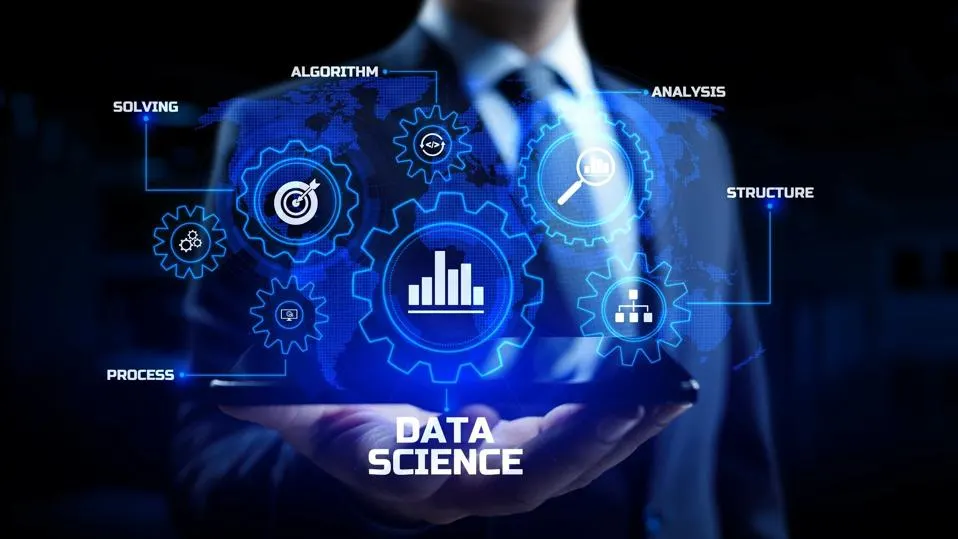
Data Science and Big Data in Drug Discovery
December 27, 2023I. Introduction
A. Significance of Data Science and Big Data in Drug Discovery
In the dynamic landscape of drug discovery, data science and big data have emerged as pivotal drivers of innovation, transforming the traditional paradigms of pharmaceutical research. The significance of these technologies lies in their ability to harness vast amounts of information, analyze complex biological data, and expedite the identification and development of novel therapeutic compounds. Key aspects of their significance include:
- Accelerated Decision-Making:
- Data science facilitates the rapid analysis of diverse datasets, enabling researchers to make informed decisions at various stages of drug discovery. The speed at which insights can be gleaned expedites the overall drug development process.
- Precision Medicine Advancements:
- Big data allows for the integration of diverse datasets, including genomics, proteomics, and patient records. This integration supports the development of personalized and targeted therapies, tailoring treatments to individual genetic makeup and disease characteristics.
- Identification of Novel Drug Targets:
- Data science techniques, such as machine learning and bioinformatics, aid in the identification of novel drug targets by analyzing complex biological networks and uncovering potential points of intervention.
- Prediction of Drug-Drug Interactions:
- Big data analytics assist in predicting potential interactions between drugs, reducing the risk of adverse effects and optimizing drug combinations for enhanced therapeutic efficacy.
- Optimization of Clinical Trial Design:
- Data-driven insights enhance the design and execution of clinical trials, helping researchers identify optimal patient populations, dosage regimens, and biomarkers for effective and efficient trials.
- Efficient Drug Repurposing:
- Data science enables the exploration of existing datasets to identify opportunities for drug repurposing, finding new therapeutic uses for existing compounds and potentially reducing development timelines and costs.
- Integration of Multi-Omics Data:
- The integration of diverse ‘omics’ data, including genomics, transcriptomics, and metabolomics, allows for a comprehensive understanding of disease mechanisms, facilitating the discovery of novel biomarkers and therapeutic targets.
B. Overview of Traditional Drug Discovery Challenges
- High Development Costs:
- Traditional drug discovery is often associated with exorbitant costs, driven by the extensive resources required for experimental research, clinical trials, and regulatory compliance.
