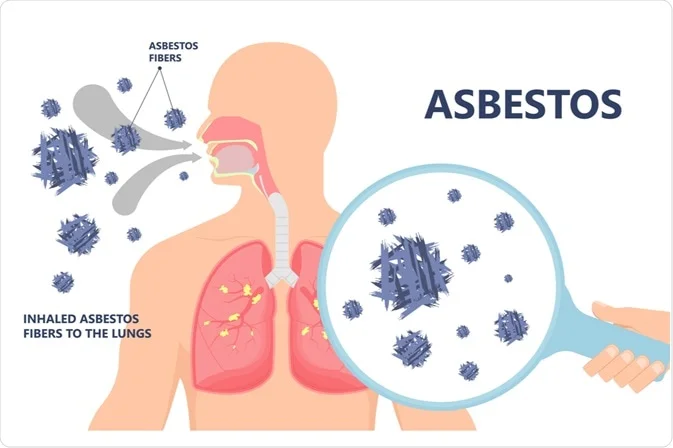
Navigating the Omics Landscape of Mesothelioma: A Comprehensive Guide
November 28, 2023Table of Contents
I. Introduction
In the dynamic landscape of cancer research, emerging omics approaches have become instrumental in unraveling the complexities of diseases like mesothelioma. This introductory segment defines the evolving omics methodologies employed in the study of cancer and underscores the promise that multi-omics holds for achieving a profound understanding of mesothelioma.
Defining Emerging Omics Approaches to Study Cancer:
- Genomics:
- Genetic Blueprint: Genomics delves into the complete set of genes within a cell, uncovering the genetic blueprint that governs normal cellular functions and the aberrations leading to cancer.
- Mutational Landscape: Understanding genomic alterations in mesothelioma sheds light on key mutations driving its development and progression.
- Transcriptomics:
- Gene Expression Patterns: Transcriptomics focuses on the study of RNA transcripts, elucidating the gene expression patterns that define mesothelioma cells.
- Insights into Dysregulation: Examining the transcriptome provides insights into how genetic information is translated into functional molecules, revealing dysregulations specific to mesothelioma.
- Epigenomics:
- Epigenetic Modifications: Epigenomics investigates changes in gene activity that do not involve alterations to the underlying DNA sequence. This includes DNA methylation and histone modifications.
- Impact on Mesothelioma Progression: Understanding epigenetic changes provides crucial insights into how alterations in gene regulation contribute to mesothelioma progression.
- Proteomics:
- Protein Landscape: Proteomics explores the entire complement of proteins within a cell, elucidating the dynamic protein landscape that orchestrates cellular functions.
- Biomarker Discovery: Identification of mesothelioma-specific protein signatures aids in biomarker discovery for early detection and targeted therapies.
- Metabolomics:
- Metabolic Signatures: Metabolomics examines the small molecules involved in cellular metabolism, revealing unique metabolic signatures associated with mesothelioma.
- Metabolic Reprogramming: Understanding metabolic reprogramming in mesothelioma cells provides insights into energy production and nutrient utilization in the tumor microenvironment.
Promise of Multi-Omics for a Deeper Understanding of Mesothelioma:
- Holistic Insights:
- Comprehensive Analysis: Multi-omics approaches integrate data from genomics, transcriptomics, epigenomics, proteomics, and metabolomics, offering a comprehensive view of mesothelioma.
- Holistic Understanding: The synergistic analysis of multiple omics layers enhances our ability to comprehend the intricate molecular landscape and heterogeneity of mesothelioma.
- Identifying Molecular Networks:
- Network Biology: Multi-omics facilitates the identification of interconnected molecular networks within mesothelioma cells.
- Uncovering Crosstalk: Examining how different omics layers interact reveals crosstalk between genetic, epigenetic, and metabolic processes, providing a more nuanced understanding.
- Personalized Approaches:
- Precision Medicine: Multi-omics contributes to the foundation of precision medicine by enabling the identification of individualized molecular profiles in mesothelioma patients.
