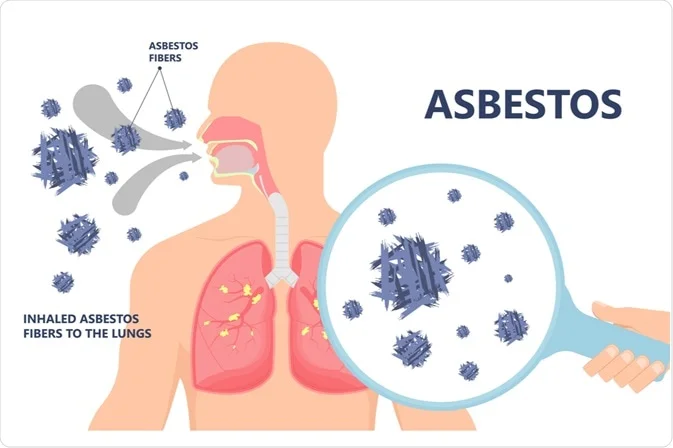
How Genomics and Big Data Can Beat Mesothelioma
November 28, 2023I. Introduction
Mesothelioma, a rare and aggressive cancer, has witnessed a rising incidence following asbestos exposure. The intricate interplay between environmental exposure and genetic susceptibility underscores the need for a comprehensive understanding of the disease. This introduction sets the stage for exploring mesothelioma at the molecular level through the lens of multi-omics, where genomic, transcriptomic, proteomic, and other omics data converge to unravel the complexities of mesothelioma’s biology.
1. Rising Incidence Post Asbestos Exposure:
- Asbestos-Related Origins: Mesothelioma’s emergence is intricately linked to asbestos exposure, with individuals exposed to this mineral exhibiting an increased risk of developing the disease.
- Latent Period Realities: The extended latent period between exposure and diagnosis emphasizes the challenges in early detection and prevention, contributing to the rising incidence.
2. Leveraging Multi-Omics to Understand Mesothelioma:
- Genomic Insights:
- Identification of Driver Mutations: Genomic analyses unveil key driver mutations in mesothelioma, shedding light on the molecular underpinnings that fuel tumor development.
- Tumor Heterogeneity: Multi-omics approaches capture the heterogeneity within mesothelioma, revealing diverse genomic profiles that influence disease progression.
- Transcriptomic Exploration:
- Gene Expression Patterns: Transcriptomics dissects gene expression patterns, offering insights into the dysregulation of cellular processes and the identification of subtype-specific signatures.
- Temporal Dynamics: Longitudinal transcriptomic studies unravel the temporal dynamics of mesothelioma, tracing how gene expression evolves over the course of the disease.
- Proteomic Unraveling:
- Mass Spectrometry Profiling: Proteomics, through techniques like mass spectrometry, identifies protein drivers and post-translational modifications, providing functional insights into mesothelioma.
- Proteogenomic Integration: Global proteogenomic initiatives integrate proteomic data with genomics, correlating protein expression with genomic alterations for a comprehensive understanding.
- Beyond Omics Data:
- Multi-Omics Integration: The integration of diverse omics data types — genomics, transcriptomics, proteomics, and more — paints a holistic picture of mesothelioma’s molecular landscape.
- Clinical Correlation: Linking multi-omics data to clinical outcomes enhances our ability to translate molecular insights into meaningful advancements in patient care.
In the face of the rising incidence of mesothelioma post asbestos exposure, the integration of multi-omics approaches emerges as a beacon of hope. By unraveling the disease’s molecular intricacies, from genomic mutations to proteomic drivers, researchers aim to pave the way for early detection, targeted therapies, and improved patient outcomes. This journey into the molecular depths of mesothelioma reflects a commitment to addressing the challenges posed by this formidable cancer through a comprehensive and integrated scientific approach.
II. Genomic Mutations and Biomarkers Implicated
Exploring the genomic landscape of mesothelioma reveals key mutations, with BAP1, NF2, and CDKN2A emerging as pivotal players. These genomic alterations not only contribute to the understanding of mesothelioma’s molecular underpinnings but also serve as diagnostic and prognostic biomarkers, shaping the landscape of personalized medicine for this challenging cancer.