- Lengthy Development Timelines:
- The conventional drug development process is time-consuming, typically taking over a decade from target identification to market approval. Delays can occur at various stages, including preclinical research, clinical trials, and regulatory approvals.
- High Attrition Rates:
- Many drug candidates fail to progress beyond preclinical or clinical phases due to efficacy issues, safety concerns, or unforeseen side effects. This high attrition rate contributes to the cost and time challenges in drug development.
- Limited Target Identification:
- Identifying viable drug targets is a complex task, and the limited understanding of disease mechanisms can impede the discovery of suitable targets for therapeutic intervention.
- Inefficiencies in Clinical Trials:
- Traditional clinical trial designs may lack flexibility and adaptability, leading to inefficiencies in patient recruitment, data collection, and overall trial execution.
- Lack of Personalization:
- One-size-fits-all approaches in drug development may not account for individual variations in patient responses. Lack of personalization can result in suboptimal treatment outcomes and adverse reactions.
- Data Silos and Integration Challenges:
- Traditional drug discovery often involves working with fragmented and siloed datasets. Integrating diverse data sources poses challenges, hindering the comprehensive analysis required for effective decision-making.
The introduction of data science and big data into drug discovery aims to address these challenges, offering the potential for more efficient, cost-effective, and personalized approaches to the development of new therapeutic interventions.
II. Current Landscape of Drug Discovery
A. Limitations of Traditional Drug Discovery Methods
Traditional drug discovery methods, while foundational, face several limitations that hinder the efficiency and success of the process. Understanding these challenges is crucial for appreciating the need for transformative approaches:
- High Cost and Resource Intensity:
- Traditional drug discovery is a resource-intensive process that demands significant financial investments. The costs associated with experimental research, clinical trials, and regulatory compliance contribute to the overall high cost of drug development.
- Lengthy Development Timelines:
- The conventional drug development pipeline involves multiple stages, from target identification to clinical trials and regulatory approval. These stages, each with its set of requirements and challenges, contribute to lengthy development timelines, often exceeding a decade.
- High Failure Rates:
- A substantial percentage of drug candidates fail during various stages of development, such as preclinical testing or clinical trials. This high attrition rate is attributed to issues related to efficacy, safety, and unforeseen adverse effects.
- Limited Target Identification:
- Identifying viable drug targets is a complex and time-consuming task. The traditional understanding of disease mechanisms may be limited, impeding the discovery of suitable targets for therapeutic intervention.
- Animal Model Discrepancies:
- Translating findings from preclinical studies in animal models to human clinical trials can be challenging. Differences in biology between species may lead to discrepancies in drug responses, potentially impacting the predictive value of preclinical data.
- Insufficient Personalization:
- Traditional drug development often employs a one-size-fits-all approach, neglecting individual variations in patient responses. Lack of personalization can result in suboptimal treatment outcomes and an increased risk of adverse reactions.
- Data Silos and Fragmented Knowledge:
- Information relevant to drug discovery is often scattered across different research groups, institutions, and publications. Data silos and fragmented knowledge hinder the comprehensive understanding of disease biology and potential drug targets.
B. The Growing Importance of Data-Driven Approaches
- Precision Medicine Paradigm:
- Advanced Analytics for Biomarker Discovery:
- Data-driven analytics, including machine learning and bioinformatics, play a critical role in the discovery of biomarkers. These biomarkers serve as indicators of disease presence, progression, and response to treatment.
- System Biology and Network Pharmacology:
- Data-driven approaches enable the integration of large-scale biological data to construct systems-level models of diseases. System biology and network pharmacology contribute to a holistic understanding of complex biological interactions.
- Target Identification and Validation:
- Data-driven techniques facilitate the identification and validation of potential drug targets. By analyzing biological networks, genetic data, and molecular pathways, researchers can prioritize targets with higher therapeutic potential.
- Predictive Modeling for Drug Efficacy:
- Advanced analytics, including computational models and simulations, enable predictive modeling of drug efficacy. This approach helps researchers anticipate how drugs will interact with biological systems and predict potential outcomes.