- Tailoring Therapies: Personalized treatment strategies can be developed by considering the unique molecular characteristics identified through multi-omics analyses.
- Biomarker Discovery and Early Detection:
- Early Warning Signs: Multi-omics enhances the discovery of robust biomarkers for early detection, allowing interventions at more treatable stages of mesothelioma.
- Monitoring Treatment Response: The combination of omics approaches aids in monitoring treatment responses, guiding adjustments for optimal therapeutic outcomes.
In conclusion, as we navigate the intricate realm of mesothelioma, the integration of emerging omics approaches offers an unprecedented opportunity to unravel the molecular intricacies of this challenging cancer. The promise of multi-omics lies in its potential to provide a deeper, more holistic understanding of mesothelioma, paving the way for transformative advancements in diagnosis, treatment, and ultimately improving outcomes for individuals affected by this disease.
II. Genomics and Sequencing
Exploring the genomic landscape of mesothelioma involves deciphering DNA mutations, chromosomal changes, and employing bioinformatics mapping techniques. The following section delves into the intricacies of mesothelioma genomes, shedding light on genetic alterations and leveraging bioinformatics for comprehensive insights. Additionally, the reference to the TCGA genomic landscape provides a foundational understanding of mesothelioma tumors at the molecular level.
1. DNA Mutations and Chromosomal Changes in Meso Genomes:
- Genetic Alterations: Mesothelioma genomes harbor a spectrum of DNA mutations, including single nucleotide variations (SNVs), insertions, deletions, and structural variations.
- Chromosomal Instability: Chromosomal changes, such as copy number variations (CNVs) and chromosomal rearrangements, contribute to the genomic instability observed in mesothelioma.
- Driver Mutations: Identification of driver mutations—those causally implicated in oncogenesis—provides critical insights into the molecular mechanisms driving mesothelioma.
2. Bioinformatics Mapping of Genetic Alterations:
- High-Throughput Sequencing: State-of-the-art high-throughput sequencing technologies are employed for comprehensive mapping of genetic alterations in mesothelioma genomes.
- Variant Calling Algorithms: Bioinformatics tools, including variant calling algorithms, are crucial for distinguishing between germline and somatic mutations, filtering noise, and identifying genuine genetic changes.
- Annotation and Interpretation: Annotation tools provide functional insights into the impact of genetic alterations on genes and pathways, aiding in the interpretation of the genomic landscape.
3. TCGA Genomic Landscape of Mesothelioma Tumors:
- The Cancer Genome Atlas (TCGA): TCGA is a seminal initiative that characterizes the genomic landscape of various cancers, including mesothelioma.
- Comprehensive Genomic Data: TCGA provides comprehensive datasets encompassing genomics, transcriptomics, and clinical information, facilitating an integrative understanding of mesothelioma.
- Key Findings: Analysis of TCGA data has unveiled specific genetic alterations, signaling pathways, and molecular subtypes within mesothelioma, contributing to our knowledge of the disease.
Clinical Implications and Future Directions:
- Targeted Therapies: Genomic insights guide the development of targeted therapies tailored to the specific genetic alterations observed in mesothelioma.
- Patient Stratification: Identification of molecular subtypes based on genomic characteristics allows for more refined patient stratification, enabling personalized treatment approaches.
- Biomarker Discovery: Genomic data serves as a rich resource for biomarker discovery, facilitating the identification of indicators for early detection, prognosis, and treatment response.
Challenges and Ongoing Research:
- Tumor Heterogeneity: Mesothelioma exhibits intratumor and intertumor heterogeneity, posing challenges in capturing the full spectrum of genetic alterations.
- Integration with Other Omics Layers: Ongoing research focuses on integrating genomic data with other omics layers, such as transcriptomics and proteomics, to gain a more comprehensive understanding of mesothelioma biology.