1. BAP1 Mutations:
- Tumor Suppressor Role: BAP1, a tumor suppressor gene, plays a critical role in regulating cell growth and preventing the development of cancer.
- Mutational Landscape: Genomic analyses frequently identify mutations in the BAP1 gene in mesothelioma, leading to loss of its tumor-suppressive functions.
- Clinical Implications: BAP1 mutations serve as diagnostic markers, indicating a higher likelihood of mesothelioma, and may have prognostic value, influencing disease aggressiveness and treatment response.
2. NF2 Mutations:
- Tumor Suppression Disruption: NF2, another tumor suppressor gene, is involved in controlling cell division and preventing the formation of tumors.
- Genomic Alterations: Mesothelioma often exhibits mutations in the NF2 gene, contributing to the dysregulation of cellular processes.
- Diagnostic Utility: NF2 mutations contribute to the diagnostic landscape of mesothelioma, aiding in the differentiation of this cancer from other conditions.
3. CDKN2A Mutations:
- Cell Cycle Regulation: CDKN2A is a key regulator of the cell cycle, preventing uncontrolled cell division and tumor formation.
- Frequent Alterations: Genomic studies frequently detect mutations in the CDKN2A gene in mesothelioma, disrupting normal cell cycle regulation.
- Prognostic Significance: CDKN2A mutations may hold prognostic value, influencing the course of the disease and informing treatment decisions.
4. Diagnostic and Prognostic Biomarkers:
- Diagnostic Markers:
- BAP1 Loss: Loss of BAP1 function, often due to mutations, serves as a diagnostic marker, suggesting the presence of mesothelioma.
- NF2 and CDKN2A Alterations: Genomic alterations in NF2 and CDKN2A contribute to the diagnostic arsenal, aiding in the identification of mesothelioma in clinical settings.
- Prognostic Biomarkers:
- BAP1 Inactivation: Inactivation of BAP1, whether through mutations or other mechanisms, may have prognostic implications, influencing disease aggressiveness and patient outcomes.
- Genomic Subtyping: Beyond individual mutations, genomic subtyping based on the collective genomic alterations can provide prognostic insights, guiding personalized treatment approaches.
Conclusion:
The genomic mutations in BAP1, NF2, and CDKN2A stand as sentinel markers in the molecular landscape of mesothelioma. Beyond their diagnostic utility, these genomic alterations offer glimpses into the intricate biology of the disease and hold potential as prognostic indicators. As genomic insights continue to deepen, the identification and understanding of these biomarkers pave the way for tailored therapeutic strategies, underscoring the transformative potential of personalized medicine in the realm of mesothelioma.
III. Omics Approaches to Early Detection
Early detection of mesothelioma is a critical challenge, and leveraging omics approaches provides a promising avenue for identifying molecular signatures indicative of the disease at its incipient stages. This section explores the application of transcriptomics for uncovering gene expression signatures and the analysis of microRNA profiles in peripheral blood as potential omics-based strategies for early detection.
1. Transcriptomics for Gene Expression Signatures:
- Unraveling Gene Expression Patterns:
- High-Throughput RNA Sequencing: Transcriptomics, facilitated by high-throughput RNA sequencing technologies, allows for the comprehensive analysis of gene expression patterns in mesothelioma.
- Tumor vs. Normal Comparison: Differential gene expression analysis compares mesothelioma tissue with normal tissue, unveiling unique signatures associated with the disease.
- Identification of Diagnostic Markers:
- Disease-Specific Signatures: Transcriptomic analyses aim to identify disease-specific gene expression signatures that can serve as diagnostic markers for early detection.
- Subtype Discrimination: Subtyping based on transcriptomic data enhances the precision of early detection, discerning between different mesothelioma subtypes.
- Longitudinal Studies:
- Temporal Dynamics: Longitudinal transcriptomic studies track gene expression changes over time, offering insights into the temporal dynamics of molecular alterations during mesothelioma development.