- Integration of Real-World Data:
- Real-world data, encompassing patient records, electronic health records, and post-market surveillance data, are increasingly integrated into drug discovery processes. This real-world evidence provides valuable insights into drug effectiveness and safety.
C. The Role of Big Data in Transforming Drug Discovery
- Large-Scale Genomic Data:
- Big data in drug discovery involves the analysis of large-scale genomic datasets. This includes sequencing data from diverse patient populations, aiding in the identification of genetic factors associated with diseases and potential drug responses.
- Omics Data Integration:
- Big data analytics enable the integration of various ‘omics’ data, such as genomics, proteomics, and metabolomics. This integrative approach provides a comprehensive view of the molecular landscape of diseases and facilitates the identification of therapeutic targets.
- High-Throughput Screening:
- Big data technologies support high-throughput screening methods, allowing researchers to analyze large datasets generated from experiments testing the effects of thousands of compounds on biological systems.
- Real-Time Data Analytics:
- The processing of real-time data streams is a key aspect of big data in drug discovery. Real-time analytics provide timely insights during clinical trials, allowing for adaptive trial designs and more informed decision-making.
- Machine Learning for Predictive Modeling:
- Big data and machine learning algorithms contribute to predictive modeling in drug discovery. These models analyze large datasets to predict drug efficacy, identify potential side effects, and optimize treatment regimens.
- Biobanking and Patient Cohort Analysis:
- Big data technologies facilitate the analysis of large biobanks and patient cohorts. This includes studying diverse patient populations to identify genetic variations, biomarkers, and factors influencing drug responses.
- Data Sharing and Collaboration:
- The use of big data encourages collaboration and data sharing across research institutions and pharmaceutical companies. Shared datasets contribute to a collective understanding of diseases and accelerate drug discovery efforts.
In conclusion, the current landscape of drug discovery reflects a transition toward data-driven approaches, underpinned by the growing importance of advanced analytics and big data. These transformative approaches hold the promise of addressing traditional limitations, expediting the drug development process, and ushering in a new era of precision medicine and personalized therapeutic interventions.
III. Key Components of Data Science in Drug Discovery
Data science plays a pivotal role in revolutionizing drug discovery by providing sophisticated tools and methodologies to extract meaningful insights from complex biological data. The key components of data science in drug discovery encompass various stages of the research process:
A. Data Collection and Integration:
- Biological Databases:
- Clinical and Patient Data:
- Aggregating and integrating clinical data from electronic health records, patient registries, and clinical trials to understand disease characteristics, patient demographics, and treatment outcomes.
- Chemical and Structural Databases:
- Accessing databases containing information on chemical structures, compound libraries, and drug-target interactions to identify potential drug candidates and understand their pharmacological profiles.
- Real-World Data Sources:
- Incorporating real-world data sources, such as data from wearable devices, mobile health applications, and patient-reported outcomes, to gain insights into patient behaviors, treatment adherence, and disease progression.
- Omics Data Integration:
- Integrating diverse ‘omics’ data, including genomics, transcriptomics, proteomics, and metabolomics, to create comprehensive molecular profiles for diseases and identify potential therapeutic targets.
- Literature Mining:
- Extracting valuable information from scientific literature through text mining techniques to identify relevant studies, novel findings, and potential targets for drug discovery.
B. Data Analysis and Predictive Modeling:
- Exploratory Data Analysis (EDA):
- Employing EDA techniques to understand the characteristics and patterns within datasets, helping researchers identify trends, outliers, and potential associations.
- Statistical Analysis:
- Conducting statistical analyses to assess the significance of observations, validate hypotheses, and quantify relationships between variables in the context of drug discovery.
- Predictive Modeling for Drug Efficacy:
- Developing predictive models to assess the efficacy of potential drug candidates. Machine learning algorithms are often used to analyze historical data and predict the likely outcomes of new compounds.
