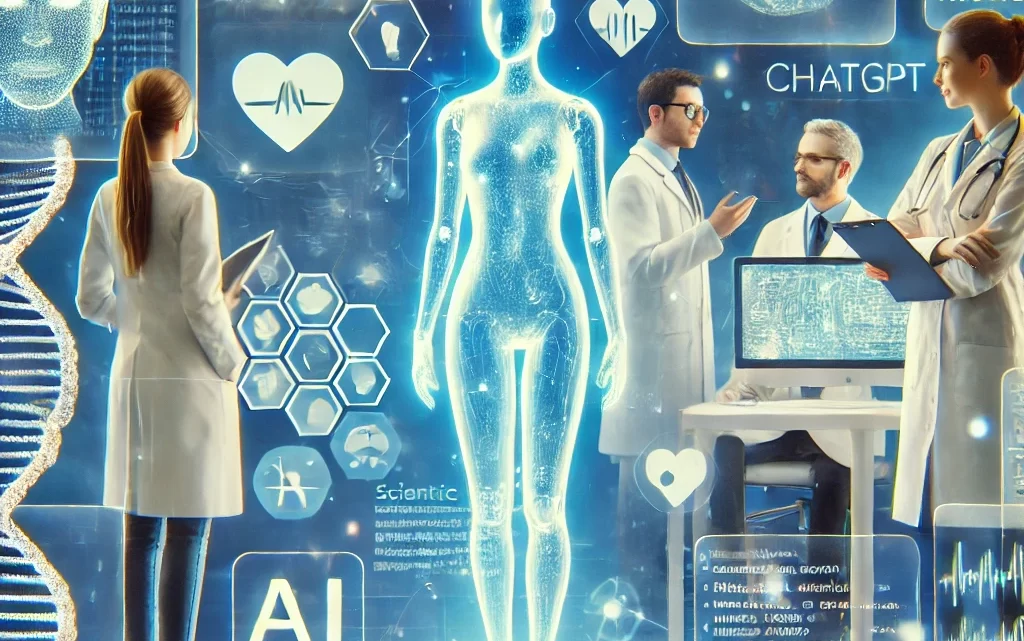
AI in Healthcare and Scientific Writing
December 17, 2024The rise of large language models (LLMs), such as ChatGPT, marks a transformative moment in how we generate and interact with text, especially within healthcare and academic writing. Driven by advanced neural networks, increasing computational power, and vast training datasets, these tools have disrupted traditional methods of communication, offering a myriad of possibilities while posing notable challenges. This blog explores the practical opportunities, inherent limitations, and implications of using LLMs like ChatGPT, with a focus on healthcare and biomedical research.
Opportunities of AI-Powered Text Generation
Artificial intelligence models like ChatGPT have revolutionized text generation, creating coherent, human-like outputs with remarkable accuracy. This capability makes them indispensable for academic writing and scientific communication, particularly in the biomedical domain. Non-native English speakers benefit significantly, as these tools democratize access to refined language, helping users articulate complex ideas effortlessly. A user can provide raw, unstructured content—bullet points or rough notes—and the AI transforms it into polished text, allowing for further refinement.
ChatGPT’s strengths also extend to specialized tasks beyond writing. For example, it can assist programmers with generating or converting source code, identifying bugs, or proofreading lines of code. In healthcare, it has passed standardized tests like the USMLE medical exam, showcasing its adaptability as a general-purpose AI without specific fine-tuning. While its current performance is impressive, future iterations may be fine-tuned for domain-specific tasks, potentially outperforming traditional machine learning models trained on structured datasets.
What sets LLMs apart is their chatbox functionality, which enables intuitive, free-text communication with users. Unlike traditional software tools requiring structured input, LLMs can engage interactively, improving accessibility to information for diverse groups, including patients and visually impaired individuals. These features hold promise for enhancing patient education, allowing healthcare professionals to bridge communication gaps effectively.