- Early Warning Signs: Early changes in gene expression patterns may serve as early warning signs, enabling intervention at a stage where therapeutic outcomes are more favorable.
2. MicroRNA Profiles in Peripheral Blood:
- Circulating Biomarkers:
- MicroRNA Stability: MicroRNAs (miRNAs), stable in peripheral blood, present a promising source of circulating biomarkers for early detection.
- Non-Invasive Sampling: Analysis of miRNA profiles in peripheral blood offers a non-invasive approach, overcoming some of the challenges associated with tissue-based sampling.
- Diagnostic Potential:
- Dysregulated Expression: Dysregulation of specific miRNAs in peripheral blood may signify the presence of mesothelioma even at early stages.
- Diagnostic Panels: Combinations of miRNAs may be used to construct diagnostic panels, enhancing the sensitivity and specificity of early detection strategies.
- Monitoring High-Risk Populations:
- Asbestos-Exposed Individuals: Monitoring miRNA profiles in peripheral blood may be particularly relevant for individuals with a history of asbestos exposure, identifying those at higher risk for developing mesothelioma.
Conclusion:
Omics approaches, particularly transcriptomics and microRNA profiling, offer promising avenues for the early detection of mesothelioma. Unraveling gene expression signatures through transcriptomics and analyzing specific miRNA profiles in peripheral blood provide valuable insights into the molecular landscape of the disease. These omics-based strategies not only contribute to the development of diagnostic markers but also hold the potential to revolutionize the early intervention and management of mesothelioma, ultimately improving patient outcomes.
IV. Bioinformatics Analysis on Standard of Care
Bioinformatics plays a crucial role in analyzing the standard of care for mesothelioma, particularly in understanding pharmacogenomics and the variable chemotherapy response observed among patients. This section explores how bioinformatics contributes to unraveling the intricacies of treatment outcomes and sheds light on factors influencing why some patients respond better to standard care interventions.
1. Pharmacogenomics and Chemotherapy Response:
- Individual Genomic Variability:
- Patient-Specific Genomic Makeup: Bioinformatics assesses individual genomic variability, seeking to understand how genetic differences influence the response to chemotherapy.
- Identification of Drug Metabolizing Genes: Pharmacogenomics studies identify genes involved in drug metabolism, offering insights into variations in drug response.
- Drug Sensitivity Prediction:
- In Silico Modeling: Bioinformatics employs in silico modeling to predict drug sensitivity based on the genomic profile of a patient’s tumor.
- Personalized Treatment Strategies: Pharmacogenomic analyses guide the selection of chemotherapy drugs tailored to the specific genetic characteristics of each patient.
2. Why Some Patients Respond Better:
- Genomic Biomarkers:
- Identification of Predictive Biomarkers: Bioinformatics identifies genomic biomarkers that may predict a patient’s likelihood to respond favorably to standard chemotherapy.
- Influence of Tumor Heterogeneity: Understanding the heterogeneity within mesothelioma tumors at the genomic level helps explain varied responses to standard care.
- Immune System Interactions:
- Immunogenomic Correlations: Bioinformatics explores correlations between the genomic landscape of mesothelioma and the host immune response, elucidating factors that influence treatment efficacy.
- Immunotherapy Response Predictors: Insights gained through bioinformatics may uncover genomic features that predict how a patient’s immune system will respond to immunotherapeutic interventions.
- Evolution of Genomic Alterations:
- Temporal Analysis: Longitudinal bioinformatics studies track the evolution of genomic alterations during treatment, providing a dynamic understanding of how the genomic landscape influences response over time.
- Clonal Selection: The clonal selection of resistant subpopulations can be studied through bioinformatics, explaining why some patients develop resistance to standard treatments.
Conclusion:
Bioinformatics analysis on the standard of care for mesothelioma, particularly in the context of pharmacogenomics and chemotherapy response, unveils the complexity of individual treatment outcomes. The ability to predict drug sensitivity, identify genomic biomarkers, and understand the interplay between tumor heterogeneity and immune responses contributes to the development of more personalized and effective treatment strategies. As bioinformatics tools advance, they continue to be instrumental in deciphering the genomic intricacies of mesothelioma and, in turn, improving the precision and efficacy of standard care interventions.