Conclusion:
Genomics and sequencing methodologies play a pivotal role in unraveling the genetic intricacies of mesothelioma. From identifying DNA mutations and chromosomal changes to utilizing advanced bioinformatics tools and leveraging large-scale initiatives like TCGA, the genomic landscape of mesothelioma is becoming increasingly elucidated. The ongoing integration of genomics into clinical practice holds the promise of advancing precision medicine, ultimately improving outcomes for individuals grappling with this challenging cancer.
III. Transcriptomics and Expression Profiles
Transcriptomics, involving the study of RNA expression, unveils the dynamic gene expression profiles within mesothelioma cells. This section delves into the intricacies of RNA sequencing, the identification of gene signatures and biomarker panels, and the transformative role of transcriptomics in advancing precision medicine for mesothelioma.
1. RNA Sequencing of Mesothelioma Cells and Pathways:
- Comprehensive Gene Expression Analysis: RNA sequencing allows for a comprehensive analysis of the transcriptome, providing insights into the gene expression patterns of mesothelioma cells.
- Differential Expression Analysis: Comparative analysis of RNA expression between normal and mesothelioma tissues reveals dysregulated pathways implicated in disease progression.
- Pathway Enrichment Analysis: Bioinformatics tools are employed for pathway enrichment analysis, elucidating the functional implications of altered gene expression in mesothelioma.
2. Identifying Gene Signatures and Biomarker Panels:
- Gene Signatures: Transcriptomics aids in the identification of specific gene signatures associated with mesothelioma subtypes, stages, or treatment responses.
- Biomarker Panels: Transcriptomic analyses contribute to the discovery of biomarker panels that can serve as diagnostic, prognostic, or predictive indicators.
- Non-Invasive Biomarkers: RNA expression profiles in biofluids, such as blood or pleural effusions, offer non-invasive opportunities for biomarker discovery and disease monitoring.
3. Transcriptomics for Precision Medicine:
- Patient Stratification: Transcriptomic data facilitates the stratification of mesothelioma patients into distinct molecular subtypes, guiding more personalized treatment strategies.
- Targeted Therapies: Understanding the gene expression profiles enables the identification of specific molecular targets for targeted therapies tailored to individual patients.
- Treatment Response Monitoring: Monitoring changes in gene expression during treatment allows for real-time assessment of treatment responses and adaptation of therapeutic strategies.
Clinical Implications and Future Directions:
- Precision Oncology Approaches: Transcriptomics contributes to the foundation of precision oncology, where treatment decisions are tailored based on the unique RNA expression profiles of mesothelioma tumors.
- Therapeutic Opportunities: The identification of druggable targets through transcriptomic analyses opens avenues for novel therapeutic interventions.
- Integration with Genomic Data: Integrating transcriptomic data with genomic information enhances the understanding of the molecular landscape, providing a more comprehensive basis for precision medicine.
Challenges and Ongoing Research:
- Intra-Tumor Heterogeneity: Intra-tumor heterogeneity poses challenges in capturing the full spectrum of gene expression patterns within different regions of a mesothelioma tumor.
- Longitudinal Studies: Ongoing research focuses on longitudinal transcriptomic studies to unravel the dynamic changes in gene expression over the course of mesothelioma progression and treatment.
Conclusion:
Transcriptomics stands as a cornerstone in deciphering the molecular intricacies of mesothelioma. From unraveling RNA expression profiles to identifying gene signatures and biomarker panels, transcriptomic analyses offer valuable insights with profound implications for precision medicine. As research advances, the integration of transcriptomics into clinical practice holds the potential to revolutionize treatment approaches, providing more personalized and effective strategies for individuals facing mesothelioma.
IV. Proteomics and Functional Analysis
Proteomics, involving the comprehensive study of proteins, provides insights into the functional aspects of mesothelioma. This section explores the application of mass spectrometry for protein profiling, the efforts of the NCI Clinical Proteomic Tumor Analysis Consortium, and the significance of verifying genomic findings at the protein level.
