response to treatment. Gene sets identify survival predictors in various cancers. |
Acute Leukemia Studies | Golub and other researchers use statistical methods to classify leukemia types, identifying patient subgroups and class predictors. |
This table organizes the major developments and applications of microarray technology in bioinformatics and cancer research.
Specific Applications of Microarrays in Cancer Research
The versatility of microarray technology has allowed it to be applied to various types of cancer, including breast cancer, brain tumors, and leukemia. Below are a few key examples of its impact:
Breast Cancer
Microarray technology has had a profound impact on breast cancer research, particularly in the classification of breast cancer subtypes. Researchers have identified five major subtypes based on gene expression patterns, such as Luminal A and Luminal B, which differ in their prognosis and response to therapy. For example, Luminal A breast cancers, which express estrogen receptors (ER), are associated with a more favorable prognosis. Microarrays have also led to the development of gene signatures that predict the risk of metastasis, helping doctors decide on the most appropriate treatment options.
Brain Tumors
Microarrays have been instrumental in understanding the biology of brain tumors. For instance, microarray analysis has helped identify gene signatures that distinguish medulloblastomas from other brain tumors, providing better diagnostic and prognostic information. Molecular network analysis of gliomas has also uncovered key pathways and novel genes involved in tumor development, opening new possibilities for targeted therapies.
Leukemia
In leukemia research, microarrays have been used to classify different subgroups of acute myeloid leukemia (AML) based on gene expression profiles. These subgroups, which are associated with different chromosomal lesions, have distinct treatment outcomes. Microarrays have also been used to identify class predictors for these subgroups, improving prognostic accuracy and guiding treatment decisions.
The Increasing Importance of Bioinformatics
The explosion of data generated by microarray technology has underscored the importance of bioinformatics in cancer research. Bioinformatics involves the application of computational and statistical methods to analyze large datasets, and it has become an essential tool in managing the complex data generated by microarrays. Advanced software and statistical techniques are used to process and analyze gene expression data, enabling researchers to identify meaningful patterns and correlations that might otherwise go unnoticed.
As cancer research continues to generate vast amounts of data, bioinformatics will play an increasingly important role in interpreting these datasets and translating them into actionable insights for improving patient care. The integration of bioinformatics with microarray technology has enabled researchers to develop predictive models and biomarkers that can guide clinical decision-making, paving the way for more personalized and effective cancer treatments.
Conclusion
Microarray technology has had a transformative impact on cancer research, enabling researchers to study gene expression on a genome-wide scale. By providing insights into cancer biology, tumor classification, prognosis prediction, and therapeutic target identification, microarrays have revolutionized our understanding of cancer and contributed to the development of more personalized treatment strategies. As technology and bioinformatics tools continue to advance, the potential applications of microarrays in cancer research will only continue to expand, ultimately leading to improved outcomes for cancer patients worldwide.
Key Takeaways
- Microarray technology allows for the simultaneous analysis of thousands of genes, providing a global view of gene expression.
- Microarrays have revolutionized cancer research by helping to classify cancers, predict prognosis, and identify therapeutic targets.
- Bioinformatics plays a crucial role in analyzing the large datasets generated by microarray experiments.
- The continued advancement of microarray technology and bioinformatics will lead to further breakthroughs in cancer diagnosis and treatment.
Frequently Asked Questions on Microarray Bioinformatics in Cancer Research
What is bioinformatics, and why is it becoming increasingly important in biological research, particularly in cancer studies?
Bioinformatics is the application of computational and statistical methods to analyze biological data. It has become essential due to the explosion of data generated by high-throughput technologies such as genomic sequencing and microarrays. Traditional, gene-by-gene approaches are no longer sufficient to handle this data volume, making bioinformatics necessary to manage, integrate, analyze, and interpret complex biological information to achieve a global view of cellular functions and disease processes. In cancer research, it helps in understanding complex gene interactions, identifying cancer subtypes, and predicting treatment outcomes.
How does microarray technology work to measure gene expression, and what makes it valuable in cancer research?
Microarray technology measures the activity of gene expression across the entire genome in a single experiment. It works by hybridizing labeled mRNA from a sample to a solid surface containing thousands of unique DNA sequences. The intensity of the resulting fluorescence indicates the level of gene expression, which can then be quantified and statistically analyzed. This technology is particularly valuable in cancer research because it allows for the simultaneous study of numerous genes, enabling a global understanding of gene expression patterns associated with cancer types, subtypes, and treatment responses. It moves beyond the limitations of gene-by-gene analysis to reveal complex biological networks and identify potential targets for therapy and diagnostics.
What are the main applications of microarray technology in cancer research beyond simply measuring gene expression levels?
