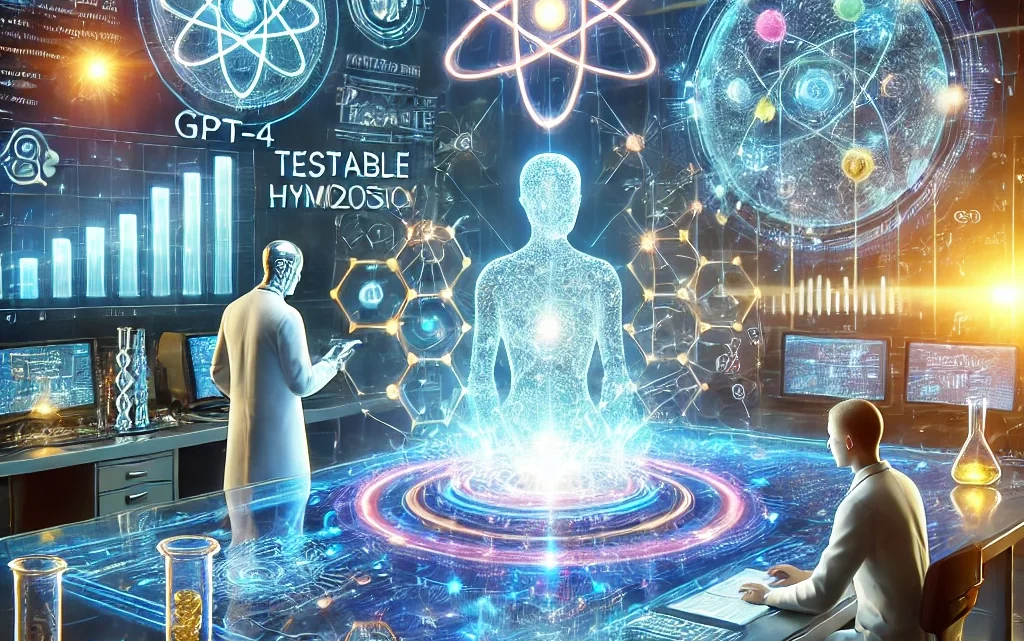
Emerging and Evolving Research Areas in Bioinformatics:
December 29, 2024In the fast-paced world of bioinformatics, staying informed about the most relevant and promising research areas is crucial, especially for newcomers and beginners in the field. While some topics may have reached their peak or have been superseded by newer advancements, there are still a number of exciting research areas that are evolving rapidly and holding strong potential. This post will highlight these areas, discuss their importance, applications, and how they’re shaping the future of bioinformatics.
1. Protein Structure Prediction: Beyond the Basics
While traditional protein secondary structure prediction methods have reached a plateau in performance, the field has shifted focus towards predicting more complex protein properties. Tools such as Disprot, IUPred, and RONN are gaining attention for their ability to predict the “unstructure” or flexible regions of proteins, offering a fresh perspective on protein behavior. Advances in protein docking and interaction predictions are also driving innovation, making this area a critical and evolving focus in bioinformatics.
Recent Trends & Tools:
- Deep learning-based methods have significantly enhanced the accuracy of protein structure prediction, moving beyond simple secondary structures to incorporate more intricate details such as protein folding and interaction networks.
- AlphaFold, a revolutionary tool developed by DeepMind, has brought about a breakthrough in the prediction of protein 3D structures.
2. Next-Generation Sequencing (NGS) Data Analysis
While short-read (<50 bp) alignment algorithms were once crucial for sequence alignment, the increasing length of sequence reads, particularly with platforms like PacBio and Oxford Nanopore, has rendered these methods less necessary. The focus now is on the development of algorithms that can handle long-read sequences, providing more accurate and comprehensive insights into genomic data.
Recent Trends & Tools:
- Long-read sequencing technologies are now capable of generating more complete and accurate genomic data, reducing the need for short-read alignment.
- Newer alignment algorithms such as minimap2 and graph-based approaches are better equipped to handle long reads and complex genomic regions.
3. RNA-Seq and Microarray Data Integration
While microarrays were once the go-to method for gene expression profiling, the advent of RNA-Seq technologies has shifted the landscape. However, both methods still have their place in research, with RNA-Seq providing deeper insights and microarrays still used in certain applications. The integration of RNA-Seq and microarray data is a growing area of interest, as researchers aim to leverage the strengths of both platforms for more accurate and comprehensive analyses.
Recent Trends & Tools:
- The development of hybrid approaches that combine RNA-Seq with microarray data for better quantification of gene expression.
- Bioconductor packages like DESeq2 and edgeR, which streamline RNA-Seq analysis, are improving data integration and reducing the need for separate normalization methods.