V. Clinical Trials Guiding Precision Medicine
Clinical trials represent a dynamic frontier in mesothelioma research, guiding precision medicine through genomic screening for targetable mutations and exploring the potential of novel immunotherapy agents and combination therapies. This section delves into how these clinical trials, driven by bioinformatics and genomics, contribute to advancing the field and shaping personalized treatment approaches for mesothelioma patients.
1. Genomic Screening for Targetable Mutations:
- Identification of Therapeutically Relevant Mutations:
- Comprehensive Genomic Profiling: Clinical trials leverage comprehensive genomic profiling, enabled by bioinformatics tools, to identify specific mutations within mesothelioma tumors.
- Targetable Alterations: Genomic screening aims to pinpoint targetable alterations, such as mutations in specific genes, paving the way for precision medicine interventions.
- Patient Stratification:
- Biomarker-Driven Trials: Bioinformatics facilitates patient stratification based on genomic biomarkers, ensuring that individuals with specific mutations are enrolled in trials testing corresponding targeted therapies.
- Adaptive Trial Designs: Trials adapt based on emerging genomic data, optimizing patient selection and treatment arms as new insights unfold.
2. Novel Immunotherapy Agents and Combination Therapies:
- Immunogenomic Strategies:
- Immunogenomic Profiling: Bioinformatics tools analyze the immunogenomic landscape of mesothelioma, identifying potential immunotherapy targets and predicting patient responses.
- Patient Selection for Immunotherapy: Biomarker-driven approaches assist in selecting patients likely to benefit from immunotherapeutic interventions.
- Combination Therapies:
- Synergistic Approaches: Clinical trials explore the synergy between immunotherapy agents and traditional treatments, aiming for enhanced efficacy through combination therapies.
- Genomic Biomarkers for Combination Selection: Bioinformatics guides the identification of genomic biomarkers that predict which patients will derive the most benefit from specific combination regimens.
- Adaptive Immunotherapy Trials:
- Dynamic Biomarker Monitoring: Bioinformatics supports the dynamic monitoring of biomarkers during immunotherapy trials, enabling adaptive adjustments to treatment strategies based on evolving genomic landscapes.
- Real-Time Data Integration: Adaptive trials integrate real-time genomic and clinical data, ensuring that treatment decisions align with the latest insights into mesothelioma biology.
Conclusion:
Clinical trials, fueled by bioinformatics and genomics, are at the forefront of advancing precision medicine for mesothelioma. Genomic screening identifies targetable mutations, allowing for tailored treatments, while trials exploring novel immunotherapy agents and combination therapies usher in a new era of therapeutic possibilities. The integration of bioinformatics ensures that these trials are adaptive, responsive to emerging genomic data, and aligned with the goal of providing personalized and effective care for individuals affected by mesothelioma. As these trials progress, the synergy between bioinformatics and clinical research continues to shape the future of mesothelioma treatment.
VI. Sequencing Initiatives Informing Treatment
Sequencing initiatives play a pivotal role in informing treatment decisions for mesothelioma patients. This section explores how molecular tumor boards and omics tumor analysis contribute to personalized care, and how initiatives like the International Cancer Genome Consortium (ICGC) facilitate data sharing to match clinical trials with targeted therapies.
1. Molecular Tumor Boards and Omics Tumor Analysis:
- Multidisciplinary Decision-Making:
- Integration of Genomic Data: Molecular tumor boards bring together experts from various disciplines to analyze genomic data, facilitating a multidisciplinary approach to treatment decision-making.
- Clinical Interpretation: Bioinformatics tools aid in the interpretation of omics data, providing insights into the molecular characteristics of mesothelioma tumors.
- Individualized Treatment Plans:
- Tailoring Therapies to Genomic Profiles: Molecular tumor boards use omics tumor analysis to tailor treatment plans based on the specific genomic alterations present in each patient’s tumor.
- Optimizing Treatment Regimens: Bioinformatics-driven analyses assist in optimizing treatment regimens, considering the unique genomic landscape of each individual case.