- Quantitative Structure-Activity Relationship (QSAR) Modeling:
- Building QSAR models to understand the relationship between chemical structures of compounds and their biological activities, aiding in the design of new drug candidates.
- Network Analysis:
- Applying network analysis techniques to study complex interactions within biological systems, including protein-protein interactions, pathway analysis, and systems biology modeling.
- Text Mining for Data Extraction:
- Using text mining tools to extract structured information from unstructured text data, such as scientific literature, patents, and clinical trial reports, to augment drug discovery knowledge.
C. Machine Learning Algorithms for Drug Discovery:
- Virtual Screening:
- Implementing machine learning algorithms for virtual screening of chemical libraries, predicting the likelihood of compounds binding to specific targets and assessing their potential as drug candidates.
- Target Identification and Validation:
- Leveraging machine learning for the identification and validation of potential drug targets by analyzing biological data, identifying patterns, and prioritizing targets with therapeutic potential.
- Drug-Drug Interaction Prediction:
- Developing models to predict potential interactions between drugs, assessing the risk of adverse effects and optimizing drug combinations for enhanced therapeutic efficacy.
- Patient Stratification:
- Applying machine learning algorithms to stratify patient populations based on molecular profiles, enabling personalized treatment strategies and improving the success rates of clinical trials.
- Adverse Event Prediction:
- Using machine learning to predict potential adverse events associated with drug candidates, allowing researchers to assess safety profiles and make informed decisions during drug development.
- Generative Models for Compound Design:
- Exploring generative models, such as generative adversarial networks (GANs) and variational autoencoders (VAEs), to design novel chemical compounds with desired pharmacological properties.
- Deep Learning for Image Analysis:
- Integrating deep learning algorithms for the analysis of medical images in drug discovery, aiding in the identification of biomarkers, disease patterns, and treatment responses.
By integrating these key components, data science becomes a powerful catalyst in drug discovery, enabling researchers to navigate the complexities of biological systems, make informed decisions, and accelerate the identification and development of novel therapeutics.
V. Applications of Big Data in Drug Discovery
Big data analytics has transformed the landscape of drug discovery by providing unprecedented insights into biological systems, disease mechanisms, and treatment responses. The applications of big data in drug discovery are diverse and impactful, contributing to advancements in precision medicine and accelerating the development of novel therapeutics:
A. Genomic and Proteomic Data Analysis:
- Identification of Genetic Variants:
- Analyzing large-scale genomic data to identify genetic variants associated with diseases. Big data enables the identification of rare and common variants, contributing to a more comprehensive understanding of genetic factors in drug response.
- Pharmacogenomics and Personalized Medicine:
- Utilizing genomic data to identify genetic variations influencing drug metabolism, efficacy, and safety. This information supports the development of personalized medicine approaches, tailoring treatments based on individual genetic profiles.
- Functional Genomics:
- Applying big data analytics to functional genomics data, including transcriptomics and epigenomics, to understand how genes are expressed and regulated in different disease states. This aids in target identification and validation.
- Proteomic Profiling:
- Analyzing large-scale proteomic datasets to identify protein biomarkers associated with diseases or drug responses. This information is crucial for understanding the molecular mechanisms of diseases and developing targeted therapies.
B. Biomarker Discovery Using Big Data:
- Integration of Multi-Omics Data:
- Integrating data from diverse ‘omics’ sources, such as genomics, transcriptomics, proteomics, and metabolomics, to identify comprehensive biomarker profiles associated with diseases or drug responses.
- Predictive Biomarker Identification:
- Using big data analytics to identify predictive biomarkers that can indicate how patients are likely to respond to specific treatments. This information guides patient stratification in clinical trials and enhances treatment outcomes.
- Biomarker Panels for Disease Diagnosis:
- Developing biomarker panels using big data to aid in the early diagnosis and prognosis of diseases. These panels may include a combination of genomic, proteomic, and other molecular markers.
- Dynamic Biomarker Monitoring:
- Implementing big data analytics for dynamic monitoring of biomarker changes over time, providing insights into disease progression, treatment response, and potential resistance mechanisms.