1. Mass Spectrometry Profiling of Proteins:
- Proteome Characterization: Mass spectrometry enables the systematic identification and quantification of proteins within mesothelioma cells, offering a comprehensive view of the proteome.
- Post-Translational Modifications: Proteomic analysis extends to the identification of post-translational modifications, providing insights into protein function and regulation.
- Quantitative Proteomics: Advances in quantitative proteomics allow for the comparison of protein expression levels between normal and mesothelioma tissues.
2. NCI Clinical Proteomic Tumor Analysis Consortium Efforts:
- Consortium Objectives: The National Cancer Institute (NCI) Clinical Proteomic Tumor Analysis Consortium (CPTAC) focuses on advancing proteomic technologies to understand the molecular basis of cancer.
- Mesothelioma Proteomic Studies: CPTAC’s efforts include proteomic studies specific to mesothelioma, contributing valuable data to the broader understanding of the disease.
- Data Integration: Integrating proteomic data with genomics and other omics layers enhances the multidimensional characterization of mesothelioma.
3. Verifying Genomic Findings at the Protein Level:
- From Genomics to Proteomics: Proteomic analysis serves as a crucial step in verifying genomic findings, confirming the translation of genetic alterations into functional changes at the protein level.
- Correlation with Transcriptomics: Integrating proteomic and transcriptomic data allows for a more comprehensive correlation between gene expression and protein abundance.
- Validation of Biomarkers: Protein-level validation is essential for validating potential biomarkers discovered through genomic and transcriptomic analyses.
Clinical Implications and Future Directions:
- Functional Insights: Proteomics provides functional insights into the role of specific proteins and pathways in mesothelioma, aiding in the identification of therapeutic targets.
- Biomarker Discovery: Proteomic data contributes to biomarker discovery, offering potential markers for diagnosis, prognosis, and treatment response.
- Personalized Medicine: Understanding the proteomic landscape enhances the potential for developing personalized therapeutic approaches tailored to the unique protein profiles of mesothelioma patients.
Challenges and Ongoing Research:
- Quantitative Challenges: Precise quantification of proteins, especially low-abundance ones, remains a challenge in proteomic analyses.
- Technological Advancements: Ongoing research focuses on technological advancements in mass spectrometry and proteomic methodologies to improve sensitivity and coverage.
Conclusion:
Proteomics emerges as a powerful tool for functional analysis, offering a detailed examination of the protein landscape within mesothelioma. Mass spectrometry, coupled with initiatives like the NCI CPTAC, contributes to unraveling the intricacies of protein expression and post-translational modifications. Verifying genomic findings at the protein level not only validates molecular insights but also holds immense potential for guiding precision medicine strategies in the diagnosis and treatment of mesothelioma. As proteomic technologies continue to advance, the integration of proteomics with other omics data shapes a more comprehensive understanding of this complex disease.
V. Immuno-Omics and Microenvironment
Immuno-omics, encompassing the study of the immune system’s role in cancer, is pivotal for understanding the intricate interplay between tumor immunocytes and cancer cells. This section explores the application of single-cell analysis to study tumor immunocytes and the mapping of interactions within the immune landscape and cancer cells in the microenvironment.
1. Single-Cell Analysis of Tumor Immunocytes:
- Resolution at Single-Cell Level: Single-cell analysis techniques, such as single-cell RNA sequencing, enable the study of individual immune cells within the tumor microenvironment.
- Heterogeneity Assessment: This approach provides insights into the heterogeneity of immune cell populations, unveiling distinct functional states and responses.
- Characterization of Tumor-Infiltrating Immune Cells: Single-cell analysis allows for the characterization of various immune cell types present in the tumor, including T cells, B cells, macrophages, and dendritic cells.
2. Mapping Interaction of Immune Landscape and Cancer Cells:
- Spatial Transcriptomics: Techniques like spatial transcriptomics provide a spatially resolved view of gene expression within the tumor, enabling the mapping of immune cell distribution.