Microarray technology has several key applications in cancer research, including identifying molecular subtypes of cancer based on gene expression patterns, understanding the biology of specific cancer types by revealing affected pathways, and predicting clinical outcomes such as metastasis and treatment response. This involves not just understanding which genes are turned on and off, but also how these changes relate to cancer phenotypes, patient prognosis, and therapeutic interventions. Ultimately, microarray data is used to develop diagnostic tools, predict patient responses to different treatments, and identify new therapeutic targets.
How has microarray analysis contributed to our understanding of breast cancer, and what specific subtypes have been identified?
Microarray analysis has revolutionized our understanding of breast cancer by enabling the identification of distinct molecular subtypes with different clinical outcomes. Specifically, microarray studies have revealed five major subtypes: Luminal A (ER+, favorable prognosis), Luminal B (ER+, less favorable prognosis), ERBB2 (ERBB2 overexpression, generally ER-negative, poor prognosis), Basal-like (triple-negative, aggressive), and Normal-like (gene expression similar to non-epithelial cells). These classifications have significantly impacted diagnosis and treatment decisions by providing a more nuanced view of the disease, guiding therapeutic decisions, and enhancing our ability to predict metastasis and treatment responses.
What insights have been gained from microarray studies about brain tumors, and how do these insights compare with traditional classification methods?
Microarray studies have provided invaluable insights into brain tumor biology, classifying them into molecularly distinct groups that sometimes diverge from traditional histological classifications. It has identified unique gene signatures for different types of tumors like medulloblastomas and gliomas, revealing biological mechanisms and identifying potential therapeutic targets. These molecular classifications often correlate more strongly with patient survival and treatment response than classical histology. Microarray analysis has also shown that gene expression profiles reflect underlying molecular bases, including mutations, and enable researchers to identify subtypes with similar histology but different prognoses and treatment needs.
How does microarray analysis aid in classifying cancer and what are the two main approaches?
Microarray analysis provides tools for cancer classification, with two main approaches: class discovery and class prediction. Class discovery involves using gene expression data to identify previously unrecognized tumor subtypes, revealing new biological groupings not discernible through traditional means. Class prediction involves assigning tumor samples to defined subclasses based on a subset of significant genes, often correlating molecular profiles with patient outcomes or therapy responses. This allows for the creation of diagnostic and prognostic tools based on gene expression patterns rather than conventional morphology.
What is the difference between class discovery and class prediction in microarray analysis?
In microarray analysis, class discovery is about identifying new, previously unrecognized subtypes within a dataset. It’s an exploratory approach that uses the gene expression data itself to reveal new ways of categorizing the samples based on molecular profiles. Class prediction, on the other hand, uses a defined set of known subtypes based on previously discovered or established data. In class prediction, the goal is to classify new samples to a particular category within the pre-defined classes. Essentially, discovery is about finding new categories and prediction is about fitting samples into established categories.
What are the future implications of applying microarray technologies to clinical practice and what are some limitations?
The merging of robotics, biotechnology, and computer sciences in microarray analysis has greatly impacted how biomedical research is conducted. Microarray technologies offer immense potential in clinical practice through improved diagnostics, the ability to predict disease progression and treatment responses, and the identification of new therapeutic targets based on gene expression profiling. This personalized medicine approach can tailor treatment strategies, increase efficacy, and potentially improve patient survival. Some limitations include challenges in integrating data across different studies, standardizing analysis methods, addressing technical variabilities, and further refining the knowledge on data interpretation to fully exploit the potential of microarrays in personalized medicine.
Glossary
Bioinformatics: The application of computer science and statistics to analyze biological data, such as DNA and RNA sequences.
Gene Expression: The process by which the information encoded in a gene is used to synthesize a functional gene product, typically a protein.
Microarray: A laboratory tool used to measure the expression levels of thousands of genes simultaneously. This is typically done through base-pair hybridization.
cRNA: Complementary RNA, synthesized in the laboratory from extracted mRNA, is labeled with a fluorescent dye to analyze the gene expression.
Base-Pair Hybridization: The process where complementary strands of nucleic acids bind together through hydrogen bonds, essential to microarray technology.
Transcriptome: The complete set of RNA transcripts present in a cell or tissue at a specific time and place.
Oncological Research: A field dedicated to the study of cancer, its causes, and the development of new treatments.
Gene Ontology: A standardized classification system that describes gene functions and biological pathways.
Histology: The study of the microscopic anatomy of tissues.
Molecular Markers: Molecules that can be used to identify specific biological states, such as disease conditions.
Adjuvant Therapy: Additional treatment given after the main treatment (such as surgery) to reduce the risk of cancer recurrence.