4. Genome-Wide Association Studies (GWAS)
The correction of multiple testing issues in GWAS has seen significant advancements, but the focus has shifted from simply improving statistical methods to refining the understanding of genetic variants and their functional implications. Researchers are now exploring more efficient ways to link genetic variations to specific diseases and traits.
Recent Trends & Tools:
- Machine learning algorithms are being used to identify novel genetic associations in GWAS, moving beyond simple p-value correction to incorporate more sophisticated data models.
- Polygenic risk scores are becoming more prevalent as tools to predict disease susceptibility based on genetic variants.
5. Gene Prediction and Annotation
In-silico gene prediction, especially for prokaryotes, was once a heavily researched topic, but improvements in RNA profiling and the expansion of genomic databases have made traditional methods less critical. Current research is now more focused on improving gene annotation accuracy using a combination of experimental and computational approaches.
Recent Trends & Tools:
- The rise of RNA-Seq technologies has revolutionized gene prediction, offering more accurate insights into transcript boundaries and gene expression.
- The integration of machine learning techniques with RNA-Seq data is making gene annotation more precise and faster than ever before.
6. Gene and Protein Music: A Creative Twist on Data Analysis
While protein music and genome sonification may seem like a quirky research topic, there is a niche community exploring its potential for visualizing large-scale genomic data. Tools like ComposAlign allow researchers to sonify genomic data, offering an innovative way to “listen” to genomic sequences and alignments, providing an alternative perspective for bioinformaticians.
Recent Trends & Tools:
- The development of sonification tools that map genes to musical motifs, allowing researchers to explore genomic data in a more interactive and intuitive way.
7. Single-Cell Genomics: Unraveling the Complexity of Individual Cells
Single-cell genomics has transformed the way we study cellular diversity, allowing for the analysis of gene expression, mutations, and epigenetic variations at the single-cell level. This technique has provided deep insights into cellular heterogeneity, especially in complex tissues like the brain and tumors. As the technology evolves, the challenge is to enhance its resolution and integrate single-cell data with other omics layers to build a more comprehensive understanding of biological systems.
Recent Trends & Tools:
- Single-cell RNA sequencing (scRNA-seq) continues to evolve, offering more refined methods for profiling gene expression at a single-cell resolution.
- Multi-omics single-cell analysis is becoming increasingly important as researchers look to combine transcriptomic, epigenomic, and proteomic data at the single-cell level.
8. CRISPR-Cas9 and Gene Editing Technologies
Gene editing technologies, particularly CRISPR-Cas9, have revolutionized molecular biology and bioinformatics. This technology allows for precise modifications to the genome, and bioinformaticians are working on improving the tools that guide these modifications. These advancements include predicting off-target effects, designing more efficient CRISPR tools, and integrating CRISPR outcomes into genomic data analysis workflows.
Recent Trends & Tools:
- AI-driven CRISPR design tools like CRISPR-DO and DeepCRISPR are improving the efficiency and accuracy of gene editing.
- Predictive algorithms are helping to minimize off-target effects, making gene editing safer and more reliable.
9. Artificial Intelligence in Drug Discovery and Precision Medicine
Artificial intelligence (AI) is transforming drug discovery and precision medicine by leveraging large-scale biological data to identify potential therapeutic targets. AI-driven models are becoming increasingly adept at predicting drug responses based on genetic and clinical data. As the AI field evolves, bioinformatics researchers are using machine learning and deep learning techniques to design more effective drugs, personalize treatments, and streamline clinical trials.
Recent Trends & Tools:
- Machine learning algorithms are being used to predict drug-target interactions, identify biomarkers, and forecast patient responses to specific treatments.
- Deep learning models, such as neural networks, are being applied to predict drug efficacy and safety, which can significantly reduce the time and cost involved in drug development.
10. Personalized and Predictive Genomics
The field of genomics is increasingly focused on tailoring healthcare to individual patients through personalized medicine. Predictive genomics, which uses genetic information to forecast disease risk and treatment responses, is becoming more integrated into clinical practice. Bioinformatics tools are crucial in analyzing genomic data to provide actionable insights that can guide therapeutic decisions.
Recent Trends & Tools:
- The development of predictive models for diseases like cancer, cardiovascular disease, and neurodegenerative disorders based on genetic, environmental, and lifestyle factors.
- Genome-wide prediction tools that combine genomics with environmental and phenotypic data to forecast disease risks and recommend personalized treatments.
11. Cloud Computing and Big Data in Bioinformatics
The vast amounts of data generated in bioinformatics research require powerful computing resources for storage, analysis, and collaboration. Cloud computing has emerged as an essential tool for bioinformatics, providing scalable and accessible computing power to analyze big data. Researchers are now exploring new methods for data storage, retrieval, and sharing, as well as ensuring data privacy and security in the cloud environment.