- Functional Crosstalk: Studying the interactions between immune cells and cancer cells at the molecular level reveals functional crosstalk and communication.
- Tumor Immune Microenvironment (TIME): Understanding the dynamic landscape of immune cells within the tumor microenvironment, often referred to as the Tumor Immune Microenvironment (TIME), is crucial for deciphering the immune response to cancer.
Clinical Implications and Future Directions:
- Immunotherapeutic Strategies: Immuno-omics data guide the development of immunotherapeutic strategies by identifying targetable immune checkpoints and signaling pathways.
- Patient Stratification: The immune landscape revealed by immuno-omics contributes to patient stratification, predicting responses to immunotherapies and guiding personalized treatment approaches.
- Biomarker Discovery: Immuno-omics aids in the discovery of immune-related biomarkers, serving as indicators for treatment response and prognosis.
Challenges and Ongoing Research:
- Integration with Other Omics Layers: Ongoing research focuses on integrating immuno-omics data with genomics, transcriptomics, and proteomics to achieve a comprehensive understanding of the tumor microenvironment.
- Temporal Dynamics: Studying the temporal dynamics of immune responses within the tumor microenvironment is an evolving area of research.
Conclusion:
Immuno-omics provides a unique lens through which to explore the immune landscape within the tumor microenvironment. Single-cell analysis techniques offer unprecedented resolution, uncovering the heterogeneity and functional states of tumor-infiltrating immune cells. The mapping of interactions between the immune system and cancer cells informs the development of immunotherapeutic strategies and enhances our understanding of the dynamic interplay shaping the response to cancer. As immuno-omics continues to evolve, its integration with other omics layers holds the promise of transforming our approach to cancer treatment and fostering precision immunotherapy tailored to the individual characteristics of each patient’s immune response.
VI. Pharmaco-Omics and Drug Testing
Pharmaco-omics, an interdisciplinary field, explores the relationship between an individual’s omics profile and their response to drugs. This section examines the use of genomic screening to identify therapeutic vulnerabilities and delves into the role of pharmacogenomics in understanding chemotherapy response.
1. Genomic Screening for Therapeutic Vulnerabilities:
- Precision Medicine Approaches: Genomic screening involves the systematic analysis of an individual’s genomic profile to identify genetic alterations that may serve as therapeutic targets.
- Targeted Therapies: Identification of specific mutations or alterations through genomic screening allows for the selection of targeted therapies tailored to the unique genetic makeup of a patient’s tumor.
- Functional Genomics: Integration with functional genomics approaches enables the assessment of the functional consequences of identified genomic alterations.
2. Pharmacogenomics and Chemotherapy Response:
- Individualized Drug Response: Pharmacogenomics focuses on understanding how genetic variations influence an individual’s response to drugs, including chemotherapy agents.
- Genetic Variants and Drug Metabolism: Variants in genes involved in drug metabolism, transport, and target pathways influence drug efficacy and toxicity.
- Predictive Biomarkers: Pharmacogenomics identifies predictive biomarkers that inform clinicians about a patient’s likelihood of responding to specific chemotherapy drugs.
Clinical Implications and Future Directions:
- Tailored Treatment Plans: Pharmaco-omics guides the development of tailored treatment plans, optimizing therapeutic outcomes while minimizing adverse effects.
- Early Identification of Responders and Non-Responders: Understanding pharmacogenomic variations allows for the early identification of patients likely to respond positively or experience toxicity, enabling timely adjustments to treatment regimens.
- Integration with Clinical Decision Support Systems: The integration of pharmacogenomic data into clinical decision support systems enhances the implementation of precision medicine in oncology.
Challenges and Ongoing Research:
- Multifactorial Nature of Drug Response: Drug response is multifactorial, influenced by genetic, environmental, and lifestyle factors, necessitating ongoing research to unravel the complexities.