Metastasis: The spread of cancer cells from the primary tumor to other parts of the body.
Gliomagenesis: The process of the development of gliomas, a type of brain tumor.
Karyotype: The number and appearance of chromosomes in the cell nuclei of an organism or species.
Class Discovery: The process of identifying previously unrecognized subtypes within a dataset, in this case a set of gene expression profiles.
Class Prediction: The process of using known subtypes to classify an unknown gene expression profile using a previously discovered set of gene expression markers.
Microarray Bioinformatics in Cancer: A Study Guide
Quiz
- What is the primary function of bioinformatics in the context of biological research, and why is it becoming increasingly important?
- Describe the basic principle of microarray technology. What is being measured, and how is this information acquired?
- How has the use of microarray technology shifted the approach to biological research from the “traditional” methods?
- Explain the importance of gene expression in the context of biological research. How does it differ from genetic information contained in DNA?
- What are the two main reasons for using microarray technology in cancer research?
- Describe the five molecular subtypes of breast cancer identified using microarray technology, as well as how the subtypes are differentiated.
- In the study of brain tumors, what has gene expression profiling revealed about medulloblastomas in contrast to primitive neuroectodermal tumors?
- How has microarray technology improved the classification of gliomas beyond traditional histological methods?
- What is the difference between class discovery and class prediction in the context of microarray data analysis?
- Based on the research discussed, how can microarrays potentially improve patient outcomes beyond diagnosis?
Answer Key
- Bioinformatics uses mathematical, statistical, and computational methods to process and analyze biological data. It’s becoming increasingly vital due to the massive amount of data generated by modern technologies like genomic sequencing and microarray chips, enabling researchers to gain a global view of cellular processes.
- The primary principle is base-pair hybridization. Microarrays measure the activity of gene expression by extracting mRNA, creating complimentary RNA (cRNA), labeling it with a fluorescent dye, and hybridizing it to DNA sequences on a slide. The intensity of the dye for each gene represents the gene expression level.
- Traditional research often focused on a gene-by-gene approach in a laboratory environment. Microarray technology enables a global view of gene expression in a single experiment, requiring computational analysis and shifting research into a “virtual lab” environment.
- Gene expression refers to the dynamic process of converting genetic information into RNA and proteins, which varies across tissues and conditions. Unlike the static genetic information contained in DNA, gene expression profiles are dynamic and provide a measure of current cellular activity, such as responses to a disease state.
- Microarray technology is used to understand the underlying biology of cancer types, including their gene mutations and biological pathways, as well as classify cancers according to variables like organ type or a patient’s prognosis, or predict treatment response, and site of metastasis.
- The five breast cancer subtypes are: Luminal A (ER positive, favorable prognosis); Luminal B (ER positive, less favorable prognosis); ERBB2 (overexpresses ERBB2, mostly ER negative, poor prognosis); Basal-like (lacks ER, PR, and ERBB2, aggressive); Normal-like (expresses genes from adipose tissue, also has basal/luminal gene expression). They are differentiated by their expression profiles of specific genes, particularly receptor status and cytokeratins.
- Gene expression profiling revealed that medulloblastomas are biologically distinct from primitive neuroectodermal tumors (PNETs), despite their similar histological characteristics. Specifically, medulloblastomas were linked to cerebellar granule cells and the Sonic Hedgehog pathway.
- Microarray technology enabled the identification of molecular subtypes of gliomas that correlate better with survival and treatment response than the classic, morphology-based subtypes, and also identified the molecular mechanisms underlying their behavior.
- Class discovery involves identifying previously unrecognized tumor subtypes based on gene expression profiles, while class prediction involves using a known set of genes to assign samples to a predefined class.
- Microarrays can be used to predict prognosis, identify patients who would benefit most from adjuvant therapy, predict responses to specific therapies, and identify patients at high risk of disease recurrence or metastasis.
Essay Questions
- Discuss the transformative impact of microarray technology on cancer research, considering both the benefits and limitations in the approach.
- How does the study of gene expression profiles in breast cancer and brain tumors contribute to our understanding of disease biology and inform clinical decision-making?
- Describe the importance of integrating clinical parameters with gene expression profiles in cancer research. How does this improve classification and treatment?
- Analyze the implications of the shift from traditional laboratory methods to bioinformatics approaches in biological research and how does this shift benefit researchers?
- Critically evaluate the recent applications of microarray technology in oncology, specifically how class discovery and class prediction can improve patient care.
Reference
Tao, Z., Shi, A., Li, R., Wang, Y., Wang, X., & Zhao, J. (2017). Microarray bioinformatics in cancer-a review. J buon, 22(4), 838-843.