Recent Trends & Tools:
- Cloud-based platforms like Amazon Web Services (AWS) and Google Cloud are providing bioinformatics researchers with flexible, on-demand computational resources.
- Data-sharing platforms are being developed to allow researchers to access and analyze large datasets in real-time, while adhering to ethical guidelines regarding data privacy and security.
12. Blockchain Technology for Bioinformatics Data Security
Blockchain technology, known for its application in cryptocurrency, is increasingly being explored for its potential in bioinformatics to ensure data security, privacy, and integrity. As bioinformatics datasets grow in size and complexity, ensuring that data remains tamper-proof and accessible only to authorized individuals becomes critical. Blockchain can provide a decentralized, transparent method of recording and sharing data that can help overcome many of the current limitations in bioinformatics data sharing and protection.
Recent Trends & Tools:
- Decentralized data management systems are being developed to store genomic and clinical data in a way that prevents unauthorized access while still enabling transparency and collaboration.
- Blockchain-based platforms like MedChain are being used to facilitate secure sharing and collaboration in health research, ensuring data privacy and security while enabling real-time data sharing among institutions and researchers.
13. Synthetic Biology and Bioinformatics
Synthetic biology is a rapidly developing field that combines biology, engineering, and computer science to design and construct new biological parts, devices, and systems. Bioinformatics plays a crucial role in synthetic biology by providing the computational tools needed to design genetic circuits, model biological systems, and predict the behavior of synthetic organisms. As synthetic biology continues to advance, bioinformatics will be instrumental in developing more complex and efficient synthetic organisms for use in medicine, agriculture, and environmental applications.
Recent Trends & Tools:
- Gene synthesis and pathway design tools are being enhanced with bioinformatics algorithms that predict the behavior of synthetic organisms in different environments.
- Synthetic biology databases such as SynBioHub are providing a platform for storing, sharing, and analyzing synthetic biology data, enabling more streamlined and efficient designs.
14. Epigenomics and Its Role in Disease Understanding
Epigenomics is the study of changes in gene activity that do not involve alterations in the DNA sequence itself but still play a critical role in regulating gene expression. These changes, such as DNA methylation and histone modifications, are central to many diseases, including cancer, neurological disorders, and autoimmune diseases. Bioinformatics tools are crucial for analyzing epigenomic data, as they enable the mapping and interpretation of complex modifications across the genome, providing valuable insights into disease mechanisms and potential therapeutic targets.
Recent Trends & Tools:
- DNA methylation sequencing methods like Bisulfite-Seq and ATAC-Seq are becoming more accessible and are widely used for epigenetic profiling.
- Epigenetic databases like ENCODE and Roadmap Epigenomics are growing in scope, offering a rich source of reference data to study how epigenetic modifications influence gene expression and contribute to disease.
15. Metagenomics and Microbiome Analysis
Metagenomics, the study of genetic material recovered directly from environmental samples, is a field that is expanding rapidly due to the growth of high-throughput sequencing technologies. The human microbiome, in particular, is a complex ecosystem that significantly impacts human health. By analyzing metagenomic data, bioinformaticians can identify the microorganisms present in a sample and understand how their interactions affect disease states such as obesity, diabetes, and autoimmune disorders.
Recent Trends & Tools:
- Metagenomic sequencing platforms like Illumina and PacBio are widely used to sequence microbial communities, enabling detailed microbiome profiling.
- Microbiome analysis tools such as QIIME and MG-RAST help researchers process, analyze, and visualize microbiome data, offering insights into microbial diversity, function, and their links to health.
16. Automating Bioinformatics Workflows
The complexity and volume of bioinformatics data are leading to the development of automated tools and platforms that can streamline research workflows. These systems help minimize human error, reduce time spent on data processing, and increase reproducibility. As bioinformatics workflows become more automated, researchers can focus more on interpreting data and drawing meaningful conclusions rather than spending time on manual data analysis tasks.
Recent Trends & Tools:
- Automated bioinformatics pipelines are being developed to integrate various steps of analysis, from raw sequence data processing to advanced statistical analysis.
- Cloud-based workflow automation tools such as Nextflow and Cromwell are gaining popularity, allowing researchers to design, manage, and execute bioinformatics workflows in scalable and reproducible ways.
17. Artificial Intelligence and Machine Learning in Bioinformatics
Artificial Intelligence (AI) and Machine Learning (ML) are transforming bioinformatics by providing powerful tools for analyzing vast amounts of biological data. These technologies enable the discovery of hidden patterns, make predictions, and automate complex tasks that would otherwise be time-consuming. AI and ML are being used for tasks such as genome annotation, drug discovery, biomarker identification, and personalized medicine.
Recent Trends & Tools:
- Deep learning algorithms are being used to predict protein folding, understand genetic mutations, and create more accurate models of disease mechanisms.