C. Real-World Evidence and Patient Data in Drug Development:
- Patient Data for Targeted Clinical Trials:
- Leveraging real-world patient data, including electronic health records (EHRs) and patient-reported outcomes, to identify suitable patient populations for targeted clinical trials. This enhances the efficiency of trial design and patient recruitment.
- Post-Market Surveillance and Safety Monitoring:
- Utilizing real-world evidence for post-market surveillance to monitor the safety and efficacy of approved drugs in diverse patient populations. Big data analytics enable the detection of potential adverse events and safety signals.
- Treatment Effectiveness in Real-World Settings:
- Analyzing real-world data to assess the effectiveness of treatments in diverse patient populations, beyond the controlled environment of clinical trials. This information helps bridge the gap between clinical trial results and real-world clinical practice.
- Healthcare Utilization and Resource Optimization:
- Analyzing healthcare utilization patterns and resource allocation using big data. This information is valuable for optimizing healthcare resources, identifying areas for improvement, and enhancing the overall efficiency of drug development and healthcare delivery.
- Patient-Centric Drug Development:
- Incorporating patient perspectives and preferences from real-world data to inform drug development strategies. Patient-centric approaches improve the relevance and success of drug development efforts.
- Population Health Insights:
- Using big data analytics to gain insights into population health trends, disease prevalence, and treatment outcomes. This information contributes to public health strategies and informs drug development priorities.
The applications of big data in drug discovery are multifaceted, spanning from the molecular understanding of diseases to the pragmatic considerations of patient-centric drug development. By harnessing the power of big data, researchers can navigate the complexities of drug discovery with unprecedented precision and efficiency.
V. Enhancing Drug Screening and Development
In the realm of drug discovery, the integration of big data and data science methodologies has brought about transformative approaches to enhance drug screening, design, and overall development processes. The utilization of advanced technologies has significantly accelerated the identification of potential drug candidates and streamlined the drug development timeline. Key aspects include:
A. High-throughput Screening with Big Data:
- Comprehensive Compound Libraries:
- Integration of big data allows researchers to curate extensive compound libraries, incorporating chemical structures, bioactivity data, and information from diverse sources. This comprehensive data landscape facilitates the selection of diverse compounds for high-throughput screening.
- Data-Driven Target Selection:
- Leveraging big data analytics to identify and prioritize drug targets based on large-scale biological datasets. This data-driven approach ensures that targets are selected with a higher likelihood of success in subsequent stages of drug development.
- Machine Learning for Hit Identification:
- Implementing machine learning algorithms to analyze high-throughput screening data and identify potential hits or lead compounds. These algorithms can recognize patterns indicative of biological activity, expediting the hit identification process.
- Optimization of Screening Assays:
- Analyzing historical screening data to optimize assay conditions and experimental parameters. This iterative process, guided by big data insights, enhances the accuracy and efficiency of high-throughput screening campaigns.
- Predictive Models for Compound Prioritization:
- Developing predictive models to prioritize compounds based on their likelihood of success in subsequent stages of drug development. These models consider a range of factors, including chemical properties, biological activity, and safety profiles.
B. In Silico Drug Design and Virtual Screening:
- Molecular Dynamics Simulations:
- Utilizing big data in molecular dynamics simulations to study the dynamic behavior of drug candidates at the molecular level. This approach provides insights into the binding interactions, stability, and conformational changes of compounds.
- Quantitative Structure-Activity Relationship (QSAR) Models:
- Implementing QSAR models powered by big data to predict the relationship between chemical structures and biological activities. These models guide the design of new compounds with improved pharmacological properties.
- Generative Models for Compound Design:
- Leveraging generative models, such as generative adversarial networks (GANs) and variational autoencoders (VAEs), for in silico compound design. Big data facilitates the training of these models on diverse chemical datasets to generate novel, structurally diverse compounds.
- Virtual Screening of Compound Libraries:
- Conducting virtual screening of compound libraries against specific targets or biological pathways. Big data enables the analysis of large datasets of chemical structures, improving the accuracy of virtual screening predictions.