Challenges: Bias, Transparency, and Hallucinations
Despite their capabilities, AI-powered systems like ChatGPT come with inherent limitations that cannot be overlooked. The quality and accuracy of their output rely heavily on training data, which often contains bias reflective of content creators. This raises concerns about representation, as marginalized communities may be excluded, leading to outputs that amplify existing disparities.
A significant issue lies in the lack of transparency regarding the data sources and fine-tuning methods used to train these models. Users are often unaware of how ChatGPT arrives at its answers, and it does not provide confidence levels or uncertainties for its responses. When faced with unfamiliar topics, the model can fabricate information—a phenomenon known as “artificial hallucination”—delivering incorrect content with unwarranted confidence. In academic writing, this manifests when ChatGPT generates fake citations, including fabricated journal titles and PubMed IDs.
Concerns surrounding data privacy add another layer of complexity. OpenAI’s platform, for instance, processes user inputs and retains conversation history for further training. This poses a serious risk in healthcare settings, where sensitive medical information must be handled with the utmost caution. Users are advised against sharing protected health data, and any use of AI within healthcare facilities must comply with local IT regulations.
AI and Scientific Activities: Ethical and Practical Implications
The integration of LLMs into scientific workflows has raised ethical questions about authorship and responsibility. While tools like ChatGPT can streamline the writing process, they cannot replace critical aspects of scientific rigor, such as manual literature reviews and fact-checking. Editors and publishers are grappling with the role of LLMs in submissions, with some journals implementing bans while others consider updates to their policies. Transparency is key—any substantial use of LLMs in writing should be acknowledged, including the specific version used.
However, LLMs offer significant potential for addressing editorial challenges faced by journals and reviewers. Tasks like proofreading, formatting, and ensuring semantic consistency, which are time-consuming but require minimal expertise, can be outsourced to AI under proper supervision. While LLMs cannot replace peer reviewers’ judgment on scientific quality, they may ease the growing burden of manuscript submissions.
Practical Applications in Healthcare Communication
One of the most promising opportunities for LLMs lies in improving healthcare communication. If properly trained and validated, these tools could assist in patient education, translating complex medical information into simple, digestible language. This could improve access to care, particularly for underserved populations, and act as a support system for overburdened healthcare professionals.
For instance, ChatGPT could generate summaries of recent research findings, explain disease management guidelines, or provide step-by-step information on procedures and medication management. Similarly, physicians could leverage AI to simplify clinical workflows, such as dictating reports, extracting information from electronic medical records, or interpreting specialty reports (e.g., radiology) for general practitioners and patients. Such applications hold the potential to enhance resource utilization while preserving the essential human connection between doctors and patients.
Regulatory Challenges and Ethical Considerations
The use of generative AI in healthcare introduces several regulatory hurdles, particularly surrounding transparency and explainability. AI’s black-box nature makes it difficult for physicians to trust outputs without understanding the underlying reasoning. This lack of interpretability challenges core principles of medical ethics, such as patient autonomy and informed consent. In regions like the European Union, the AI Act and GDPR underscore the need for transparency, requiring AI applications in healthcare to disclose decision-making processes.
Furthermore, any software used for clinical decision-making qualifies as a medical device and must adhere to strict regulatory standards. These include quality and risk management systems to demonstrate medical benefits and ensure patient safety. ChatGPT, for instance, has not been approved as a medical device, and its use in routine clinical care could expose healthcare professionals to legal accountability.
Conclusion: A Balanced Path Forward
Large language models like ChatGPT offer unprecedented opportunities for advancing healthcare communication, scientific writing, and general-purpose tasks. They hold the potential to democratize access to information, improve patient education, and assist healthcare professionals in time-consuming workflows. However, challenges related to data bias, hallucinations, transparency, and privacy cannot be ignored.
The future of AI in healthcare depends on striking the right balance—leveraging its strengths while mitigating its limitations through rigorous training, regulation, and ethical safeguards. As we embrace this powerful technology, it is essential to proceed with caution, ensuring that AI serves as a tool to augment human expertise rather than replace it.