- AI-driven drug discovery platforms like Atomwise and Insilico Medicine are accelerating the process of identifying potential drug candidates by analyzing biological data more efficiently.
- ML-based predictive models are aiding in the identification of disease biomarkers by recognizing patterns in patient data, enabling more precise diagnostic tools.
18. Single-Cell Genomics and Transcriptomics
Single-cell genomics and transcriptomics are revolutionizing our understanding of cellular diversity and function. By analyzing individual cells, researchers can gain insights into gene expression, mutations, and cell-to-cell variability that are often missed when studying bulk tissue samples. These techniques are particularly valuable for studying complex tissues, such as the brain or tumor microenvironments, where heterogeneity plays a critical role in disease progression.
Recent Trends & Tools:
- Single-cell RNA sequencing (scRNA-seq) is being widely used to profile gene expression at the single-cell level, providing detailed insights into cellular heterogeneity and gene regulatory networks.
- Single-cell genomic platforms like 10x Genomics and Fluidigm are helping researchers capture high-resolution data from individual cells, making it possible to study rare cell populations or investigate dynamic processes like immune responses and cancer evolution.
19. Next-Generation Sequencing (NGS) and Bioinformatics Integration
Next-Generation Sequencing (NGS) technologies have dramatically reduced the cost and time required for DNA sequencing, enabling large-scale genomic studies. These technologies are increasingly integrated with bioinformatics pipelines to process, analyze, and interpret the massive amounts of data generated. NGS is now widely used in various applications, including whole-genome sequencing, exome sequencing, RNA sequencing, and targeted gene sequencing.
Recent Trends & Tools:
- NGS-based diagnostic tools are improving the accuracy and speed of genetic testing, facilitating the identification of genetic disorders, cancer mutations, and infectious diseases.
- Bioinformatics pipelines like GATK (Genome Analysis Toolkit) and STAR (Spliced Transcripts Alignment to a Reference) are designed to handle the large volumes of data produced by NGS, ensuring efficient analysis and accurate results.
20. Precision Medicine and Bioinformatics
Precision medicine, which tailors treatment based on individual genetic profiles, is becoming a cornerstone of modern healthcare. Bioinformatics plays a crucial role in analyzing genomic and clinical data to guide personalized treatment strategies. By leveraging data from a patient’s genome, lifestyle, and environment, bioinformatics tools help clinicians design more effective, targeted therapies that maximize patient outcomes.
Recent Trends & Tools:
- Personalized cancer treatment is increasingly relying on bioinformatics tools to identify specific mutations in tumors, enabling clinicians to select the most appropriate therapies.
- Pharmacogenomics is being used to predict how individuals will respond to specific drugs based on their genetic makeup, optimizing drug efficacy and reducing adverse effects.
21. Data Standardization and Interoperability in Bioinformatics
As the amount of biological data grows, ensuring that data is standardized and interoperable across different platforms and systems becomes essential. This is particularly important for collaborative research efforts and for ensuring that data can be shared and analyzed across different institutions and countries. Bioinformatics standards are evolving to facilitate data sharing, improve reproducibility, and ensure that data can be integrated across different domains.
Recent Trends & Tools:
- FAIR principles (Findable, Accessible, Interoperable, Reusable) are becoming the foundation for data standards in bioinformatics, ensuring that data can be easily accessed and integrated by different researchers and systems.
- Bioinformatics data repositories such as GenBank, EMBL, and the Gene Expression Omnibus (GEO) are continually improving their data standards to ensure that large datasets can be shared and interpreted with consistency.
22. Bioinformatics in Agricultural and Environmental Science
Bioinformatics is making significant contributions to agriculture and environmental science, where genomic data can help optimize crop yields, improve pest resistance, and mitigate the impact of climate change. With the rise of genomic technologies and bioinformatics tools, researchers can better understand plant and animal genetics, monitor biodiversity, and develop more sustainable agricultural practices.
Recent Trends & Tools:
- Crop genomics is helping scientists develop genetically modified crops that are more resistant to drought, pests, and diseases, improving food security in the face of climate change.
- Environmental DNA (eDNA) sequencing, combined with bioinformatics, is enabling more accurate monitoring of biodiversity and ecosystem health, helping protect endangered species and track invasive species.
Conclusion: Embracing Bioinformatics for a Healthier Future
The future of bioinformatics is bright, with new technologies and methodologies continually emerging that push the boundaries of what is possible in biological research and healthcare. From genomics and personalized medicine to artificial intelligence and synthetic biology, the integration of bioinformatics into various scientific disciplines is driving unprecedented advances in our understanding of biology.
As we move forward, bioinformatics will continue to play a critical role in improving human health, addressing global challenges such as infectious diseases, chronic conditions, and environmental sustainability. By embracing these innovations, researchers, clinicians, and bioinformaticians can work together to create a healthier and more sustainable future for all.