- Integration of Cheminformatics Data:
- Integrating cheminformatics data, including information on chemical properties, toxicity, and bioavailability, into in silico drug design processes. This comprehensive approach considers multiple dimensions to guide rational drug design.
C. Accelerating Drug Development Timelines with Data Science:
- Predictive Modeling for Clinical Trial Design:
- Using predictive modeling and data science techniques to optimize clinical trial designs. This includes patient stratification, identification of optimal endpoints, and adaptive trial designs, ultimately accelerating the clinical development process.
- Real-World Evidence for Decision-Making:
- Incorporating real-world evidence, such as data from electronic health records and patient registries, into decision-making processes. This pragmatic approach informs go/no-go decisions, enhances trial designs, and provides insights into the real-world effectiveness of interventions.
- Data-Driven Patient Recruitment:
- Analyzing big data to identify suitable patient populations and recruitment strategies for clinical trials. Data-driven recruitment approaches improve the efficiency of patient enrollment, reducing timelines for trial completion.
- Machine Learning for Drug Safety:
- Implementing machine learning models to predict potential safety issues early in the development process. These models analyze safety-related data to identify signals and mitigate risks before advancing to later stages of development.
- Optimization of Formulation and Manufacturing:
- Applying data science to optimize drug formulation and manufacturing processes. This includes predictive modeling for formulation development and the use of data-driven approaches to enhance manufacturing efficiency.
- Adaptive Licensing and Regulatory Strategies:
- Incorporating data-driven insights into regulatory strategies, including adaptive licensing approaches. Real-time data analytics and predictive modeling contribute to more flexible and efficient regulatory pathways.
In summary, the integration of big data and data science techniques is revolutionizing drug screening and development processes. From high-throughput screening to in silico drug design and the acceleration of development timelines, these approaches are reshaping the landscape of drug discovery, making it more efficient, cost-effective, and informed.
VI. Challenges and Ethical Considerations
The integration of big data in drug discovery comes with various challenges and ethical considerations that need careful attention to ensure responsible and transparent practices. Addressing these challenges is crucial for maintaining the integrity of research and safeguarding the privacy and rights of individuals involved. Key challenges and ethical considerations include:
A. Handling and Managing Large-Scale Datasets:
- Data Quality and Integration:
- Challenge: Managing the quality and integration of diverse datasets from different sources, including biological databases, clinical records, and real-world evidence, poses a significant challenge. Ensuring the accuracy, completeness, and consistency of large-scale datasets is essential for reliable analyses.
- Ethical Consideration: Researchers must transparently disclose the sources and limitations of the data used in their analyses. Misinterpretations or biases in large datasets can lead to erroneous conclusions, emphasizing the need for cautious handling.
- Data Storage and Infrastructure:
- Challenge: Storing and managing massive volumes of data requires robust infrastructure and storage solutions. The scalability and security of data storage systems are essential to prevent data loss, ensure accessibility, and protect against unauthorized access.
- Ethical Consideration: Researchers should implement secure and compliant data storage practices, with a commitment to data preservation and accessibility. Transparent data management practices contribute to reproducibility and data sharing.
- Data Governance and Standardization:
- Challenge: Establishing effective data governance frameworks and standards is challenging, especially when dealing with heterogeneous datasets. Lack of standardized formats and terminologies can hinder data interoperability and collaboration.
- Ethical Consideration: Researchers must advocate for transparent data governance practices, including adherence to standards and the use of open data formats. Promoting data standardization enhances collaboration and facilitates meaningful comparisons across studies.
B. Ensuring Data Privacy and Security in Drug Discovery:
- Patient Confidentiality:
- Challenge: Safeguarding patient confidentiality and preventing the unauthorized re-identification of individuals within large datasets is a complex task. The risk of unintended disclosure increases with the granularity of data, raising concerns about privacy breaches.