- Real-Time Data Integration:
- Dynamic Patient Monitoring: Omics tumor analysis allows for real-time monitoring of genomic changes, enabling adjustments to treatment strategies as the tumor evolves.
- Treatment Adaptations: Bioinformatics tools support the dynamic adaptation of treatment plans, ensuring that they align with the evolving molecular profile of the mesothelioma tumor.
2. ICGC and Data Sharing for Trial Matching:
- International Cancer Genome Consortium (ICGC):
- Global Genomic Collaboration: ICGC serves as a global collaborative effort to characterize the genomic alterations in various cancer types, including mesothelioma.
- Datasets for Research and Clinical Use: The data generated by ICGC provides valuable insights for both research purposes and clinical applications, contributing to the understanding of mesothelioma at the molecular level.
- Data Sharing to Match Trials and Therapies:
- Facilitating Trial Matching: Bioinformatics facilitates the sharing of genomic data, enabling researchers and clinicians to match patients with mesothelioma to relevant clinical trials based on their molecular profiles.
- Optimizing Therapeutic Selection: Data sharing initiatives allow for the optimization of therapeutic selection, ensuring that patients are enrolled in trials most likely to benefit them based on their genomic characteristics.
- Cross-Institutional Collaborations:
- Pooling Resources and Expertise: Data sharing initiatives encourage cross-institutional collaborations, pooling resources and expertise to accelerate the discovery of effective treatments for mesothelioma.
- Breaking Down Silos: Bioinformatics plays a key role in breaking down silos and enabling seamless data sharing, fostering a collaborative ecosystem for mesothelioma research.
Conclusion:
Sequencing initiatives, facilitated by molecular tumor boards and data sharing platforms like ICGC, are pivotal in informing treatment decisions for mesothelioma patients. The integration of bioinformatics into these initiatives enhances the analysis and interpretation of omics data, enabling personalized and dynamic treatment approaches. As global collaborations and data-sharing efforts continue to evolve, the synergy between bioinformatics and sequencing initiatives promises to advance precision medicine for mesothelioma, ultimately improving patient outcomes and expanding our understanding of this complex disease.
VII. Bioinformatics Infrastructure Needs
Meeting the bioinformatics infrastructure needs is paramount for advancing mesothelioma research. This section explores the importance of adhering to FAIR data principles for integrative investigation and emphasizes the necessity of training interdisciplinary teams on multi-omics approaches to harness the full potential of bioinformatics in unraveling the complexities of mesothelioma.
1. FAIR Data Principles for Integrative Investigation:
- FAIR Data Principles:
- Findable, Accessible, Interoperable, Reusable (FAIR): Adhering to FAIR data principles ensures that data generated in mesothelioma research is findable, accessible, interoperable, and reusable.
- Enhancing Data Quality: Bioinformatics infrastructure must prioritize data quality and adherence to FAIR principles, facilitating robust and reproducible analyses.
- Integrative Investigation:
- Data Integration Across Omics: Bioinformatics infrastructure should support the seamless integration of diverse omics data types, fostering a comprehensive understanding of mesothelioma.
- Cross-Disciplinary Collaboration: Integrative investigation requires collaboration across disciplines, emphasizing the need for bioinformatics tools that support data harmonization and integration.
- Platform Agnostic Approach:
- Platform Neutrality: Bioinformatics tools should adopt a platform-agnostic approach, ensuring compatibility with various sequencing platforms and analytical techniques.
- Interoperability: Interoperability is crucial for integrating data generated from different platforms, allowing for a more holistic analysis of mesothelioma genomes.
2. Training Interdisciplinary Teams on Multi-Omics:
- Multi-Omics Proficiency:
- Cross-Training: Interdisciplinary teams need cross-training initiatives to build proficiency in multi-omics approaches, fostering a collaborative and synergistic research environment.
- Understanding Data Integration: Training programs should emphasize the nuances of integrating genomics, transcriptomics, proteomics, and other omics data for a comprehensive analysis.
- Statistical and Computational Skills:
- Enhanced Statistical Literacy: Bioinformatics training should enhance the statistical literacy of researchers, enabling them to critically analyze and interpret complex multi-omics data.