- Implementation in Clinical Practice: The challenge lies in effectively implementing pharmaco-omics findings into routine clinical practice, requiring collaborative efforts between researchers, clinicians, and regulatory bodies.
Conclusion:
Pharmaco-omics heralds a new era in personalized medicine, aligning treatment strategies with the individual molecular characteristics of each patient. Genomic screening identifies therapeutic vulnerabilities, paving the way for precision medicine approaches, while pharmacogenomics sheds light on the intricate interplay between genetic variations and chemotherapy response. As research in pharmaco-omics progresses, the translation of these findings into clinical practice holds the potential to revolutionize drug selection, dosage optimization, and treatment monitoring, ultimately improving outcomes for cancer patients.
VII. The Future of Multi-Omics Integration
The future of biomedical research is intricately tied to the seamless integration of diverse omics datasets. This section explores the merging of genomic, transcriptomic, and proteomic information, the application of computational approaches and machine learning to omics data, and the promising role of systems biology in guiding precision therapy.
1. Merging Genomic, Transcriptomic, Proteomic Datasets:
- Holistic Molecular Profiling: The integration of genomic, transcriptomic, and proteomic datasets aims to provide a holistic view of the molecular landscape within cells.
- Comprehensive Understanding: Merging these omics layers allows for a more comprehensive understanding of the interplay between genetic information (genomics), gene expression patterns (transcriptomics), and protein abundance and function (proteomics).
2. Computational Approaches and Machine Learning Applied to Omics:
- Data Integration Algorithms: Advanced computational algorithms are employed to integrate multi-omics datasets, overcoming challenges such as differences in data types and scales.
- Pattern Recognition: Machine learning techniques are applied to recognize patterns within integrated omics data, revealing complex relationships and identifying potential biomarkers and therapeutic targets.
- Predictive Modeling: Computational approaches enable the development of predictive models for disease outcomes, treatment responses, and the identification of patient subgroups.
3. Promise of Systems Biology Guiding Precision Therapy:
- Systems Biology Paradigm: Systems biology, incorporating multi-omics data, considers biological systems as interconnected networks rather than isolated components.
- Network Analysis: Analyzing the networks formed by genes, transcripts, and proteins provides a systems-level understanding, uncovering the regulatory mechanisms and signaling pathways driving diseases like cancer.
- Precision Therapy Strategies: Systems biology insights guide the development of precision therapy strategies, tailoring interventions based on the dynamic interactions within cellular systems.
Clinical Implications and Future Directions:
- Patient-Centric Approaches: Multi-omics integration supports patient-centric approaches, where treatment decisions are informed by a personalized understanding of the molecular landscape.
- Therapeutic Combinations: The identification of synergistic interactions between genomic, transcriptomic, and proteomic alterations opens avenues for developing combination therapies targeting multiple aspects of the disease.
- Real-Time Adaptations: Advanced computational tools enable real-time analysis of multi-omics data, facilitating dynamic adaptations of treatment plans based on evolving molecular profiles.
Challenges and Ongoing Research:
- Data Standardization: Standardizing multi-omics data across different platforms and technologies remains a challenge, requiring ongoing efforts for data harmonization.
- Interpretability of Complex Networks: Interpreting the intricate networks revealed by systems biology approaches is an ongoing area of research, with a focus on translating complex information into actionable insights.
Conclusion:
The future of multi-omics integration holds immense promise for advancing precision medicine. By merging genomic, transcriptomic, and proteomic datasets and applying computational and machine learning approaches, researchers gain a deeper understanding of disease mechanisms and treatment responses. The systems biology paradigm, with its emphasis on interconnected networks, is poised to guide precision therapy strategies, ushering in an era where the intricate complexities of individual patients’ molecular profiles inform targeted and effective interventions. As research in this field progresses, the integration of multi-omics approaches into clinical practice is expected to revolutionize the landscape of personalized medicine, offering more tailored and impactful treatments for complex diseases like cancer.