- Ethical Consideration: Researchers must prioritize the de-identification and anonymization of patient data to protect individual privacy. Robust privacy-preserving techniques, such as differential privacy, should be implemented to minimize re-identification risks.
- Cybersecurity Threats:
- Challenge: Large-scale datasets are susceptible to cybersecurity threats, including hacking, data breaches, and ransomware attacks. Ensuring the security of sensitive health data is imperative to prevent unauthorized access and protect against data manipulation.
- Ethical Consideration: Researchers and institutions must implement robust cybersecurity measures to safeguard health data. Transparent communication about security measures and proactive disclosure of breaches are ethical imperatives.
- Informed Consent and Data Use:
- Challenge: Obtaining informed consent for the use of patient data in research is challenging, especially when dealing with retrospective analyses of existing datasets. Ensuring that patients are adequately informed about potential uses of their data is crucial.
- Ethical Consideration: Researchers should uphold the principles of informed consent, providing clear and understandable information about data usage. Ethical review boards play a critical role in evaluating the appropriateness of data use and ensuring compliance with ethical standards.
C. Ethical Considerations in the Use of Patient Data:
- Patient Autonomy and Control:
- Challenge: Balancing the benefits of research with the rights of patients to control their data poses a challenge. Patients may have varying levels of comfort with data sharing, and respecting their autonomy is essential.
- Ethical Consideration: Researchers should empower patients with control over their data, allowing them to make informed choices about its use. Transparent communication and mechanisms for opt-in or opt-out are critical for respecting patient autonomy.
- Equitable Access to Benefits:
- Challenge: Ensuring that the benefits of drug discovery research are distributed equitably is a challenge, especially when data may be sourced from diverse populations. There is a risk of disparities in access to innovative treatments.
- Ethical Consideration: Researchers should strive for inclusivity in study populations and address disparities in healthcare access. Ethical considerations should extend beyond data collection to the equitable distribution of benefits derived from research outcomes.
- Transparent Communication:
- Challenge: Transparently communicating research findings, methodologies, and potential implications to the public can be challenging, especially when dealing with complex big data analyses.
- Ethical Consideration: Researchers have an ethical obligation to communicate their findings in a transparent and accessible manner. This includes sharing limitations, uncertainties, and potential societal impacts to foster public trust and understanding.
- Accountability and Responsible Conduct:
- Challenge: Ensuring accountability for ethical conduct throughout the research process is challenging, particularly in large, collaborative projects.
- Ethical Consideration: Researchers should adhere to principles of responsible conduct, fostering a culture of integrity and accountability. Clear authorship attribution, adherence to ethical guidelines, and transparency in reporting are essential components.
In navigating these challenges and ethical considerations, researchers, institutions, and policymakers must work collaboratively to establish and adhere to ethical standards that prioritize the rights, privacy, and well-being of individuals contributing to drug discovery research. Transparency, accountability, and a commitment to ethical principles are paramount in maintaining public trust and advancing responsible research practices.
VII. Integration with Emerging Technologies
The intersection of big data in drug discovery is further enhanced by the integration of emerging technologies, fostering innovative approaches and addressing inherent challenges. This synergy not only accelerates the drug discovery process but also contributes to enhanced security, efficiency, and data-driven decision-making. Key aspects include:
A. Synergies with Artificial Intelligence in Drug Discovery:
- Predictive Analytics for Target Identification:
- Integration: Artificial intelligence (AI) algorithms, including machine learning and deep learning, are integrated with big data to predict and prioritize potential drug targets based on complex biological datasets. AI enhances the efficiency of target identification by identifying patterns and relationships within vast datasets.
- Benefits: Accelerated target identification, increased precision in target selection, and the ability to explore novel therapeutic avenues.
- Drug Repurposing and AI-Driven Insights:
- Integration: AI is utilized to analyze large-scale datasets, including omics data, clinical records, and literature, to identify existing drugs with potential for repurposing. Machine learning models predict new therapeutic uses based on shared molecular pathways or known drug interactions.