- Computational Proficiency: Proficiency in computational tools and programming languages is essential for handling large-scale omics datasets efficiently.
- Ethical Considerations:
- Responsible Data Use: Training programs should instill ethical considerations regarding the responsible use of genomic and omics data, emphasizing data privacy, informed consent, and responsible sharing practices.
- Ethics in Multi-Omics Research: The interdisciplinary training should include discussions on ethical challenges specific to multi-omics research, such as data integration and privacy concerns.
Conclusion:
Addressing the bioinformatics infrastructure needs for mesothelioma research involves a dual focus on FAIR data principles and training interdisciplinary teams on multi-omics approaches. Adherence to FAIR principles ensures the quality and accessibility of data, while cross-disciplinary training empowers researchers to leverage the full potential of bioinformatics for integrative investigations. As the field advances, robust infrastructure and skilled interdisciplinary teams are essential pillars for unlocking the intricate molecular landscape of mesothelioma and translating these insights into meaningful advancements in diagnosis and treatment.
VIII. The Future of Omics Informing Mesothelioma Care
The future of mesothelioma care lies in the convergence of multi-omics data and advanced machine learning techniques. This section explores the potential of integrating diverse omics datasets through machine learning and leveraging artificial intelligence (AI) to accelerate biomarker discovery, ushering in a new era of precision medicine for mesothelioma patients.
1. Multi-Omics Integration with Machine Learning:
- Holistic Data Synthesis:
- Comprehensive Insight Generation: Integrating genomics, transcriptomics, proteomics, and other omics data types provides a holistic view of the molecular landscape of mesothelioma.
- Machine Learning Algorithms: Advanced machine learning algorithms analyze multi-omics data, identifying intricate patterns and relationships that may not be apparent through individual omics analyses.
- Predictive Modeling:
- Disease Progression Prediction: Machine learning models, trained on integrated omics data, can predict the progression of mesothelioma, offering valuable insights for clinical decision-making.
- Treatment Response Prediction: Predictive modeling helps anticipate individual responses to specific treatments, guiding the selection of personalized therapeutic strategies.
- Identification of Novel Biomarkers:
- Uncovering Hidden Relationships: Machine learning identifies complex relationships within the multi-omics data, unveiling novel biomarkers that may serve as diagnostic or prognostic indicators.
- Precision in Biomarker Selection: By considering the interplay between different molecular layers, machine learning enhances the precision of biomarker selection for mesothelioma.
2. Accelerating Biomarker Discovery with AI:
- AI-Driven Data Analysis:
- High-Throughput Analysis: AI algorithms enable high-throughput analysis of vast omics datasets, accelerating the identification of potential biomarkers associated with mesothelioma.
- Automated Pattern Recognition: AI automates the process of pattern recognition in complex biological data, streamlining the discovery of relevant biomolecular signatures.
- Data Mining and Integration:
- Mining Biomedical Literature: AI tools mine vast biomedical literature to uncover existing knowledge relevant to mesothelioma, facilitating the integration of historical insights with current research.
- Knowledge Graph Construction: AI contributes to the construction of knowledge graphs that map relationships between genes, proteins, and pathways implicated in mesothelioma.
- Real-Time Learning:
- Adaptive Algorithms: AI algorithms continuously learn from new data, adapting to evolving patterns in mesothelioma molecular profiles.
- Real-Time Updates: The ability of AI to provide real-time updates ensures that biomarker discovery remains at the cutting edge of scientific understanding.
Conclusion:
The future of omics informing mesothelioma care is characterized by the synergistic integration of multi-omics data and advanced machine learning. Machine learning algorithms, powered by AI, not only provide a comprehensive understanding of the disease but also expedite the discovery of biomarkers crucial for personalized diagnosis and treatment. As these technologies continue to evolve, they hold the promise of transforming mesothelioma care by unraveling its molecular intricacies and paving the way for more targeted and effective interventions. The intersection of multi-omics and AI represents a powerful paradigm shift towards precision medicine in the management of mesothelioma.