- Benefits: Rapid identification of repurposable drugs, cost-effective exploration of existing compounds, and potential acceleration of drug development timelines.
- AI-Powered Drug Design and Optimization:
- Integration: In silico drug design is enhanced by AI algorithms that generate and optimize molecular structures based on desired pharmacological properties. Generative models and reinforcement learning contribute to the design of novel compounds with optimized properties.
- Benefits: Accelerated drug design processes, exploration of diverse chemical spaces, and the identification of novel drug candidates with improved efficacy and safety profiles.
- Predictive Toxicology and Safety Assessment:
- Integration: AI models are applied to predict potential toxicity and safety issues associated with drug candidates. These models analyze chemical properties, biological interactions, and historical safety data to identify potential risks early in the development process.
- Benefits: Enhanced safety assessments, early identification of potential adverse effects, and the reduction of safety-related attrition in later stages of drug development.
B. Blockchain for Secure Data Management:
- Secure Data Sharing and Collaboration:
- Integration: Blockchain technology is integrated to create decentralized and secure data-sharing platforms. Smart contracts enable transparent and secure collaboration among multiple stakeholders, ensuring data integrity and traceability.
- Benefits: Enhanced security and transparency in data sharing, reduced risks of data tampering, and streamlined collaboration in multi-institutional research projects.
- Patient-Centric Data Ownership:
- Integration: Blockchain empowers patients with greater control over their health data. Patients can securely share specific data elements with researchers while maintaining control over access permissions through blockchain-based consent mechanisms.
- Benefits: Improved patient privacy, increased trust in data-sharing processes, and a more patient-centric approach to data ownership and consent.
- Data Traceability and Auditing:
- Integration: Blockchain provides an immutable and transparent ledger for tracking the entire lifecycle of data, including data acquisition, processing, and sharing. This facilitates robust auditing and ensures the traceability of data-related activities.
- Benefits: Enhanced data traceability, auditability, and accountability, reducing the risks of data manipulation or unauthorized access.
C. Trends and Advancements at the Intersection of Data Science and Drug Discovery:
- Real-Time Data Analytics:
- Integration: Advances in real-time data analytics enable the continuous monitoring and analysis of data streams generated from various sources, including clinical trials, wearable devices, and electronic health records.
- Benefits: Timely insights into patient responses, adaptive trial designs, and the ability to make data-driven decisions in real-time.
- Quantum Computing for Drug Discovery:
- Integration: The potential integration of quantum computing with big data holds promise for solving complex computational problems in drug discovery, such as simulating molecular interactions and optimizing chemical structures.
- Benefits: Accelerated simulations, improved accuracy in molecular modeling, and the exploration of quantum algorithms for drug discovery applications.
- Integration with 5G Technology:
- Integration: The synergy between big data and 5G technology enhances connectivity, enabling the seamless transfer of large datasets, real-time collaboration, and the integration of IoT devices in drug discovery processes.
- Benefits: Faster data transfer, enhanced remote collaboration, and the utilization of IoT devices for real-time monitoring and data collection.
- Explainable AI for Interpretability:
- Integration: Explainable AI techniques are integrated with big data analytics to enhance the interpretability of AI-driven models in drug discovery. This is crucial for understanding the rationale behind model predictions.
- Benefits: Improved trust in AI models, better alignment with regulatory requirements, and increased understanding of the factors influencing model predictions.
- Personalized Medicine and Biomarker Discovery:
- Integration: Advances in data science and big data analytics contribute to the discovery of personalized medicine strategies and biomarkers. Patient-specific data, including genomics and clinical information, are analyzed to tailor treatments and identify predictive biomarkers.
- Benefits: Tailored treatment plans, improved patient outcomes, and the identification of patient subgroups for targeted therapies.
In summary, the integration of emerging technologies with big data in drug discovery opens new frontiers for innovation. From AI-driven insights to blockchain-enabled secure data management, these synergies enhance the efficiency, security, and ethical conduct of drug discovery processes, ultimately contributing to the development of safer and more effective therapeutics.